This research was based on assessing the use of e-governance by local authorities to improve service delivery: A case of Chitungwiza Municipality. The research objectives were to find out the benefits of e-governance system, to find out the challenges being faced by Chitungwiza Municipality in implementation and use of e-governance system and to proffer possible strategies that can be employed to ameliorate some of the challenges being faced. The research was confined to Chitungwiza respondents only. Literature review then focused on giving description of e-governance, benefits of e-governance, challenges being faced by the local authorities in using e-governance, strategies that can be adopted by local authorities to implement and use e-governance and the use of e-governance system by other local authorities in different countries. Descriptive research design was used. Stratified random sampling technique was used to select the respondents. The sample for the research was 50 respondents which constituted 10 employees, 10 top management and 30 residents. Questionnaires and personal interviews were used as the research instruments. An overall response rate of 84% was obtained from the questionnaires and interviews. Data presentation and analysis was done. Findings obtained from the research study were presented in tables, graphs and pie-charts followed by the analysis. Major findings of the research were that, Chitungwiza Municipality is faced with financial shortages, lack of adequate machinery and minimum support from the government so as to fully implement and use e-governance system to improve service delivery. The council does not have its website where people can access information. Some of the council departments do not have adequate computers and some no longer function well. People have limited knowledge on e-governance and they do not have adequate knowledge on the benefits of using e-governance system. Strategies that can be adopted by Chitungwiza Municipality to implement and use e-governance include engaging public private partnerships, training of personnel, increasing awareness to the public on e-governance, and city twinning. Lastly recommendations were made which include, efforts should be made to ensure that e-governance system is taken aboard by the Municipality in years to come in order to improve service delivery to the public through advanced use of internet and council website, the council need to plan and have a council website so as to make services accessible to the citizens ,training of staff should be done so that they can gain deep understanding on the benefits of e-governance and the council should make use of public private partnerships and city twinning so as to acquire financial and other resources in order to implement and use e-governance system to improve service delivery.
INTRODUCTION |
From an instructional design perspective, the presence of
support devices, also known as tools in computer-based learning environments (CBLEs) aims to enhance learning
outcomes/performance [1]. Nevertheless, independent of the
intentions behind the design of the tools, tools are value-neutral
until learners use them [2]. How (in-) adequately learners use
the tools affects the tools functionality and hence influences
the performance [3]. However, using tools often seems an
unappealing activity for learners and hence they often avoid to
use the tools [4]. |
The problem raised by tool use indicates that there is a
complex, interactive and non-linear tool-learner relationship
[5]. Unfortunately, this relationship of tools and learners as
intellectual partners and their partnering processes is still
limited in research and needs to be unraveled. On the one
hand, there is the tool with different characteristics. These
characteristics are linked to the intention of the instructional
designer [6]. On the other hand, there is the learner with inherent,
intrinsic characteristics. These characteristics are cognitive,
metacognitive as well as motivational. How exactly tool and
learner characteristics interact and affect tool use; and how tool
use impacts the tools’ functionality and therefore influences
performance are questions that were examined in the course of
four experiments (Table 1). |
The four studies were built upon Winne’s cognitive conditions,
Perkin’s framework and Iiyoshi and Hannafin’s tool use
strategies [5,7,8]. Optimal tool use –which would lead to
positive learning outcomes-was conceptualized as a thoughtful
process in which: |
1. The tool is present and functional. The impact of tool use on
performance should reveal the tools functionality. Additionally,
if the tools are present, further characteristics associated to
the tools may come into play. These are the type of tools (eg.
cognitive tools), the tools delivery mode (embedded vs. nonembedded
tools) and tool advice (explanation vs. no explanation
of tool functionality). 2. Learners recognize the tool [5], choose the tool that may be
better for their learning [7], and use the tool(s) skillfully [8]. If
learners are capable of recognizing, choosing and using tools
skillfully, they possess metacognitive characteristics such as
perceptions and self-regulation skills that help them in the tool
use process. |
3. Learners are motivated to use the tools skillfully. Learners’
motivation determines the effort learners will invest in using
the tools and is therefore linked to the optimization of tool use.
Self-efficacy and goal-orientation are two characteristics with a
motivational nature that have been explored in tool use research
[5,7-9]. |
In addition to the aforementioned tool and learner characteristics,
these conditions also refer to a learner characteristic with
a cognitive nature that should not be taken for granted when
investigating tool use. This characteristic, namely domainspecific
prior knowledge is inherent to the learner. Prior
knowledge is known for its power to predict performance
[9,10] and is considered as an important characteristic either
enabling or impeding learning with the use of tools [8,11,12].
Prior knowledge also seems to impact tool use and interact with
metacognitive and motivational learner characteristics [11-13]. |
THE STUDIES AND SUMMARY OF FINDINGS |
The initial study was exploratory and was essential to set the
baseline of the following studies. In order to do so, it was
important to first investigate whether the complexity of tool
use only stood by CBLEs or not. Therefore, we endeavored to
analyze the use of tools in an environment extrinsic to CBLEs.
The use of tools was examined in a learning environment
using a psychomotor task [14]. Learners had to build a LEGO
figure with the help of two tools: a guideline and/or a video.
There were four conditions, with guideline, with video with
guideline and video and with no tools. The results revealed
that the tools were functional: Significant positive effects on
performance were observed. This means that the groups using
tools performed better than those without them. However, when
confronted with multiple tools, learners had trouble identifying
the most functional tool. It seems that the different types of tools influenced the way learners perceived the tool functionality. As
a consequence, learners used the “least functional tool” instead.
Effects of learner characteristics and further tool characteristics
could not be retrieved. |
In the subsequent studies (1, 2 and 3) more extended and indepth
investigations were performed. The experimental studies
were carried out in a CBLE context. The experiments only
differed with respect to the tools used: Adjunct questions (Study
1), semi-structured concept maps (Study 2) and multiple tools:
adjunct questions and semi-structured concept maps (Study 3)
[15,16]. Each of the studies had two embedded conditions with
explanation of the tool functionality and two non-embedded
conditions without explanation of the tool functionality.
Studies 2 and 3 included a control condition (no tools, no
explanations of the tool functionality). In general the results
revealed that domain prior knowledge, embedded tools and
adjunct questions influenced quantity of tool use positively. In
contrast, goal orientation, self-efficacy and the explanation of
the tool functionality showed a negative effect on quantity of
tool use while perceptions and self-regulation showed mixed
effects. Regarding quality of tool use, goal orientation and
non-embedded tools showed a positive relationship with it.
