Keywords
|
Text Detection, Zero Cross Edge Detector, Harris Corner Detector, Background Subtraction, Image Morphological Dilation, Gradient Vector and Direction, Stroke Width |
INTRODUCTION
|
Text data present in images and video contain useful information for automatic annotation, indexing and structuring of images. There has been a growing demand for image and video data in applications due to the significant improvement in the processing technology, network subsystems and availability of large storage systems. The text detection stage seeks to detect the presence of text in a given image and video. If this text information can be extracted and harnessed efficiently, it can provide a much truer form of content–based access to images and videos. Hence, text detection from image and video is an important research topic in computer vision. These produced a wide variety of sources, including the long distance educational programs, medical diagnostic systems, business and surveillance applications, broadcast and entertainment industries, etc. Recently, with the increasing availability of low cost portable cameras and camcorders, the number of images and videos being captured are growing at an explosive rate. However, this is a very challenging task due to the presence of various fonts, colors, sizes, orientations, complex backgrounds, varying illuminations and distortions. |
However, such huge amount of images and videos make it increasingly difficult for us to locate specific images and videos of interest. Therefore, there is an urgent demand to develop a Content Based Information Retrieval (CBIR) system that can automatically index image and video documents efficiently based on their semantic contents. Toward this goal, much effort has been done and many algorithms have been proposed for CBIR systems in the literature. Although some impressive progress has been achieved in recent years, the existing CBIR systems are still far from perfect due to the semantic gap, which is defined as the discrepancy between machine-level descriptors using low-level image features (color, edge, texture, shape, spatial relationship, etc.) and semantic-level descriptors using high level semantic features (objects, events, logical inference, reasoning, abstract concept, etc.). For example, object ontology based methods that use spatial position, quantized color, and texture features can only describe the semantics of simple images without many contents. |
Text in image and video can be classified into two categories: caption text and scene text. Caption text is artificially overlaid on the images or video frames at the time of editing. Although some types of caption text are more common than others, it is observed that caption text can have arbitrary font, size, color, orientation, and location. For video document, a caption text event may remain stationary and rigid over time, or it may translate, grow or shrink, and change color. A good text extraction system must be able to handle as wide a variety of these types of text as possible in order to support a content-based indexing system. Text naturally occurring within the scene, or scene text, also occurs frequently in image and video. Examples include text appearing on vehicles (license plate, maker/model/dealer names, company names on commercial vehicles, bumper stickers etc.), text on signs and billboards, and text on T-shirts and other clothing. Technically, the extraction of scene text is a much tougher task due to varying size, position, orientation, lighting, and deformation. Contrast to a large amount of text extraction techniques for caption, only limited work is found in the literature that focuses on robust scene text extraction from images and videos. |
Generally, most image-based text extraction approaches can be used for video documents as well, since video can be considered as a sequence of images (frames). However, compared with still images, video has some unique properties that may affect the text extraction. On one side, video usually has low resolution, low contrast, and color bleeding caused by compression, which are undesirable characteristics for text extraction; on the other side, text in video typically persists for at least several seconds to give human viewers the necessary time to read it. This temporal redundancy of video is very valuable for text verification and text tracking. |
The present methods give advantage over different font, size, color and orientation, language independent and low average time spent on each pixel. The most important and widely used application of a text extraction system is textbased image/video indexing and retrieval by using the recognized outputs of the system. Besides that, many other applications of text extraction system have been developed as well, such as camera-based text reading system for visually impaired people, wearable translation robot, wearable text-tracking system, vehicle license plate recognition, and road sign text detection for mobile device. |
RELATED WORK
|
Text detection has been an active research topic for decades and review of all the text detection methods is impossible. So some papers related to the proposed system are mentioned as below: |
Jung K, Kim K and Jain A [1] provided a survey of text information extraction in images and videos. A classification of the different algorithms for text detection and localization is mentioned. They surveyed several localization methods such as region-based methods, Connected Component-based methods, Edge-based methods, Texture-based methods and Text Extraction in Compressed Domain. As single method implementation did not provide satisfactory performance hence integration of methods is done. |
Xu Zhao, Kai-Hsiang Lin, Yun Fu [2] proposed a corner based approach to detect text and caption from videos, because in each characters there exists dense and orderly presences of corner points. Several discriminative features were used to describe the text regions formed by the corner points whose usage can be extended different applications. An algorithm is implemented to detect moving captions in videos where motion features extracted by optical flow are combined with text features to detect the moving caption patterns. The proposed system detects video text with high precision and efficiency. Language independent is overcome by this proposed system. |
