Keywords
|
Mobile Adhoc Network (MANET), Genetic Algorithm (GA), Least Delay Multicast Tree Alorithm(LDT) |
INTRODUCTION
|
Mobile Ad Hoc Networks (MANETs) are groups of self-organizing mobile nodes in dynamic topology networks. MANETs do not need infrastructure units such as base stations or access points in advance. Each movable node in the MANETs has a routing function whereby it communicates by forwarding datagram’s via intermediate nodes. If two movable nodes are located within the forwarding range, they communicate with each other directly. Otherwise, they need another node to forward their datagram’s and require a datagram forwarding operation using a multi-point hopping method. MANETs are characterized by non-restricted mobility and easy deployment, which makes them very popular. |
The AODV routing protocol is a reactive routing protocol; therefore, routes are determined only when needed. Hello messages may be used to detect and monitor links to neighbors. If Hello messages are used, each active node periodically broadcasts a Hello message that all its neighbors receive. Because nodes periodically send Hello messages, if a node fails to receive several Hello messages from a neighbor, a link break is detected. When a source has data to transmit to an unknown destination, it broadcasts a Route Request (RREQ) for that destination. At each intermediate node, when a RREQ is received a route to the source is created. If the receiving and does not have a current route to the destination, it rebroadcasts the RREQ. If the receiving node is the destination or has a current route to the destination, it generates a Route Reply (RREP). The RREP is unicast in a hop-by hop fashion to the source. As the RREP propagates, each intermediate node creates a route to the destination. When the source receives the RREP, it records the route to the destination and can begin sending data. If multiple RREPs are received by the source, the route with the shortest hop count is chosen. |
As data flows from the source to the destination, each node along the route updates the timers associated with the routes to the source and destination, maintaining the routes in the routing table. If a route is not used for some period of time, a node cannot be sure whether the route is still valid; consequently, the node removes the route from its routing table. |
If data is flowing and a link break is detected, a Route Error (RERR) is sent to the source of the data in a hop-by hop fashion. As the RERR propagates towards the source, each intermediate node invalidates routes to any unreachable destinations. When the source of the data receives the RERR, it invalidates the route and reinitiates route discovery if necessary. |
In general, the design of a QoS multicast routing protocol with multi-constrained metrics has not always taken into consideration the consumption of battery energy. Thus, upon operation of the whole network, some mobile nodes can have problems with energy overhead due to a lack of balance in their battery energy consumption. Once these selected nodes in the multicast tree run out of residual battery energy, an interruption condition arises that is generated in the link during packet forwarding. Thus, there are numerous interruptions that can occur in selected packet forwarding routing paths. The multicast service lifetime will not be maintained continuously until the completion of packet forwarding. The idea is to maximize the duration so that all mobile nodes are up until one of them is drained of energy. |