Lastly, significant correlations among quantity and quality of
tool use were observed and only quantity of tool use impacted
performance. |
The following section discusses the results in more detail. The
findings that are related to the tool functionality and hence the
effects of tool use on performance are firstly addressed. Next,
the results based on the effects of tool and learner characteristics
on tool use are outlined. |
MAIN FINDINGS |
Tool Use and Performance |
In order to fully address the functionality of the tools, it was
expected that tool use would impact performance positively [4].
In all the studies, the use of the tools had a positive effect on the
performance. In the Exploratory Study, the conditions with tools
outperformed the control condition. However, learners using
the tool that was considered the ‘least’ functional showed better
performance. Moreover, learners with multiple tools could not
see which tool was the most functional one. |
In the following studies (Studies 1, 2 & 3) tool use was
investigated from a quantitative (time and frequency) and
qualitative perspective. Studies 1 and 2 used single tools.
Study 3 used multiple tools. In Study 1 (with adjunct questions
as tools) the results showed that only time spent on the tools
impacted performance in a positive way. The other aspects of
tool use (frequency and quality) showed no significant effects
on performance. In Study 2 (with semi-structured concept
maps as tools), both aspects of quantity of tool use (time and
frequency) influenced performance. The results were mixed.
While the time spent on tools impacted performance positively,
frequency of tool use had a negative impact on performance.
Quality of tool use did not impact performance significantly.
To allow further comparisons and possibly analyze the tools
functionality more deeply, Study 2 included a control condition
without tools. The addition of a control condition revealed that the differences among conditions in relation to performance
were minimal and no significant differences could be retrieved.
This means that the learners in the conditions with tools did not
perform any better than the ones with no tools, but they did not
perform worse either. |
In the last study (Study 3: with adjunct questions and semistructured
concept maps as tools), positive effects of time spent
on the tool on performance were observed. However, these effects
were only observed when using the adjunct questions. Time
spent on the semi-structured concept maps did not significantly
impact performance. Furthermore, neither frequency nor
quality of tool use of either of the tools impacted performance
in a significant way. However, a significant difference regarding
performance was retrieved between learners using tools and
those with no tools (experimental vs. control condition). |
Interrelationships among time, frequency and quality were
also investigated in Studies 1, 2 and 3. The results, first,
indicated that there seemed to be significant correlations
between frequency and quality of tool use. The direction of this
relationship, however, is mixed. Study 1 suggested a negative
correlation between quality and frequency of tool use while
Study 2 indicated a positive relationship among these two tool
use measurements. Study 1 used adjunct questions and Study
2 employed semi-structured concept maps. In addition, in
Study 3 a negative correlation between quality of tool use and
time spent on tools (in adjunct questions) was reported; also a
positive relationship between time spent on adjunct questions
and time spent on concept maps was found. Figure 1 represents
the aforementioned findings. |
Learner and Tool Characteristics Effects on Tool Use |
The findings showing the effects of the different learner and
tool characteristics will be described in terms of tool use.
This means that the different learner and tool characteristics
influencing the quantity of tool use (time and frequency) will be
firstly explained. The different learner and tool characteristics
influencing the quality of tool use will be described afterwards. |
Quantity of tool use: Time spent on tools |
Self-regulation, perceptions and goal orientation influenced the
time spent on the tools. With respect to the tool characteristics, tool type, tool advice and tool delivery mode influenced the
time spent on the tools. How exactly they affected tool use
reveals a complex interplay among the different learner and tool
characteristics and is further explained. |
Three self-regulation skills influenced the time spent on the
tools. Two out of these three self-regulations skills, namely
‘elaboration’ (Study 2) and ‘critical thinking’ (Study 3) had a
positive impact on the time spent on the tools. In contrast, the
self-regulation skill of ‘organization’ (Study 2) impacted time
spent on the tools negatively. Considering the definitions of
‘elaboration’ and ‘critical thinking’, these results suggest that
the more learners were oriented towards a deeper understanding
by means of study activities, in this case the use of tools
(elaboration), and the deeper the understanding learners had
about the tools the more time they decided to spend on the use
of the tools [17]. The difference was that high elaborators spent
more time on semi-structured concept maps whereas high critical
thinkers spent more time on the adjunct questions. Moreover,
learners with high organization skills, that is, learners who are
able to reorganize learning material to perform optimally were
inclined to spend significantly less time on the tools, specifically
semi-structured concept maps [17]. |
The role of perceptions seemed mixed. ‘Perceived functionality’
showed a negative influence on the time spent on tools. This
means that the degree to which learners believed that using a
certain tool would enhance his/her performance in order to reach
a goal contributed to spending less time on the tools, specifically
semi-structured concept maps (Study 2) [18,19]. The effect of
perceived functionality, however, was not consistent. This effect
disappeared when it was controlled for different conditions
(Study 2) and when more learner characteristics were included
in the analysis (Study 2). The other perception of tool use,
namely ‘perceived usability’ showed positive as well as negative
effects on the time spent on tools. Considering that perceived
usability is the degree to which a learner believes that a certain
tool would be usable and easy to use, then believing that the
semi-structured concept maps would be usable and easy to use
led to more time spent on the tools (Studies 2), but believing
the same about adjunct questions led to less time spent on tools
(Studies 3) [18,19]. |
High performance avoidance goal orientation had a negative
impact on the time spent on tools. Performance avoidance
focuses on avoiding normative competence, refers to low
competence expectancies, fear of failure and avoidance of
failure, which means that learners avoided and feared failure
decreased the time they spent on the semi-structured concept
maps (Study 3). Figure 2 summarizes the findings on the learner
characteristics influencing the time spent on tools [20]. |
With respect to tool characteristics and their influence on
time spent on tools, results were as follows. In general the
type of tool influenced the time spent on the tools in Study 1
(adjunct questions) and Study 2 (semi-structured concept map).