Wonjun Kim and Changick Kim [3] proposed a novel framework to detect and extract the overlay text from the video scene. Observations were made based on existence of transient colors between inserted text and its adjacent background. First, the transition map is generated based on logarithmical change of intensity and modified saturation. Linked maps are generated to make connected components for each candidate region and then each connected component is reshaped to have smooth boundaries. The transition pixels density and the consistency of texture around each transition pixels are computed to distinguish the overlay text regions from other candidate regions. The proposed method uses local binary pattern for the intensity variation around the transition pixel in the proposed. The proposed method is very useful for the real-time application. |
Jing Zhang and Rangachar Kasturi [4] presented a new unsupervised method to detect scene text objects by modelling each text object as a pictorial structure. For each character in text object the edges of a stroke are considered as a combination of two edge sets that have high similarities in length, orientation, and curvature. Based on this three new character features such as Average Angle Difference of Corresponding Pairs, Fraction of Non-noise Corresponding Pairs, and Vector of Stroke Width were introduced which captured the similarities of stroke edge pairs and energy calculation. Link energy is computed which describes the spatial relationship and property similarity between two neighbouring characters. Unit energy (Combination of character energy and link energy) is used to measure the probability that a candidate text model is a text object and generate final detection result. |
Yi-Feng Pan, Xinwen Hou, and Cheng-Lin Liu [5] presented a hybrid approach to robustly detect and localize texts in natural scene images. To estimate the text existing confidence and scale information in image pyramid, a text region detector is designed, which help segment candidate text components. Conditional random field (CRF) model considering unary component properties and binary contextual component relationships with supervised parameter learning is proposed for efficiently filter out the non-text components. Lastly, text components are grouped into text lines or words with a learning-based energy minimization method. As all the three stages are learning based, there are some parameters which require manual tuning. Also evaluation based on a multilingual image dataset proves efficient. Zhuowen Tu, Xiangrong Chen, Alan L. Yuille and Song-Chun Zhu [6] introduced a computational framework for parsing images into basic visual patterns. They proposed a rigorous way to combine segmentation with object detection and recognition. They implemented a model based on visual patterns including texture and shading regions, and objects (text and faces). They also integrated discriminative and generative methods of inference so as to overcome drawbacks of each other and to construct a parse graph representing image. |
Chucai Yi and YingLi Tian [7] proposed a new approach to locate text regions embedded in images based on image partition and connected components grouping. From text characters to text strings, a structural analysis is performed. Using gradient feature and color feature, the candidate text characters are chosen from connected components. Then character grouping is performed to combine the candidate text characters into text strings which contain at least three character members in alignment. Experiments proved that color-based partition performs better than gradient-based partition. |
PROPOSED SYSTEM
|
A) Different variation in text of Images and videos |
Text in images and videos can exhibit many variations in images and videos. These variations lead to many problems for detecting text in images and videos. There are mainly four types of variation in text of images and videos. |
i) Geometry of text: |
In text detection of image and video, the geometry of text is the main accruing problem. The size of text variation, alignment of text and the inter character distance are showing geometry of text. Hence it is difficult to detect very small text in image and video. If an image contain repeating patterns that is if the text have repeated word than it is difficult to detect word. Mainly geometry of text in images and videos has Size: - In an image or video frame, the sizes of character have different length or width. Hence it is very hard to detect small character in image and video frames. The image or video having size variation may be Variation in sizes and small character. |
Alignment: - The alignment of single character may produce difficulty to detect in an image and video. If there is no spacing between two or more character or say they are connected character, it is hard to detect text from image and video frames. Hence we can say that the alignment may occur because of Single character alignment and Text objects with connected character. |
Inter character distance: - If the distance between character is zero or if they are overlapping to each other or same character is repeating in an image and video frames, then it creates a problem for detecting text in an image and videos. The image or video having inter character distance variation may be Repeating character. |
ii) Color of text: |
The problem occurred for detecting text form an image and video due to color, brightness, complex background and transparent character of text in image and video frames gives rises to variation that affect text detection. The image or video having color variation may be Transparent character, Different color and brightness and Complex background |
iii) Motion of text object: |
