Keywords
|
Apriori, Parallel processing, Java Concurrency Library |
INTRODUCTION
|
Data mining is a technique that used to discover hidden and useful information from data warehouses. Frequent pattern mining is one important technique of data mining. Frequent itemset is a set of items that appear frequently together in a transaction data set. To develop scalable methods for mining frequent itemsets in a large transaction dataset is a great challenge. Frequent pattern mining was first proposed by Agrawal et al. [1] for market basket analysis in the form of association rule mining. Apriori algorithm has an interesting downward closure property, among frequent k-itemsets: A k-itemset is frequent only if all of its sub-itemsets are frequent [2]. Since the Apriori algorithm was proposed, there have been extensive studies on the improvements or extensions of Apriori. |
RELATED WORK
|
Apriori is most popular frequent itemset generation mining algorithm that proposed by Agrawal et al [1]. Parallel and distributed algorithms for association mining are proposed by Park JS et al [8] to handle the scalability problem of sequential algorithms. The count distribution and data distribution parallel association algorithms proposed by Rakesh Agrawal, John C.Shafer proposed [9]. Cheung DW et al [10] proposed the efficient and effective distributed algorithm FDM (Fast Distributed Mining) for mining the association rules that proposed local and global powerful pruning techniques for improving the performance. Li Liu2 et al [11] design a multi-core processor optimization algorithm for FP-Tree using a cache-conscious FP-array and a lock free dataset tiling parallelization mechanism. |
APRIORI ALGORITHM
|
The Apriori algorithm concentrates primarily on the discovery of frequent itemsets according to a user-defined minSup [2]. An itemset is called a frequent itemset when its support is greater than or equal to the minSup threshold; otherwise, it is an infrequent itemset. In the first pass, the Apriori algorithm constructs and counts all 1-itemsets. (A k-itemset is an itemset that includes k items.) After it has found all frequent 1-itemsets, the algorithm joins the frequent 1-itemsets with each other to form candidate 2-itemsets. Apriori scans the transaction dataset and counts the candidate 2-itemsets to determine which of the 2-itemsets are frequent. The other passes are made accordingly. Frequent (k-1)-itemsets are joined to form k-itemsets whose first k-1 items are identical. Apriori remove some of the k-itemsets those (k-1)- itemsets have at least one infrequent subset. All remaining k-itemsets constitute candidate k-itemsets. The process is reiterated until no more candidates can be generated [2, 3]. Pseudo code of Apriori algorithm is given below [4]. |
Ck: Candidate itemset of size k |
Lk : frequent itemset of size k |
L1 = {frequent items}; |
for (k = 1; Lk !=Φ; k++) do begin |
Ck+1 = candidates generated from Lk; |
for each transaction t in dataset do |
increment the count of all candidates in Ck+1 that are contained in t |
Lk+1 = candidates in Ck+1 with min_support |
end |
return Uk Lk; |
The Apriori algorithm significantly reduces the size of candidate itemsets using his closure property. However, it can generate a larger number of candidate itemsets, and multiple times scanning the database that is time consuming process and reduce the performance. Sometime Apriori is hang-up for the frequent itemset generation from large transactional dataset. This problem can be overcome using Apriori algorithm implement in scalable Multi-core processor parallel computing. |
PARALLEL COMPUTING USING JAVA CONCURRENCY LIBRARIES
|
Parallel computing utilized the available multi core processor of system that reduce the execution time and increase performance [5, 6]. A multi core processor implement message passing or shared memory inter core communication methods for multiprocessing. Any application that can be threaded can be mapped efficiently to multi-core, but the improvement in performance gained by the use of multi core processors depends on the fraction of the program that can be parallelized [5]. |
The Java provides multi threading feature that enable to write concurrent applications where different threads execute simultaneously. The Java Concurrency framework is a library that is designed to be used as building blocks for creating concurrent applications. It is facilitate threading tasks especially on multi-core systems. java.util.concurrent package defines the executor framework which provides own thread management and scheduling scheme. Executor separates task execution and submission, and provides hooks for thread life-cycle monitoring and control. Callable, Future, Executer, ExecuterService and ScheduledExecutorService are the main interfaces of concurrent framework environment [7]. |
• Callable interface is similar to Runnable interface but difference is a call () method is return a value while run () method no return a value. |
• Future represents the result of asynchronous computation. There are methods to check if computation is completed, wait for it to be finished, get the results of computation, and cancel the computation. |