When multiple tools were used, however, (Study 3: adjunct
questions and semi-structured concept maps), spending more
time on adjunct questions contributed to better performance
than spending time on concept maps. The tool delivery mode
(embedded or non-embedded tools) and the tool advice (the explanation of the tool functionality or no explanation of tool
functionality) were characteristics dichotomous in nature in
Studies 1, 2 and 3. The results pointed out that learners spent
more time on embedded tools (Study 1 and 3) than on nonembedded
tools; moreover, it also seemed that learners without
the explanation of the tool functionality invested more time on
the tools (Study 2) than those with the explanation of the tool
functionality. Figure 3 represents these results. |
Quantity of tool use: Frequency of tool access: |
The frequency of tool use was only examined in non-embedded
conditions. Along the studies in this dissertation, no direct
effects of tool characteristics on frequency of tool use were
observed. Regarding learner characteristics domain prior
knowledge (Study 2) and self-efficacy (Study 1) seemed to
influence significantly the frequency of tool use. Domain prior
knowledge refers to the knowledge learners possess about the
topic presented in the instructional text from the CBLE task.
As previously addressed, domain-related prior knowledge is
an important cognitive learner characteristic to predict learning
[9,10] and either enables or impedes learning with the use of
tools [8,10,12]. Self-efficacy refers to the personal beliefs about
having the means to organize or execute the courses of action
to perform effectively (Bandura, 1997), which implies that selfefficacy
influences how learners approach tools. The impact of each of these characteristics is remarkable. Learners with high
domain prior knowledge showed more frequency of tool use
than with low prior knowledge; whereas learners with high selfefficacy
showed less frequency of tool use than learners with
low self-efficacy. In other words, learners who knew more about
the topic clicked more often on the button that would access the
tool, in this case, semi-structured concept maps. On the other
hand, the more learners believed in their own ability to complete
the CBLE task, the fewer times they attempted to click on the
button to access the tool, in this case adjunct questions. Figure 4
summarizes these findings. |
Quality of tool use: |
Goal orientation and tool delivery mode appeared to have an
impact on quality of tool use. The specific goal orientation that
influenced quality of tool use was performance approach (Study
1). Performance approach is related to learners’ concerns about
how well they perform and how others perceive their behavior
[20]. This means that learners who were more concerned about
their performance and how others perceived it had more thorough
answers in the adjunct questions than learners who were less
concerned about public recognition. Additionally, low and high
mastery avoidance also impacted quality of tool use positively
but only in interaction with the non-embedded conditions.
This suggests that learners striving to avoid misunderstanding,
failing, making mistakes and/or doing anything wrong along
with the freedom to access the tools affected the way learners
answered the adjunct questions [20]. Finally, the non-embedded
conditions also impacted quality of tool use directly (Study
1). Learners with the freedom to access the tools showed
better responses in the adjunct questions. These findings are
summarized in Figure 5. |
All in all, these results allowed sketching the complexity of
tool use not only in a CBLE but also in a psychomotor context
(Exploratory Study). The findings also make several noteworthy
contributions to the phenomenon of tool use regarding the effects
of tool and learner characteristics on tool use, and the impact
of tool use on performance. However, the present findings do
not give a uniform answer to the tool use problem. They rather
present an intricate picture of the relationships between learner
characteristics, tool characteristics, tool use and performance and question the functionality of the tools. In addition, these
results raise further questions. These questions are comprised in
three issues regarding the impact of 1) tool use on performance
which relate to the tool functionality 2) learner characteristics
on tool use and 3) tool characteristics on tool use. Furthermore,
methodological issues are addressed. These issues constitute a
challenging research plan that is further discussed (Figure 6). |
Discussion |
Issue 1: Tool Use and Performance: |
With regard to the lack of coherence in the effects of tool use on
performance, two main research questions can be identified. A
first question deals with whether the tool measurements (time,
frequency and quality) are valid indicators of what they claim
to evaluate. For instance, given the positive correlations, it is
wondered whether frequency of tool use can be more a measure
of quality than of quantity of tool use or whether quality of tool
use can be more a measure of quantity of tool use. Literature
has already suggested that quality can be examined using
time variables [21]. It is also wondered whether frequency
and quality of tool use are indicators of the same underlying
variable. The correlation found in this dissertation suggests that
the high frequency of tool use is closely related to the quality of
tool use and together affect performance negatively. Given that frequency of tool use was only measured in the non-embedded
conditions, then it could be possible that non-embedded tools
may indirectly hamper performance, as well. This positive
correlation between frequency and quality of tool use could
only be observed when learners used semi-structured concept
maps as the tools. When learners used the adjunct questions,
frequency of tool use affected performance negatively. This
finding suggests that the type of tool may influence tool usage
behavior. Therefore the correlations among time, frequency
and quality of tool use should be further explored along with
different tool types and tool delivery mode (Figure 6). |
A second question relates to how these results challenge the
functionality of the tools by raising the question on (a) whether
the three tool use measurements (time, frequency and quality)
are necessary elements to explain tool usage, and on (b) whether
the three tool use measurements should impact performance in
order for the tool to be considered as functional. Studies on tool
use seldom explored time, frequency and quality in a single
study. For instance, Crippen and colleagues explored frequency
and retrieved a positive impact on performance [22]. Elen
and Louw saw only partial effect of frequency of tool use on
performance, while Viau and Larivée showed effects of both
time and frequency on performance [1,23]. Few studies have
simultaneously explored the time, frequency and quality of tool
use [24,25]. Clarebout and colleagues only found significant
positive effects of time and quality on tool use on performance;
Jiang and Elen had positive effects of quality of tool use on
performance but negative effects of frequency of tool use on
performance and partial effects of time on tool on performance
[24,25]. Other studies have not analyzed the effects of tool use
on performance [26,27]. This evidence may therefore indicate
that in a single study three tool use measurements may not
be necessary in research on tool use. It could be that time,
frequency and quality of tool use either compensate for each
other or because they measure essentially the same. However,
it is also wondered under what circumstances, how and what
interrelationships between tool and learner characteristics
impact tool use (time & frequency) which in turn influences
performance (Figure 6). |
Issue 2: Learner Characteristics on Tool Use: |
The second issue relates to the identification of learner
characteristics that strongly affect tool use. This dissertation
was based on the theoretical assumption that prior knowledge,
metacognitive and motivational characteristics are relevant in
CBLEs. The results of the studies indicated that indeed all these
characteristics may be important to consider regarding tool use. |
Domain prior knowledge was considered an important cognitive
variable with the power to restrain or encourage tool use [8].