In low quality videos and low pixel images, the problem occurred due to motion of image, low quality image and flexible surface of image, it is difficult to detect text object from image and videos. The image or video having motion variation may be Fast moving images, Blurred Images and Flexible surface |
iv) Edge of text: |
The text extraction algorithm is based on the fact that text consists of strokes with high contrast. Thus variation in contrast at the edge of text give rises to the problem for detecting. The detection in image and video is done using edge of character. |
B) Different methods for text detection |
As there are so many different methods are proposed for text detection in images and videos based on variations of text. Some of methods like detecting text from corner of character, character energy and link energies, edge of character, segmentation and spatial grouping, stroke width transform and so on. Here we have discussed two methods: Corner based and Character and link energy based |
1) Corner based method |
This method is proposed by Xu Zhao et al [2]. In this method, a corner based approach to detect text and caption from videos. This approach is inspired by the observation that there exist dense and orderly presences of corner points in characters, especially in text and caption. We use several discriminative features to describe the text regions formed by the corner points. The usage of these features is in a flexible manner, thus, can be adapted to different applications. Language independence is an important advantage of the proposed method. |
In this method, corner point is important feature which have following advantages. This are 1) Corners are continuous and essential patterns in text regions. Corner is more stable and robust than other low level features; hence the impacts of background noises can be eliminated to a large extent. 2) The distributions of corner points in text regions are usually more orderly in comparison to the non-text regions. Therefore, the unordered non-text corner points can be filtered out according to designed features. 3) The usage of corner points generates more flexible and efficient criteria, under which the margin between text and non-text regions in the feature space is discriminative. |
a) Corner points extraction |
A corner can be defined as the intersection of two edges or a point where there are two dominant and different edge directions in a local neighbourhood of the point. In this method, Harris Corner detector is used to extract the corner points. The corner points are easily recognized by looking at intensity values within a small window. Shifting the window in any direction should yield a large change in appearance. In this, rectangle window or Gaussian window is used. Harris corner gives mathematical approach for determining the region whether it is flat, edge or corner region. |
b) Feature description |
After extracting the corner point, compute shape properties of the region containing corner points, to make the decision to accept the region as text or not. Firstly, we do Image morphology dilation on the binary corner image. In doing so, the separate corner points that are close to each other can be merged into a whole region. In the text and captions, the presences of corner points are dense because characters do not appear alone but together with other characters and usually regularly placed in a horizontal string. Therefore, the text can be effectively detected by figuring out the shape properties of the formed regions. There are following five region properties as the features to describe text regions: area, saturation, orientation, aspect ratio and position. |
Area (Ra): The area of a region is defined as the number of foreground pixels in the region enclosed by a rectangle bounding box. Area is the basic feature for text detection. The small regions generated by the disorderly corner points can be easily filtered out according to the area measurement. |
Saturation (Rs): The saturation specifies the proportion of the foreground pixels in the bounding box that also belong to the region, which can be calculated by Rs = Ra/RB, where RB represents the whole region enclosed by the bounding box. This feature is very important for the cases where the non-text corner points can also generate the regions with relative large Ra values. Thus we can filter out the regions as there are fewer pixels than the non-text region. |
Orientation (Ro): Orientation is defined as the angle (ranging from -90º to 90º) between the x-axis and the major axis of the ellipse that has the same second-moments as the region. This feature is useful where the non-text regions have relative large Ra and small Rs. |
Aspect Ratio (Ras): Aspect Ratio of a bounding box is defined as the ratio of its width to its height. In videos, text and captions usually are placed regularly along the horizontal axis. Therefore, a relative large value indicates more the presence of captions than a small Ras value. We can utilize this characteristic to filter out some of the false alarms. |
Position (Rp): The position of a region with its centroid. The position information can be used to locate the text regions with specific type and style. |
Combine the previously mentioned five features to detect text and captions and filter out the false alarms. Advantage of corner based method is that it is Language independent. The average time spent on each pixel is low as compared with texture based approaches. The missing detection is mainly caused by Blur and low contrast quality and connected component (text and non-text region). |
2) Method based on character energy and link energy: |
As, C(x,y,t,λ) represent the spatial energy distribution of an image. Its specifies that Energy at spatial co-ordinates(x,y) at time t, and wavelength λ. As intensity is real value and it is directly proportional to modulus square of electricity. Thus we can use character and link energy to detect text from Image and video. |
