Keywords
|
Premature ventricular contractions (PVCs), Electrocardiogram (ECG). |
INTRODUCTION
|
According to the recent research, 57 millions Americans and 6 millions Canadians have suffering from cardiovascular disease. Heart disease is a leading cause of death in these two and many other countries. Monitoring heart rhythms for the detection of early Problems and benign situations has been an important task of cardiologists and related medical staff or member. Premature ventricular contractions (PVCs) are ectopic heart beats originating from ventricular area. It is common form of heart arrhythmia in adults. It occurs with the combination of other problems such as myocardial infarction. Its assessment and treatment are complex. Electrocardiogram (ECG) has been widely used to diagnose PVC [1]. |
The wave shapes of PVCs are usually very different from that of the normal beats of the same subject due to the different conduction paths of the ectopic impulses. The occurrence of PVCs is indication of increased risk of sudden cardiac death, and is linked to mortality when associated with myocardial infarction [2]. |
Generally this arrhythmia may occurs in a healthy person, but mostly on elderly patients ( males: 60-80%) and also occurs on patients those suffering from cardiac diseases such as myocardial infarction, hypertension etc. A typical ECG wave of a healthy person heart rate consists of a P, QRS and T wave. A small U wave is normally visible in 50- 75% of ECG. |
I (A) ECG signal
|
Electrocardiogram (ECG) is the mostly used biomedical signal which is used to detect the several cardiac disease and abnormalities, figure 1 shows that how the ECG signal generate through the heart actvity. Electrical impulse generated by the polarization and depolarization of cardiac tissue and convert into a waveform. As we know that ECG signal is a non- stationary in nature hence their some difficulties occurs on visual analysis of ECG signal. Thus there is a need of computer based analysis of ECG signal. The electrical event of heart can be detected by the electrocardiogram which allows the PVC to be easily differentiated from normal heart beat. |
I (B) About PVC beat
|
A Premature ventricular contraction (PVCs) is a ventricular originated early heart beat that disrupt the normal rhythm of hearts fig. 2 shows the occurrence of PVC beat on the ECG signal. Arrows on the fig. 2 showing the PVC beat have large width and amplitude. It is a common event where the beat is initiated by purkinje fibres in the ventricles while in normal heart beat initiator the sinoatrial node. A PVC is a sign of decreased oxygenation to the myocardium but is also found in healthy heart. When we feel a skipped beat or palpitation in the chest then it may be a PVC. In a normal heart atria contract first and then ventricular which helped them to filling the blood. Thus the ventricles can pump a maximum amount of blood to the both lungs and to the rest of the body but in a PVC ventricles contract before the atria which optimally filled the ventricles with the blood therefore the circulation is not sufficient. |
LITERATURE REVIEW
|
Premature Ventricular Contraction (PVC) is also known as Ventricular Premature Beat (VPB). For an abnormal heart condition or arrhythmia extrasystole is caused due to which early contraction of ventricles occurs. ECG signal is very important for the detection of PVC. In the following proposed work we study and survey about the detection of PVC or different types of arrhythmia and their classification. |
Jie Zhou (2003), proposed the Automatic detection of PVC by using Artificial Neural Network i.e. Quantum Neural Network ( QNN). The fuzzy feature is used to enhance the reliability of the process and the result is based on the MITBIH Arrhythmia Database. The constantly good reliability from quantum neural network provides desirable diagnosis accuracy [1]. |
Saurabh Pal et al. (2010), proposed a data based approach where the Form Factor (FF) and R peak amplitude are calculated in term of wave morphology and the classification of PVC beats from normal beat have done using Artificial Neural Network (ANN). They obtained the sensitivity (Se) 94.11%, specificity(Sp) 97.5% , and accuracy of detection 97.5% [2]. |
Philip de Chazal et al. (2004) classified five different beat normal beats, ventricular ectopic beats (VEBs), supraventricular ectopic beats (SVEBs), fusion of normal and unknown beats type. They determined the fiducial point manually. They used the feature unscaled ECG morphology, R-R interval and heart beat segmentation information for the determination of diagnosis. For the SVEB and VEB sensitivity 75.9% and 77.7% , positive predictivity 38.5% and 81.9% and the false positive rate (FPR) 4.7% and 1.2% were respectively obtained [3]. |