Prior knowledge showed a positive effect on the frequency of
tool use (Study 2). This finding contradicts the results from
Renkl’s study (learners with high prior knowledge accessed tools
less often), and at the same time, it reveals that prior knowledge
may interact with the tool delivery mode (embedded vs. nonembedded)
[11]. Learners with high prior knowledge were more
inclined to access the tools in the non-embedded conditions than
those with low prior knowledge. However, given that frequency
of tool use impacted performance negatively (Study 2), high levels of prior knowledge may have indeed tainted tool use,
hence performance. These results are somewhat puzzling since
they bring to light that there may be an interaction between prior
knowledge and the tool delivery mode (Figure 6: Issue 2). A
further discussion is provided in issue 3. |
Metacognitive characteristics are essential for learners to
determine to what extent tools can aid their learning [4,7].
The results with respect to the impact of the metacognitive
characteristics were striking but not always consistent. For
instance, both perceptions and self-regulation skills impacted
the time spent on the tools in both a positive and a negative
way. In relation to self-regulation skills, ‘critical thinking’
encouraged more time spent on the adjunct questions (Study
3) while ‘elaboration’ encouraged more time on the semistructured
concept maps (Study 2). In contrast, ‘organization’
contributed to spending less time on the semi-structured
concept maps (Study 2). Regarding perceptions, ‘perceived
functionality’ of concept maps had a negative effect on the time
spent on the tools (Study 2). Additionally ‘perceived usability’
of concept maps showed a positive effect on the time spent on
tools (Study 2) but ‘perceived usability’ of adjunct questions
showed a negative effect on the time spent on tools (Study 3).
Moreover, given that time spent on tool impacted performance,
these results also suggest that self-regulation and perceptions
indirectly affect performance. These results strongly suggest
self-regulation skills and perceptions interacted with the tool
type. How exactly and what levels of self-regulation skills and
perceptions affect tool use and how they relate to tool type should
be further analyzed. Adding more diverse tool types into future
experiments could allow further comparisons (Figure 6: Issue 2
& 3). Moreover, although the log files provided a rich dataset, a
deeper insight is needed. Observation techniques could provide
additional data on the learners’ behavior that cannot be retrieved
through log files only. (Figure 6: Methodological issues). |
Motivational characteristics are crucial to prompt tool use [4,7].
The findings regarding the motivational characteristics show that
the effects of goal orientation and self-efficacy are not always
linear. For instance, low and high levels of mastery avoidance in
interaction with non-embedded conditions had a positive effect
on quality of tool use. In contrast, medium levels of mastery
avoidance in interaction with non-embedded conditions did
not impact tool use significantly (Study 1). The effects found
of the performance orientations indicated that ‘performance
approach’ influenced quality of tool use positively (Study 1)
and ‘performance avoidance’ influenced the time spent on the
tools negatively (Study 3). Considering that the more time
spent on the tools, the better the performance, then performance
avoidance also impacted learning outcomes in a negative
way. Furthermore, in the last study (Study 3), size effects of
mastery approach on frequency of tool use in concept maps and
mastery avoidance on quality of tool use of adjunct questions
were retrieved. The results were not significant; however, they
do provide evidence of the strength of goal orientation on tool
use and supports the claim suggesting that goal-orientation is
a promising factor in the complexity of tool use that should
be further investigated [28] (Figure 6: Issue 2). In relation to
self-efficacy, high self-efficacy levels influenced negatively the frequency of tool use. The direction of this influence, however,
remains unclear. |
The results on the motivational characteristics (self-efficacy
and goal orientation) put at stake the theoretical position
suggesting that motivation is crucial for tool use and therefore
encourage to explore tool use more deeply [4]. Based on the
present results, it is possible that learners with high self-efficacy
also had low levels of prior knowledge and learners with low
self-efficacy had high prior knowledge. The interaction among
these characteristics may eventually influence the frequency of
tool use. However, while our finding gives light to a possible
interaction, it was unfeasible to check this implication as the
results were obtained in different studies; hence, it is still a
conjecture that needs further assessment (Figure 6: Issue 2). |
An additional remark in the light of these findings is in line with
a possible interaction among learner and tool characteristics.
Certain characteristics only affected the time spent on the
tools in the presence of certain tools. The self-regulation skills
of elaboration and organization, performance avoidance goal
orientation and perceived functionality affected the time spent
on tools when using concept maps. Critical thinking affected
the time spent on tools when using adjunct questions. Perceived
usability showed a positive influence when using concept maps
but a negative one with adjunct questions. Moreover, mastery
avoidance only affected quality tool use in interaction with
the tool delivery mode specifically non-embedded conditions.
Questions are raised in identifying how exactly the type of tools
affect perceptions, and on what kind of learners need embedded
or non-embedded tools (tool delivery mode). These findings also
challenge the theoretical model in the present dissertation and
provoke a hypothesis that tool and learner characteristics may
not only interact with each other but also correlate significantly
(Figure 6: Issue 2 & 3). |
This finding suggests that perceived usability and perceived
functionality are valid measures related to tool use in CBLEs.
Therefore future studies exploring perceptions of tool use
could use the present results as a baseline (Figure 6: Issue 2).
How exactly the explanation of the tools affected perceptions
seems unclear. Additionally, investigating the relationships
between tool and learner characteristics using other research
methodology (mixed methods research) or other types of
instruments, such as interviews or think aloud protocols could
give a deeper insight into these intertwined relationships. This
will be further addressed in the methodological issues (Figure 6:
Methodological issues). |
As a last remark and to increase the complexity of the study
of tool use, there may be another reason that contributed to the
present findings. Results could be attributed to the learners’ age.
The cognitive development of learners is modified/improved
as they grew older. Consequently, tool use improves with age
[28].The older someone is the more developed their cognition
is; hence the better the performance in relationship to prior
knowledge [28]. Age can be interpreted as a variable that
integrates other factors, among those are the educational level
and self-regulation skills (Study 2). |
Issue 3: Tool Characteristics on Tool Use: |
A third issue is related to tool characteristics. This dissertation
was based on the theoretical assumption that tool type, tool
delivery mode and tool advice has an impact in CBLEs. The
results of the studies revealed very interesting findings. |
The effects of a functional and a less functional tool were
investigated in the Exploratory Study. In the following studies
(1-3), two types of cognitive tools were explored. These were a
knowledge organization tool (semi-structured concept map) and
a knowledge generation tool (adjunct question). The findings in
all studies indicate that all the tools were functional, that is, the
effects on performance seemed favorable. The positive effects
of the different tools were more evident when only one of the
two tools was present in the CBLE (Studies 1and 2). However,
when learners faced both tools –multiple tools- (Exploratory
Study and Study 3), tool use was not as optimal as expected.