• Executor is an interface for object to execute submitted tasks. This interface decouples the task submission from the task execution, and it is used instead of creating threads explicitly. For example, in the following code the DirectExecutor executes tasks in serial, and ThreadPerTaskExecutor executes every task in separate thread. Task submission is done similarly in both cases. |
• ExecutorService is an Executor that provides methods to manage the termination (shut down), task tracking and returning the Future of task which can be used to cancel execution, wait for completion, track the progress of the task. |
• ScheduledExecutorService is an ExecutorService which allows scheduling commands to be run after a delay, or to be executed periodically. |
MULTI-CORE PROCESSING APRIORI ALGORITHM
|
Step 1: Generate unique itemset (first candidate set) from a transactional dataset. |
Step 2: Create a multiple threads (equal to number of processor in computer) using Executors object. |
Step 3: Submit each itemset in CompletionService object for support count. |
Step 4: Calculated support count value and stored in variable. |
Step 5: After calculation of support count generate a frequent itemset using minSup value. |
Step 6: Prepare a new candidate itemset by increasing one item. |
Step 7: Repeat step-5 to step-6 until frequent itemset is empty. |
Step 8: End. |
SIMULATION RESULTS
|
In order to evaluate the performance of Apriori and parallel Apriori algorithms, we performed experiment on two different datasets. Dataset-1 has 3000 and Dataset-2 has 10000 transactional records. Algorithms run for both transactional datasets file with various minSup values. Require program file written in Java. Algorithms run on multicore processor computer that configuration is Intel Core i5 3.20GHz (Four Processor), 4GB RAM and 3MB Cache memory. Table-1 shows the result metric; total requires execution time for both transactional datasets with various minSup values. |
Figure-1 presents the comparison of algorithm results require execution (in seconds) for Dataset-1 with various minSup values of 250, 350, 450 and 550. From the figure-1, we can say Parallel Apriori algorithm take a less time compare to simple Apriori algorithm. |
Figure-2 presents the comparison of algorithm results require execution (in seconds) for Dataset-2 with various minSup values of 700, 900, 1100 and 1300. From the figure-2, we can say Parallel Apriori algorithm take a less time compare to simple Apriori algorithm. |
CONCLUSION
|
In this paper, we are proposing a work of parallel computing Apriori algorithm using Java concurrency package. We ran algorithms on a multi core processor with two datasets using various minSup. We measure the simple and parallel Apriori algorithm performance on multi core processor with respect to time. The parallel computing Apriori algorithm takes the less time as compare to the simple Apriori algorithm. |
Tables at a glance
|
 |
Table 1 |
|
|
Figures at a glance
|
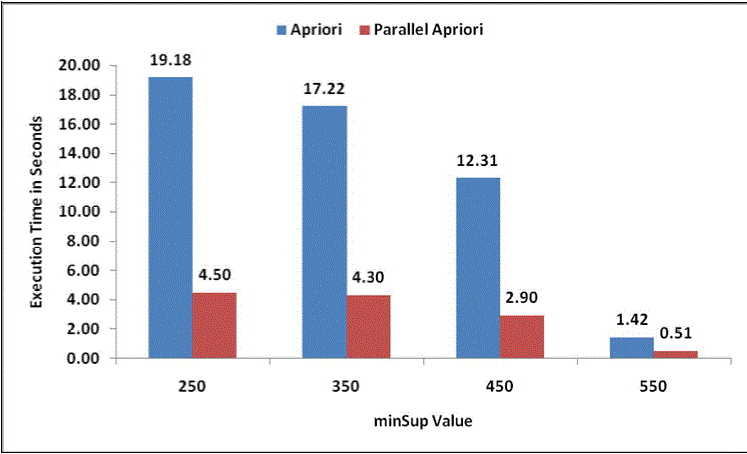 |
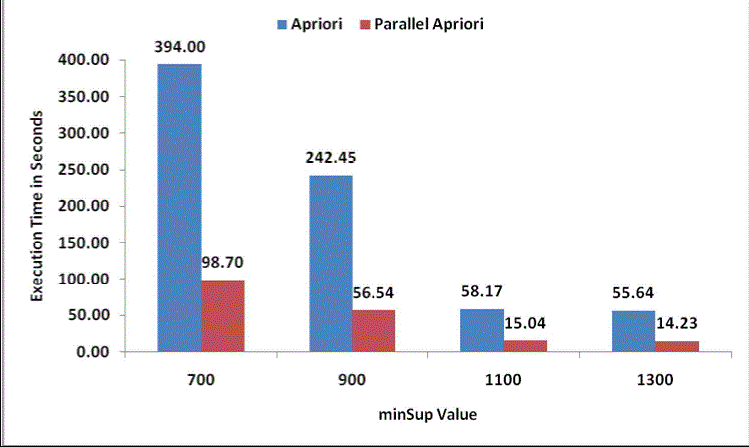 |
Figure 1 |
Figure 2 |
|
|
References
|
- Agrawal R, Imielinski T, Swami A, “Mining association rules between sets of items in large databases”, Proceedings of the 1993ACMSIGMODinternational conference on management of data (SIGMOD’93), pp 207–216, 1993.
- Agrawal R, SrikantR , “Fast algorithms for mining association rules. In: Proceedings of the 1994 international conference on very large data bases (VLDB’94), pp 487–499, 1994.
- Chin-Chen Chang, Yu-Chiang Li, Jung-San Lee, “ An Efficient Algorithm for Incremental Mining of Association Rules”, Proceedings of the 15th International Workshop on Research Issues in Data Engineering: Stream Data Mining and Applications (RIDE-SDMA’05), 2005
- Jiawei Han, MichelineKamber, “Data Mining: Concepts and Techniques (Second Edition)”, ISBN 13: 978-1-55860-901-3, Elsevier, 2006
- Amdahl, Gene, “Validity of the Single Processor Approach to Achieving Large-Scale Computing Capabilities”. AFIPS Conference Proceedings, pp.483–485, 1967.
- Anuradha.T, SatyaPasad R and S N Tirumalarao, “Parallelizing Apriori on Dual Core using OpenMP”, International Journal of Computer Applications, pp:33-39, 2012.
- http://docs.oracle.com/javase/7/docs/api/java/util/concurrent/package-summary.html, concurrent package API specification for the Java™ Platform, Standard Edition.
- Park JS, Chen MS, Yu PS, “Efficient parallel mining for association rules”, Proceeding of the 4th international conference on information and knowledge management, pp 31–36, 1995
- Agrawal R, Shafer JC, “Parallel mining of association rules: design, implementation, and experience”, IEEE Trans Knowl Data Eng , pp:962–969, 1996.
- Cheung DW, Han J, Ng V, Fu A, Fu Y, “A fast distributed algorithm for mining association rules”, Proceeding of the 1996 international conference on parallel and distributed information systems, pp 31–44, 1996.
- Li Liu2, 1, Eric Li1, Yimin Zhang1, Zhizhong Tang, “Optimization of Frequent Itemset Mining on Multiple-Core Processor” VLDB =07, Vienna, Austria, 2007.
|