This method is proposed by Jing Zhang et al [4]. In this method three character features are used to detect text objects comprising two or more isolated characters in images and videos. Each character is a part in the model and every two neighbouring characters are connected by a link. For every candidate part, compute character energy based on that each character stroke forms two edges with high similarities in length, curvature, and orientation. For every candidate link, we compute link energy based on the similarities in color, size, stroke width, and spacing between characters that are aligned along a particular direction. For every candidate text unit, combine character and link energies to compute text unit energy which measures the likelihood that the candidate is a text object. This method can capture the inherent properties of characters and discriminate text from other objects effectively. First, character energy is computed based on the similarity of stroke edges to detect candidate character regions, then link energy is calculated based on the spatial relationship and similarity between neighbouring candidate character regions to group characters and eliminate false positives. In a text, each character is a part. Two neighbouring parts are connected by link and form a text unit. Then compute character energy so that we can indicate the probability that a candidate text model is character. |
a) Initialize candidate text object |
The initialization of a candidate text object is based on assumption that the boundary of a character is closed in the image because typically the character has relatively big contrast to its background. Localize the candidate parts by extracting closed boundaries in the edge map generated by a zero-crossing based edge detector. The edge appears as a pairs. Calculate gradient vector and find the corresponding point for every boundary point. |
b) Character feature |
There are mainly there features for calculating the character and link energy. These are |
(i) Average angle difference of corresponding pairs (Dangle) Measures the average gradient direction difference of all corresponding pairs of a candidate part |
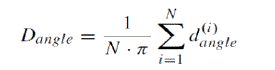 |
And, difference of the gradient direction is  |
Where, N = number of edge points of candidate part, abs() = absolute value and θp(i) and θpcorr(i) are the gradient direction of P(i) and Pcorr(i) |
(ii) Fraction of Non-Noise Pairs (Fnon-noise) |
It measures the noise. Fnon-noise is the fraction of all pairs for which the angle difference is greater than β and calculated as |
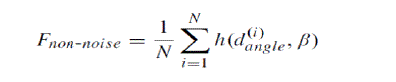 |
Where, h(d (i)angle, β) = 1 if d (i)angle less than β, else 0, N = number of edge points of candidate part and β = pre-defined angle. |
(iii) Vector of Stroke Width (Vwidth) |
This feature is used to calculate link energy. Characters have one or more dominating stroke width depending upon their fonts. Estimate two dominating stroke width for each candidate part using stroke width connections. Let Hsw be the histogram of the lengths of stroke width connection (Euclidean distance measured in pixel width units rounded to nearest integer value). To estimate dominating stroke width values Wd(i) (i ? [1,2]) through a weighted average computation using Wp(i) (i ? [1,2]). |
 |
where, Wp(i) is peak value of Hsw and two weights r1 = HSW(Wp(i) - 1)/HSW(Wp(i) ) and r2 = HSW((Wp(i) + 1)/HSW(Wp(i) ). Hence the vector stroke width Vwidth is defined as |
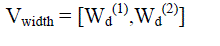 |
c) Compute Character Energy (EChar(i)) |
It is calculated by, |
 |
The value of character energy lies between 0 to 1. It measures the probability that candidate is a character. Hence, for a character, Echar is larger than non-character energy. Hence character energy can differentiate between character and non-character objects. |
d) Compute Link Energy (ELink(i,j)) |
A text object contains more than one character. Therefore, the relationships between two neighbouring characters can also provide important information for text detection. Compute link energy for every candidate link to measure the probability that two parts connected by the link are both characters. Link energy is computed by measuring two values: (i) Similarity in the properties of neighbouring parts, such as the color, stroke width, and size. (ii) Spatial consistency in the direction and distance between neighbouring parts in a string of parts. The link energy between two characters vi and vj can be defined as |
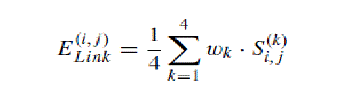 |
where, wk are non-negative weights summing to 1. We set to 0.25 because we want to give equal weight to every similarity, Si,j(k) (k=1,2,3,4) are values of similarity and can be calculated as |
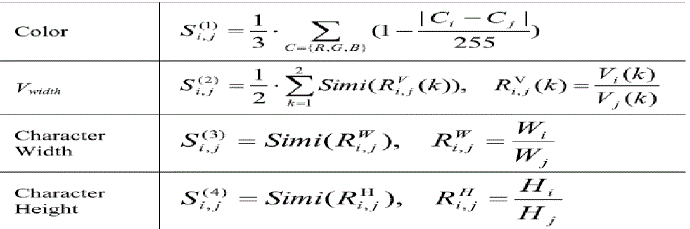 |
where, Simi(R) = min(R,1/R), Ci and Cj are the means of RBG channels of two characters vi and vj. Vi(k), Vj(k) = stroke width, Wi ,Wj = character width, Hi , Hj = character height. |
 |
The higher intensity of a link indicates the higher text unit energy. As the characters have high intensity as compared with non-characters, thus they have high text unit energy as compared to non-character. To detect text objects, text units whose text unit energies are smaller than a pre-defined threshold TText are removed from the text objects. Thus a text is detected from an image. |
Advantage of this method is that it can detect the text with various fonts, sizes, colors and orientations. But still it has some limitations. It cannot detect single character as it required two or more character for finding link energy. This method cannot solve the problem of repeating patterns, small characters and transparent characters. |
CONCLUSION
|
Detection of text in images and videos are vast topics to discuss. We have reviewed different types of variation in text of images and videos. We have also reviewed two methods for Text Detection such as detection from Corner and Character and Link energy. We have gone through various Digital Imaging tools such Matlab and Simulink. |
ACKNOWLEDGEMENT
|
I would like to present my honest gratitude to Prof. N.N. Khalsa and Prof. S.G. Kavitkar for their immense support and guidance throughout the work. |
References
|
- K. Jung, K. I. Kim, and A. K. Jain, “Text information extraction in images and video: A survey,” Pattern Recognit., vol. 37, no. 5, pp. 977–997, 2004.