That means that in the Exploratory Study, the use of the nonfunctional
tool led to better learning outcomes/performance
than by using the functional tool; and in Study 3, only the
use of adjunct questions, showed a positive relationship with
performance. |
Specifically, the result of the Exploratory Study was explained
in terms of the mirror neuron system: the non-functional tool
(video) was more functional given that dynamic visualization
may be most efficient in psychomotor tasks; in Study 3 it was
discussed that the choice of tools seems to be influenced by
how easy to use and usable learners perceive the tool (perceived
usability) [29]. This perception may or may not be positive. In
general, it could be argued that the results from the Exploratory
Study and Study 3 are in line with the task-switching paradigm
[30,31]. The task-switching paradigm involves the ability to
shift attention between cognitive tasks. This shift (in this case
the shift between one tool and the other) make learners more
likely to respond substantially slower and with a tendency to
make more errors which is addressed as a ‘switch cost’ [32]. In
the Exploratory Study and Study 3 the multiple tools possibly
had a switch cost effect in the learners. Switch costs could be
investigated in further tool use research by comparing how long
it takes for learners to use different tools, the researchers could
measure the cost in time for switching from one tool to the other.
Researchers could also assess how different aspects of the tools,
such as tool familiarity, affect any extra time cost of switching
[33] (Figure 6: Issue 3). Another approach could be through
neuroimaging experiments which involve the use of different
techniques to either directly or indirectly image the function of
the brain [32] (Figure 6: Methodological issues). |
Another reason why in Study 3 learners were more inclined
to use either one of the tools could be caused by the reading
skills that were elicited by each tool. Adjunct questions may
require more skimming skills, while the concept maps may
require more scanning skills. Broadly speaking, skimming is
fast reading to get the main idea of the text –thus answer the
adjunct question-; scanning is used to locate single fact/concepts
– that were necessary to complete the concept maps. Another
reasoning could be related to the tool familiarity (Figure 6:
Issue 3). It is possible that learners tended to use the tools they
are more familiar with (Exploratory Study and Study 3). It is a challenge in research to discover which tools may be more
functional to certain learners, for what tasks and why. Taking
qualitative research techniques, such as interviews, into account
may provide a better understanding. This is because qualitative
research aims to explore the human elements of a topic by
locating the researcher into the world and allowing him/her an
in-depth understanding on how individuals see and experience
the world [34]. In this case, qualitative research techniques may
provide a more thorough understanding on how the learners
experience the use of tools in CBLEs. |
Additionally, the types of tools explored in Studies 1-3 were
considered cognitive tools. Among cognitive tools, various
subcategories were established [8]. In this study, differences
among two different cognitive tools were encountered: a
knowledge organization tool (semi-structured concept map) and
a knowledge generation tool (adjunct question). The questions
are (a) whether a more varied types of tools, for example,
information or scaffolding tools can bring different effects on
tool use and (b) how other tools (e.g., scaffolding tools) interact
with other tool characteristics [35] (Figure 6: Issue 3). |
Tool delivery mode, that is embedded and non-embedded tools,
was investigated in the present dissertation. Learners using the
embedded tools spent more time on the tool, while learners
using the non-embedded tools, used tools more qualitatively.
More specifically, the learners using the embedded tools with
the explanation of tool functionality spent more time on the
tools, than those with embedded tools but without explanation.
Given that only the time spent on the tools had a positive effect
on performance, these results suggest that embedding tools may
be a straightforward solution for using tools and ensure positive
learning outcomes/performance and enhance tool functionality.
However, learners’ control over their learning is reduced and
this lack of control can taint the individual learning processes
[36]. Learners using the non-embedded tools, regardless of the
(no) presence of the explanation, used tools more qualitatively.
Moreover, learner characteristics of prior knowledge and selfefficacy
seem to interact with non-embedded conditions. Thus,
it is possible that the role of learner characteristics has more
incidence in the non-embedded conditions because there is
more room for their influence (Figure 6: Issue 3). In the nonembedded
conditions learners have to rely more on their own
decisions because they have more ‘freedom’ to use the tools
when they believe they need to. These results are not conclusive,
though. In Study 3, the effect of tool delivery mode seemed to
be overpowered by the explanation of the tool functionality. The
explanation of tool functionality -rather than encouraging the
learners to use the tools- discouraged them. Learners with the
explanation of tool functionality spent less time on the tools
(which eventually affected performance negatively). How and
how often the explanation of the tool functionality is added in
the CBLE seems a challenge in research on tool use. Learner
characteristics may also interact with the explanation of the tool
functionality. What and how learner characteristics affect the
explanation of tool functionality or vice versa seems a challenge
for future studies. Knowing the type of learners a priori may
help the designers of CBLEs decide when to embed tools, what
kind of tool(s) should be present (information, cognitive and/
or scaffolding) and whether or not the explanation of tool use should be present. It is therefore necessary to conduct more
investigations that can help identify the most optimal tool
characteristics for every type of learner (Figure 6: Issue 3). |
Finally, it is also questioned whether the tool characteristics
were well-developed. Regarding tool delivery mode, for
instance, in the present studies, the tools were either embedded
or non-embedded. The non-embedded tools could be at a zero
level of “embeddedness” whereas the embedded tools may
vary in their “embeddedness” level [23,37,38]. Considering
for instance that if a workout example is provided in such way
that learners have to read it then the embeddedness is level 1.