The QoS items that are called metrics include available bandwidth, end-to-end delay, probability of packet loss, delay variance (jitter), expense, and so on. Since different items have different properties, they could be classified into three categories, namely additive, multiplicative, and minimal properties. Many relevant references which have been cited in multicast routing topics usually tackle some of the general QoS metrics such as the bandwidth, packet loss rate, and propagation delay. |
A genetic algorithm (GA) is a searching algorithm that utilizes the genetic operators, for examples, crossover and mutation. It emulates the evolution idea using natural selection and the survival of the fittest concept. In each generation, a new population of solutions is created by exchanging and combining the information obtained from the solutions through the previous generation. Crossover is one of the genetic operators in which genes from two chromosomes are exchanged and the genotypes of two selected parents are merged to make two new offspring. Crossover is also referred to as recombination and sometimes called mating. Two chromosomes with greater fitness values are picked from the chromosome pool. The starting point and length of the portion to be exchanged are randomly selected. |
The two new offspring are created and put back into the chromosome pool. Mutation is another genetic operator in which new genetic structures are inducted into the population by randomly modifying some of the genes. Mutation also maintains the search algorithm to escape from local optimum and prevents converging too fast. In other words, mutation operation gives the genetic algorithm an opportunity to search for new and more feasible chromosomes in new areas of solution space. After the mutation operation, the multicast tree will be modified because the mutation operator can destroy the tree structure and outgoing degree constraints. The other genetic algorithm operations include encoding, initial population, evaluation, reproduction, crossover and mutation. |
BACKGROUND
|
Among all the delay-constrained multicast routing algorithms, least delay multicast tree algorithm (LDT) has the highest successful ratio because it connects the source and each destination with the least delay path. The successful ratio (SR) of an algorithm is defined as the number of requests successfully routed divided by the total number of routing requests. When the multicast tree constructed by the algorithm satisfies the delay constraint, the routing request is considered as successfully routed one.LDT has lowest delay comparatively AODV protocol and also successful ratio as well. LDT also low cost compare to AODV protocol |
Each node in a MANET determines the distance between itself and its neighbor nodes using some distance estimation method. All nodes use omni-directional antennas. The connectivity of the network depends on the transmission power of each node. Each node can dynamically change its transmission power level. A node can use a different power level for each multicast tree in which it participates. |
There are some limitations in the Least Delay multicast Tree algorithms that are Running time of LDT is very high, if 100 nodes in the network the running time very high, The average cost of LDT is much higher than the proposed genetic algorithm and also Packet delivery ratio and Through put has much lower than the proposed genetic algorithm. |
PROBLEM DEFINITION
|
Genetic algorithm originated from the concept of natural selection and natural genetic, considers the set of all possible solutions, called population. As the genetic algorithm has to build a population of solutions, it also acts as a searching algorithm for identifying the possible solutions. Encoding, crossover and mutation are the three operations available in the field of genetics. The outcome of an iteration of genetic algorithm is considered as a new generation and a new set of solutions is obtained by the combination and exchange of the data available from the preceding generation. The variables used in this algorithm are the genes present in a chromosome. Every chromosome gene denotes a probable solution. |