- X. Zhao, K.-H. Lin, Y. Fu, Y. Hu, Y. Liu, and T. S. Huang, “Text from corners: A novel approach to detect text and caption in videos,” IEEE Trans. Image Process., vol. 20, no. 3, pp. 790–799, Mar. 2011.
- W. Kim and C. Kim, “A new approach for overlay text detection and extraction from complex video scene,” IEEE Trans. Image Process., vol. 18, no. 2, pp. 401–411, Feb. 2009.
- Jing Zhang and R. Kasturi, “A noval text detection system based on character and link energies” in IEEE Trans. Image Processing, Vol. 23, No. 9, Sep 2014.
- Y.-F. Pan, X. Huo, and C.-L. Liu, “A hybrid approach to detect and localize texts in natural scene images,” IEEE Trans. Image Process., vol. 20, no. 3, pp. 800–813, Mar. 2010.
- Z. Tu, X. Chen, A. L. Yuille, and S.-C. Zhu, “Image parsing: Unifying segmentation, detection, and recognition,” Int. J. Comput. Vis., vol. 63, no. 2, pp. 113– 140, 2005.
- C. Yi and Y. Tian, “Text string detection from natural scenes by structure-based partition and grouping,” IEEE Trans. Image Process., vol. 20, no. 9, pp. 2594–2605, Sep. 2011.
- K. I. Kim, K. Jung, and J. H. Kim, “Texture-based approach for text detection in images using support vector machine and continuously adaptive mean shift algorithm,” IEEE Trans. Pattern Anal. Mach. Intell., vol. 25, no. 12, pp. 1631–1638, Dec. 2003.
- X. Chen and A. L. Yuille, “Detecting and reading text in natural scenes,” in Proc. IEEE Comput. Soc. Conf. Comput. Vis. Pattern Recognit., vol. 2. Jun./Jul. 2004, pp. II-366–II-373.
- P. Shivakumara, T. Q. Phan, and C. L. Tan, “A Laplacian approach to multi-oriented text detection in video,” IEEE Trans. Pattern Anal. Mach. Intell., vol. 33, no. 2, pp. 412–419, Feb. 2011.
- B. Epshtein, E. Ofek, and Y. Wexler, “Detecting text in natural scenes with stroke width transform,” in Proc. IEEE Conf. Comput. Vis. Pattern Recognit., Jun. 2010, pp. 2963–2970.
- D. Chen, J.-M. Odobez, and H. Bourlard, “Text detection and recognition in images and video frames,” Pattern Recognit., vol. 37, no. 3, pp. 595–608, 2004.
- J. Zhang and R. Kasturi, “Extraction of text objects in video documents: Recent progress,” in Proc. 8th IAPR Int. Workshop Document Anal. Syst., Sep. 2008, pp. 5–17.
- H. Tran, A. lux, T. H. L. Nguyen, and A. Boucher, “A novel approach for text detection in images using structural features,” in Proc. 3rd Int. Conf. Adv. Pattern Recognit., 2005, pp. 627–635.
- J. Zhang and R. Kasturi, “Text detection using edge gradient and graph spectrum,” in Proc. 20th Int. Conf. Pattern Recognit., Aug. 2010, pp. 3979–3982.
- P. F. Felzenszwalb and D. P. Huttenlocher, “Pictorial structures for object recognition,” Int. J. Comput. Vis., vol. 61, no. 1, pp. 55–79, 2005.
|