If the workout example requires students to calculate and fill in
the missed information during problem solving before proceed,
then the embeddedness is level 2. If a workout example not
only asks for the calculation but also requires students to
explain their reasoning, then the embeddedness level is even
higher. In this dissertation, it is possible that the embeddedness
of the tools varied. When learners used the adjunct questions,
they were required to give an answer and provide a reason to
it. When learners used the semi-structured concept maps they
were required to fill in the blanks with the correct concepts but
no further rationale was required. It is therefore possible that
different levels of “embeddedness” took place in the tools of
this investigation. Having different levels of “embeddedness”
for the same tool may allow a deeper insight into the tool
delivery mode and its effects on tool use (Figure 6). |
Methodological Issues Q |
The findings of the present dissertation raise methodological
issues as well. A first methodological issue pertains to the
approach of the investigation. The approach was mainly
quantitative. Adding qualitative approaches could shed light
to many questions that still are unanswered. Most importantly,
investigating the use of tools should take a step beyond by
adopting different research approaches. A mixed methods
research, which has been gaining in popularity since the 1980s,
is an approach to research that combines the collection and
analysis of quantitative and qualitative data [39]. A mixed
methods research is used to tackle a research question from
any relevant angle and possibly more than one type of research
perspective [40]. For instance, the use of log files in combination
with observations, think aloud protocols and/or self-reports
(e.g., interviews and questionnaires) could already allow the
recollection of data we may not know of; and hence contribute
to a more thorough understanding of the tool use problem. |
Another methodological issue is related to the design and data
analyses. Considering the number of variables involved in the
tool use interplay, a larger sample could allow more sophisticated
analyses such as structural equation modeling (SEM). More
sophisticated statistical analyses in conjunction with qualitative
methods can reveal important findings that may lead to the
unraveling of tool usage (Figure 6). Additionally, in all the
studies the samples comprised only students of educational
sciences. This may add limitations to the generalization of the
findings. Including more heterogeneous samples could validate
the results on the effects of the different learner and tool
characteristics of tool use of the present dissertation. |
A third methodological issue is raised in response to the research setting. The studies were run in an experimental setting. The
findings could be prone to criticism as they may not reflect
real life. It is therefore important to conduct complementary
studies in different research settings (i.e., ecological setting).
The research setting is also related to the physical, cultural and
social environments by which the research study is surrounded
[41]. Therefore the different research settings could allow a
more generalizable interpretation of the results (Figure 6). |
A last methodological issue is related to the presence of multiple
tools in a CBLE. Multiple tools may lead to a switch cost in
learners. Aforementioned neuroimaging experiments were
suggested in order to explore this phenomenon by analyzing the
function of the brain. Neuroimaging experiments could provide
a more fine-grained insight on the effects of multiple tools
on tool use (Figure 6). However, neuroimaging is a relatively
new approach to research specially exploring the use of tools
in CBLEs. It is therefore a great challenge for future tool use
research. |
Concluding remarks |
In view of the results in this dissertation, a summary is presented
as follows. This summary along with the discussion from
Figure 6 provides a solid and ambitious research agenda on the
optimization of tool use and can be viewed as a guideline for
designers and researchers of CBLEs. |
• The use of tools is not only a problem pertaining to CBLEs. |
• The type of tools interacts with metacognitive
characteristics, such as perceptions and self-regulation
skills. Specifically, adjunct questions may require higher
critical thinking in learners and low perceived usability.
On the other hand, concept maps may require learners
with high perceived usability, high elaboration and low
performance avoidance, low perceived functionality and
low organization skills |
• Embedded tools may be the answer to guarantee tool use. |
• The explanation of the tool functionality did not influence
tool use nor influenced tool use negatively. |
• Metacognitive characteristics such as self-regulation skills
of critical thinking, elaboration and perceived usability
may be crucial to augment the time spent on the tools.
These characteristics should be carefully considered as
they seem to function in line with the types of tools. Some
learner characteristics may have a deeper impact on tool
use with certain types of tools than others. |
• Motivational characteristics such as self-efficacy and goalorientation
seem to be closely related and intervene on
tool use. Self-efficacy may affect tool use by influencing
perceived usability. Performance avoidance levels in
learners may be closely looked at –at least in research- as
it may hamper tool use. |
• Metacognitive and motivational characteristics seem to be
interrelated on the complexity of tool use. |
• Quality and frequency of tool use may not be adequate
measurements for tool use: Quality of tool use had an insignificant effect on tool use and frequency of tool use
affected performance negatively. |
• Spending enough time on the tools may in the end the right
answer to optimize tool use by the learner and guarantee
the tools functionality |
Additionally, these results provide important insights into two
theoretical research frameworks. First, these findings sustain
the research paradigm of aptitude-treatment interaction (ATI)
[42,43]. The ATI pictures complex relations between the learner
and the tools for which little empirical verification is found
[44]. The ATI theory points out that instructional strategies
or treatments, namely tools, interact with aptitudes which are
defined as any measurable characteristic of the learner, in this
case learner-related characteristics [45]. |
Second, these findings sustain the Carroll model [46,47]. In
Carroll’s Model, performance is a function of the ratio of the
time actually spent on learning to the time needed to learn which
has been put by McIlrath & Huitt in a simple equation [47,48].
This equation is illustrated below; ‘f’ corresponds to degree of
learning: |
Learning = f (time spent/ time needed) |
Time spent is the result of the time available to learn (opportunity)
and the time a learner is willing to spent (perseverance)
[48]. Time needed is dependent on the time needed to learn
(aptitude), and achievement which is the ability to understand
the instruction and the quality of instruction [48]. Considering
this model, positive effects of tool use on performance, may
therefore merely depend on the amount of time learners spent
on the tools. However, time should not be viewed in a simplistic,
sheer manner. Carroll himself has indicated that “time as such
is not what counts, but what happens during that time” [47].
Hence optimizing the learning time is an important factor to
improve performance. A challenge for future research is to find
out the amount of time learners with different characteristics
need to spend on the tools in order to reach better performance
levels. The use of log files in this dissertation allowed more finegrained
yet challenging results. These results set a direction to
further investigate time spent on tools with more granularity.
After all, the main aim is to optimize tool use and performance
by unraveling the use of tools [49,50]. |
Figures at a glance |
 |
 |
 |
Figure 1 |
Figure 2 |
Figure 3 |
|
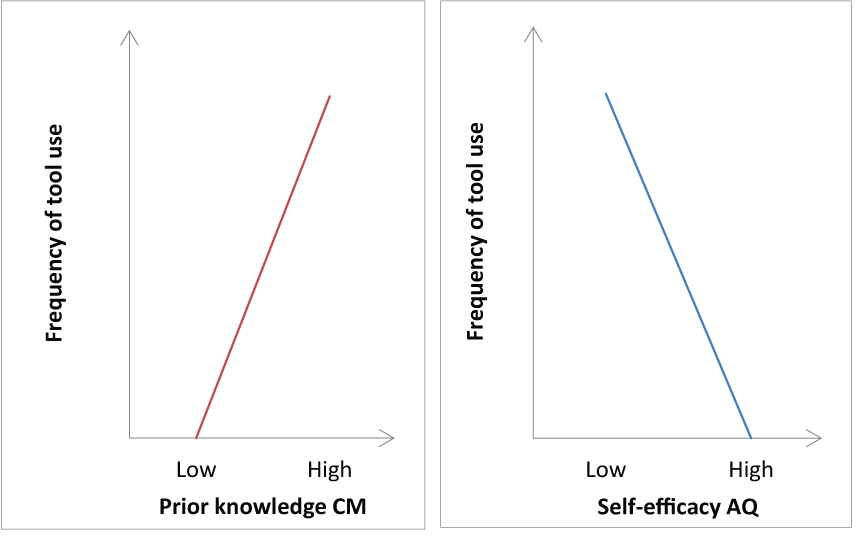 |
 |
 |
Figure 4 |
Figure 5 |
Figure 6 |
|
References |
- Viau R and Lariv̮̩e J, âÃâ¬ÃÅLearning tools with hypertext: An experiment,âÃâ¬Ã Computers & Education, Vol. 20, pp. 11-16, 1993.