Genetic algorithm contains the following processes: |
• Extended ST Encoding |
• Initial Population |
• Fitness Evaluation |
• Selection of Parents |
• Crossover Scheme |
• Mutation |
Extended ST Encoding
|
In this encoding method, integer strings called chromosomes, denote possible solutions that form a population. First, the nodes of the multicast tree should be given position indexes (by generally following in-order tree traversal). Encoding an indexed tree of n nodes using this method generates two strings s and t, each containing n integers. The sequence encoding of the multicast tree is denoted by the string s, whereas the topology encoding is denoted by the string t. |
Initial Population
|
In genetic algorithm, initially, a set of feasible multicast trees (at least two) are generated for the network topology considered by using prim’s algorithm. These trees are then encoded using any one of the encoding techniques. So, each encoded string produced by encoding a multicast tree represents a multicast route. The strings that are generated from encoding process form initial population for the genetic algorithm to proceed with other operations like selection, crossover and mutation .This initial population acts as a base on which future generations are produced by reproduction of the fittest ones among them. |
Fitness Evaluation and Selection
|
After the initial population is formed, the ones that survive better are chosen. For this selection, their fitness must be evaluated. Hence, a method (fitness function) is necessary in order to calculate the fitness of each solution in the population. This fitness function evaluates the efficiency offered by each individual solution in meeting the requirements (metrics like residual battery energy, delay and bandwidth) of the group application that is being developed. By selection process, the best ones are chosen for further iteration. But performing only these processes will not ensure in obtaining global optimality. The further steps of crossover and mutation will allow the solutions to exchange their information and hence search for paths that are more optimal. |
Crossover Scheme
|
The term crossover is used extensively in genetic engineering. This notion can even be applied in finding paths that are more suitable, satisfying the specified constraints. In this method, the selected trees are combined to give rise to new tree. It divides up the chromosomes of parent trees and recombines them. The parent chromosomes that are selected for crossover from the chromosomes pool are the ones that are having greater fitness. The initial point and length of the segment of the parent chromosomes that are swapped and merged are chosen in a random way. The new child chromosome formed by crossover is added to the chromosomes pool. |
Mutation
|
During the crossover of the parent trees, a child tree inherits the characteristics of the parents. This child tree undergoes mutation phase. Mutation is a process in which chromosome alternation i.e. reorganizing the genes of the chromosome is done in order to achieve specific characteristic in the organism. In this method, some of the node ids of the child multicast tree are rearranged in random way producing a new genetic structure. This feature of genetic algorithm prevents it from rapid convergence. Hence, mutation results in the generation of new multicast tree since the child produced after crossover is modified in this phase. The new tree is added to the population and the iteration continues until an optimal multicast tree is obtained. |
PERFORMANCE EVALUATION
|
In proposed Genetic algorithm, used to analysis the parameters such as Packet Delivery Ratio (PDR), Energy Spent and end to end delay. In that compare the results with other protocols like Adhoc on Demand Vector (AODV) and Least Delay Multicast Tree algorithm (LDT). |
In Fig. 2, AODV has maximum delay comparatively to LDT and proposed Genetic Algorithm. And also LDT is almost equal to proposed Genetic Algorithm which ensures the proposed GA used minimum end to end delay. |
In Fig. 3, LDT has high throughput comparatively to AODV and also proposed Genetic Algorithm has much higher throughput comparatively to both LDT and AODV. This analysis shows that proposed Genetic Algorithm has high throughput. It is obvious that the two algorithms have the same SR. This proves that the proposed algorithm can find the feasible multicast trees that satisfy the delay bound if one exists |
In Fig. 4, LDT has high Packet Delivery Ratio comparatively to AODV and also proposed Genetic Algorithm has much high Packet Delivery Ratio comparatively to both LDT and AODV. This analysis shows that proposed Genetic Algorithm has maximum packet Delivery Ratio. |
CONCLUSION
|
This genetic algorithm is a source-based algorithm which takes into account energy consumption as well as end-toend delay in route selection. The proposed algorithm applies crossover and mutation operations directly on trees, which simplifies the coding operation and omits the coding/decoding process. Heuristic mutation technique can improve the total energy consumption of a multicast tree. A series of experiments was performed to verify the convergence performance, End to End Delay and Energy spent of the proposed algorithm. The results demonstrate that this genetic algorithm is effective and efficient. |
Figures at a glance
|
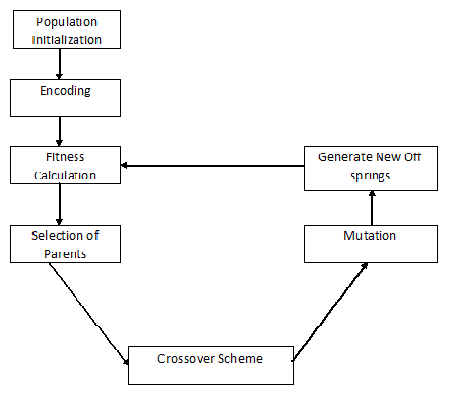 |
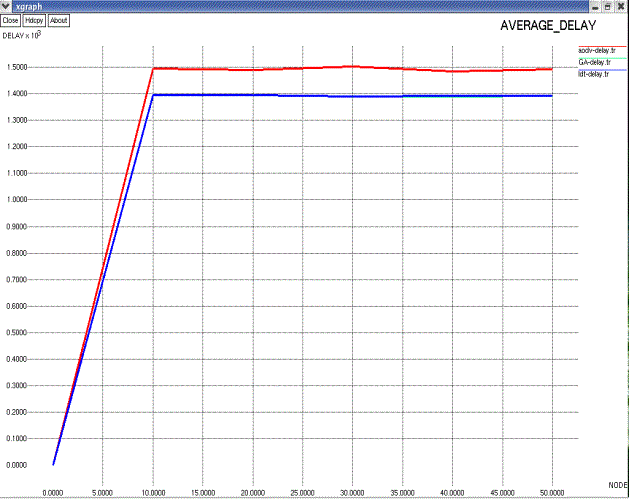 |
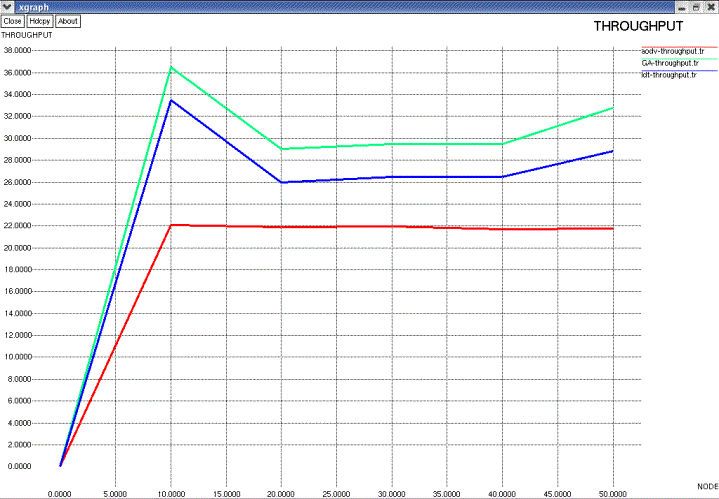 |
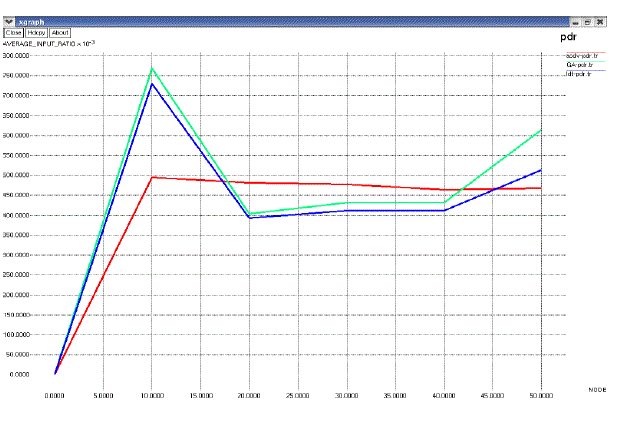 |
Figure 1 |
Figure 2 |
Figure 3 |
Figure 4 |
|
References
|
- Ahmadi F.,Tati R.,AhmadiS.andHossaini V. ,“ New hardware engine for genetic algorithms,” in Proc. ICGEC, pp. 122–126,2011.
- Feeney L.M. and Nilsson M.,“ Investigating the energy Consumption of a wireless network interface in an ad hoc networking Environment ”, in Proc.INFOCOM, pp. 1548–1557,2001.
- Haghighat A.T., Faez K., Dehghan M. and Ghahremani Y., “A genetic algorithm for Steiner tree optimization with multiple constraints using Prufer number,” Proc. EurAsia-ICT, pp. 272–280,2002.
- Lee W. C. Y., “Mobile Communication Engineering”, McGraw-Hall, 1993.
- Molnr M., Bellabas A. and Lahoud S., “The cost optimal solution of the multi-constrained multicast routing problem”, Computer Networks, vol.13, no.13, pp. 3163–3149,2012.
- Nutov and Segal M., “Improved approximation algorithms for maximum Life time problems in wireless networks”, Theoretical Computer Science, vol. 453, no. 28, pp. 88–97,2012.
- Priti Gaur., “Implementation of Multicast Routing Using Genetic Algorithm”, pg.226 – 234,2013,1998.
- Ravikunmar C.P. and Bajpai R.,” Source-based delay-bounded multicasting in multimedia networks”, Computer Commun., vol. 21, no. 2, pp.126–132
- Suresh Kumar J. and Babu Raj E., “Genetic Algorithm based Multicast Routing in Wireless Sensor Networks - A Research framework”, Volume 2,December 2012.
- Ting Lu and Jie Zhu., “Genetic Algorithm for Energy-Efficient QoS Multicast Routing”, VOL. 17,2013.
|