- Falbel A, The computer as a convivial tool. In I. Harel& S. Papert (Eds.), Constructionism. Norwood, NJ: Ablex Publishing, 1991.
- Clarebout G and Elen J, âÃâ¬ÃÅTool use in computer-based learning environments: Towards a research framework,âÃâ¬Ã Computers in Human Behavior, vol. 22, no. 5, pp. 389-411, 2006.
- Perkins DN, âÃâ¬ÃÅThe fingertip effect: How information-processing technology shapes thinking,âÃâ¬Ã Educational Researcher, vol. 14, no. 7, pp. 11-17, 1985.
- Elen J and Clarebout G,âÃâ¬ÃÅSupport in Learning Environments: Touching the Limits of Instructional Design,âÃâ¬Ã Educational Technology, vol. 45, no. 5, pp. 44-47, 2005.
- Kim B and Reeves TC, âÃâ¬ÃÅReframing research on learning with technology: In search of the meaning of cognitive tools,âÃâ¬Ã Instructional Science: An International Journal of the Learning Sciences, vol. 35, no. 3, pp. 207-256, 2007.
- Winne PH, âÃâ¬ÃÅHow software technologies can improve research on learning and bolster school reform,âÃâ¬Ã Educational Psychologist, vol. 41, no. 1, pp. 5-17, 2006.
- Iiyoshi T and Hannafin MJ. Cognitive tools for open-ended learning environments: Theoretical and implementation perspectives. Paper presented at the Annual Meeting of the American Educational Research Association, San Diego, CA, USA, 1998.
- Alexander PA and Murphy PK, âÃâ¬ÃÅProfiling the differences in students' knowledge, interest, and strategic processing,âÃâ¬Ã Journal of Educational Psychology, vol. 90, no. 3, pp. 435-447, 1998.
- Dochy FJRC and Alexander PA. âÃâ¬ÃÅMapping prior knowledge: A framework for discussion among researchers,âÃâ¬Ã European Journal of Psychology of Education, vol.10, no.3, pp.225-242, 1995.
- Renkl A, âÃâ¬ÃÅWorked-out examples: Instructional explanations support learning by self-explanations,âÃâ¬Ã Learning and Instruction, vol.12, no. 5, pp.529-556, 2002.
- Wood H, and Wood D, âÃâ¬ÃÅHelp seeking, learning and contingent tutoring,âÃâ¬Ã Computers & Education, vol.33, no.2âÃâ¬Ãâ3, pp.153-169, 1999.
- Azevedo R, Guthrie JT, and Seibert D,âÃâ¬ÃÅThe role of self-regulated learning in fostering students' conceptual understanding of complex systems with hypermedia,âÃâ¬Ã Journal of Educational Computing Research, vol.30 no.1-2, pp.87-111, 2004.
- Juarez Collazo NA, Lust G, Elen J, and Clarebout G, âÃâ¬ÃÅTool use in a psychomotor task: The role of tool and learner variables,âÃâ¬ÃÂInternational Journal of Instruction, vol.4, no.2, pp.139-160, 2011.
- Juarez Collazo NA, Corradi D, Elen J, andClarebout G, âÃâ¬ÃÅTool use of experienced learners in computer-based learning environments: Can tools be beneficial?âÃâ¬Ã Higher Education Studies, vol.4 no.1, pp26-42, 2014.
- Juarez Collazo NA, Jiang L, Elen J, and Clarebout G, âÃâ¬ÃÅTool use and performance: Relationships between tool- and learner-related characteristics in a computer-based learning environment,âÃâ¬Ã Turkish Online Journal of Educational Technology (TOJET), vol.2, no.2, pp.330-345, 2013.
- Wild KP, and Schiefele U,âÃâ¬ÃÅLernstrategienimstudium. Ergebnissezurfaktorenstruktur und reliabilitÃÆÃ¤teinesneuenfragebogens [Learning strategies in academic studies. Results about factor structure and reliability of a new questionnaire],âÃâ¬ÃÂZeitschriftfÃÆÃ¼rDifferentielle und DiagnostischePsychologie, vol.15, pp.185-200, 1994.
- Davis FD,âÃâ¬ÃÅPerceived usefulness, perceived ease of use, and user acceptance of information technology,âÃâ¬Ã MIS Quarterly, vol.13, no.3, pp.319-340,1989
- Goodwin NC,âÃâ¬ÃÅFunctionality and usability,âÃâ¬Ã Communications of the ACM,vol.30, no.3, pp.229-233, 1987.
- Elliot AJ, McGregor HA, âÃâ¬ÃÅA 2 X 2 achievement goal framework,âÃâ¬Ã J PersSocPsycholvol. 80, pp. 501-519, 2001.
- Berliner DC,âÃâ¬ÃÅWhatâÃâ¬Ãâ¢s all the fuss about instructional time? In M. Ben-Peretz& R. Bromme (Eds.),âÃâ¬Ã The nature of time in schools: Theoretical concepts, practitioner perceptions. New York: Teachers College Press, pp. 3-35, 1990.
- Crippen KJ, Biesinger KD, Muis KR, and Orgill M, âÃâ¬ÃÅThe role of goal orientation and self-efficacy in learning from web-based worked examples,âÃâ¬Ã Journal of Interactive Learning Research, vol.20, no.4, pp.385-403,2009.
- Elen J, and Clarebout G, âÃâ¬ÃÅThe use of instructional interventions: Lean learning environments as a solution for a design problem,âÃâ¬Ã In J. Elen& R. Clark (Eds.), Handling complexity in learning environments: Theory and research. Advances in Learning and Instruction Amsterdam: Elsevier, pp.185-200, 2006
- Clarebout G, Horz H, Schnotz W, and Elen J âÃâ¬ÃÅThe relation between self-regulation and the embedding of support in learning environments Educational Technology,âÃâ¬Ã Research and Development,vol.58, no.5, pp.573-587,2010.
- Jiang L, and Elen J, âÃâ¬ÃÅInstructional effectiveness of higher-order questions: The devil is in the detail of studentsâÃâ¬Ã⢠use of questions,âÃâ¬Ã Learning Environments Research, vol.14, no.3, pp.279-298,2011.
- Greene BA and Land SM, âÃâ¬ÃÅA qualitative analysis of scaffolding use in a resource-based learning environment involving the world wide web,âÃâ¬Ã Journal of Educational Computing Research, vol.23,no.2, pp. 151-179, 2000.
- Huet N, Escribe C, Dupeyrat C, and Sakdavong JC, âÃâ¬ÃÅThe influence of achievement goals and perceptions of online help on its actual use in an interactive learning environment,âÃâ¬ÃÂComputers in Human Behavior, vol.27, no.1, pp.413-420,2011.
- Aleven V, Stahl E, Schworm S, Fischer F and Wallace RâÃâ¬ÃÅHelp seeking and help design in interactive learning environments,âÃâ¬Ã Review of Educational Research, vol.73, no.3, pp.277-320, 2003.
- van Gog T, Paas F, Marcus N, Ayres P, and Sweller J âÃâ¬ÃÅThe mirror neuron system and observational learning: Implications for the effectiveness of dynamic visualizations,âÃâ¬Ã Educational Psychology Review, vol.21, no.1, pp.21-30, 2009.
- Biederman I, âÃâ¬ÃÅHuman performance in contingent information-processing tasks,âÃâ¬Ã J ExpPsycholvol. 93, pp. 219-238, 1972.
- Jersild AT, âÃâ¬ÃÅMental set and shift,âÃâ¬Ã Archives of Psychology, vol.14, no.89, pp. 81, 1927.
- Monsell S, âÃâ¬ÃÅTask switching,âÃâ¬Ã Trends in Cognitive Sciences,âÃâ¬ÃÂvol.7, no.3, pp.134-140, 2003.
- >âÃâ¬ÃÅMultitasking: Switching costs Subtle "switching" costs cut efficiency, raise risk,âÃâ¬Ã Retrieved from https://www.apa.org/research/action/multitask.aspx2006.
- Given LMâÃâ¬ÃÅIntroduction In L. M. Given (Ed.), The SAGE Encyclopedia of Qualitative Research MethodsâÃâ¬Ã London: SAGE Publications, Vol. 2, 2008.
- Lust G, Juarez CollazoNA, Elen J, and Clarebout G âÃâ¬ÃÅContent management systems: Enriched learning opportunities for all?âÃâ¬Ã Computers in Human Behaviorvol.28, no.3, pp.795-808,2012.
- Lawless KA, and Brown SW,âÃâ¬ÃÅMultimedia learning environments: Issues of learner control and navigation,âÃâ¬Ã Instructional Science, vol.25, no.2, pp.117-131,1997.
- Jiang L, âÃâ¬ÃÅInstructional effectiveness of scaffolds: Role of learner variables,âÃâ¬Ã Unpublished doctoral dissertation, KatholiekeUniversiteit Leuven, Leuven. 2010.
- SchnotzW, and Heiss, âÃâ¬ÃÅA Semantic scaffolds in hypermedia learning environments,âÃâ¬Ã Computers in Human Behavior, vol.25, no.2, pp. 371-380,2009.
- Creswell J, âÃâ¬ÃÅEducational research: Planning, conducting, and evaluating quantitative and qualitative research,âÃâ¬Ã Boston: Pearson. 2004.
- Caruth GD, âÃâ¬ÃÅDemystifying mixed methods research design: A review of the literature,âÃâ¬ÃÂMevlana International Journal of Education (MIJE), vol.3, no.2, pp.112-122, 2013.
- Bhattacharya H, âÃâ¬ÃÅResearch setting. In L. M. Given (Ed.), The SAGE Encyclopedia of Qualitative Research Methods Vol. 2, pp. 787-788, London: SAGE Publications. 2008.
- Cronbach LJ,âÃâ¬ÃÅThe two disciplines of scientifc psychology,âÃâ¬Ã American Psychologist, vol.12, no.11, pp.674-684, 1957.
- Cronbach LJ and Snow, âÃâ¬ÃÅRE Aptitudes and instructional methods. A handbook for research on interactions,âÃâ¬Ã New York: Irvington Publishers, Inc. 1977.
- Shute V, and Towle B, âÃâ¬ÃÅAdaptive e-learningâÃâ¬Ã Educational Psychologist, vol.38, no.2, pp.105 âÃâ¬Ãâ 114, 2003.
- Regian JW and Shute VJ, âÃâ¬ÃÅCognitive approaches to automated instruction,âÃâ¬Ã Hillsdale, NJ: Lawrence Erlbaum Associates, 1992.
- Carroll JB, âÃâ¬ÃÅA model of school learning,âÃâ¬Ã Teachers College Record, vol.64, pp.723-733,1963.
- Carroll JB, âÃâ¬ÃÅThe carroll model: A 25-year retrospective and prospective view,âÃâ¬Ã Educational Researcher, vol.18, no.1, pp.26-31,1989.
- McIlrath D and Huitt W, âÃâ¬ÃÅThe teaching-learning process: A discussion of models,âÃâ¬Ã Educational Psychology Interactive Retrieved March 24th, 2014, from http://www.edpsycinteractive.org/papers/modeltch.html 1995.
- Nouwen A, Urquhart Law G, Hussain S, McGovern S, Napier H et al., âÃâ¬ÃÅComparison of the role of self-efficacy and illness representations in relation to dietary self-care and diabetes distress in adolescents with type 1 diabetes,âÃâ¬ÃÂPsychol Health vol. 24, pp. 1071-1084, 2009.
- Murphy PK, Alexander PA, âÃâ¬ÃÅA Motivated Exploration of Motivation Terminology,âÃâ¬Ã ContempEducPsycholvol. 25, pp. 3-53, 2000.
|