Yasmine Benchab et al. (2009) used the artificial neural network (ANN) for the classification of heart beat of ECG signal. They distinguish between normal, PVC, right bundle branch block (RBBB) and left bundle branch block (LBBB) of ECG signal. The sensitivity (Se) and correct classification (CC) of PVC, RBBB and LBBB were Se = 93.5185%, 100%, 100% and CC = 96.1956%, 100%, 95.12% respectively [4]. |
G.M.A. Ehsanur Rahman et al. (2012) used the blood pressure (BP) for detection of PVC. Here PVC is an indication of abnormalities of heat which means heart does not work properly. They use MATLAB for their work [5]. |
Turker Ince et al. (2008) proposed an automated patient-specific electrocardiogram (ECG) beat classifier which designed for accurate detection of premature ventricular contraction (PVC). They used future extraction scheme in which the principle component analysis (PCA) is applied to the dynamic wavelet transform (DWT) and the feed forward ANN’s for the classification of ECG. Here Multi-dimensional particle swarm optimization (MD-PSO) algorithm used with ANN [6]. |
Omer T. Inan et al. (2006) proposed the classification of ECG beat for a large set of data by combining wavelet transform and timing information with the neural network classifier. To differentiate the normal and PVC beat they calculate the ratio of wavelet transform and pre/post R-R interval. An accuracy of detection was 95.16% over 40 files from the MIT- BIH arrhythmia database [7]. |
Rameshwari S Mane et al. (2013) proposed an efficient and simple detection algorithm based on feature extraction of ECG signal. Here the two parameters sensitivity and specificity are calculated to evaluate the detection performance. They classified three arrhythmia PVC, LBBB and RBBB [8]. |
Wei Jiang et al. (2007) used the evolutionary optimization of the feed forward implementation of Block based Neural Network (BbNN) and the application of BbNN approach for the classification of ECG. Here they used the MIT-BIH Arrhythmia Database record to detect the VEB and SVEB signal. A sensitivity and accuracy for VEB detection were 86.60% and 98.1% and for SVEB detection 50.6% and 96.6% respectively [9]. |
Kei-ichiro Minami et al. (1999) proposed a detection of supraventricular rhythm (SVR), ventricular rhythm (VR) and ventricular fibrillation (VF) signal by using a hardware implementation of the algorithm on a commercial single chip 8- bit CPU. Here ECG signals are given as input to the amplifier for amplification then after to the 8-bit CPU or hardware for processing and then after to the indicator which differentiate the VF, VR and SVR through the red, yellow and green light respectively [10]. |
Enseih Sadat Hosseini Rooteh et al. (2011) proposed the classification of heart arrhythmia on PVC, LBBB and RBBB by using Wavelet Transform and Neural Network. Signals are recorded from the two leads ML2 and V1. Wavelet transform is used for feature extraction and then feature vectors are classified on different methods by using neural network [11]. |
Durgesh Kumar Ojha et al. (2014) proposed the detection of P, Q, R and S wave of ECG signal using MATLAB, in which detection algorithm were implemented as mathematical signal processing operation and stastical analysis of result [12]. |
A.Quelli et al. (2012) proposed the three stage technique for classification of six type of cardiac arrhythmia i.e. Normal Sinus Rhythm (NSR), PVC, Atrial Premature contraction (APC), VF, ventricular tachycardia (VT) and supraventricular tachycardia (SVT). Three stage techniques include the use of FIR least square filter for smoothing, Autoregressive (AR) modelling for feature extraction and then QDF (Quadratic Discriminant function) based algorithm for classification [13]. |
CONCLUSION
|
This paper performed a systematic study of the classification of ECG signal and detection of different types of arrhythmia based on ECG beat that’s found on human being. Various authors worked on detection of different types of arrhythmia like PVC, LBBB, RBBB, VEB, SVEB, SVR, VR, VF, APC, NSR, VT and SVT. Mostly they used the Artificial Neural Network (ANN) and MATLAB. Most of the reviewed paper used the detection of arrhythmia i.e. PVC beat of ECG signal and give the good accuracy of detection and sensitivity. So from above review we can conclude that a better detection method should be developed which provides greater accuracy and sensitivity for detection of PVC and any other arrhythmia. |
Figures at a glance
|
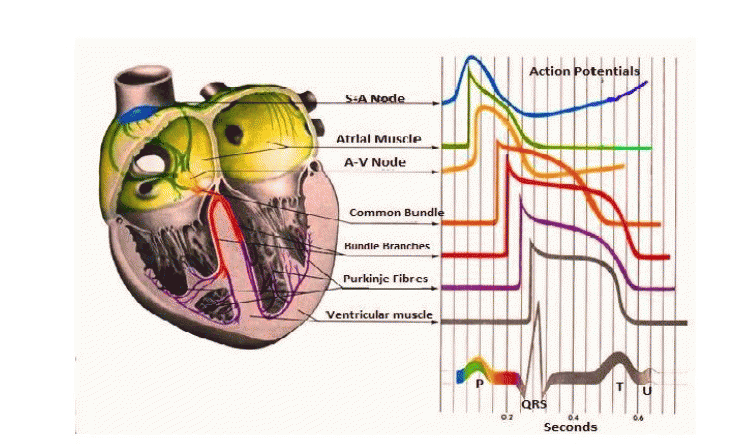 |
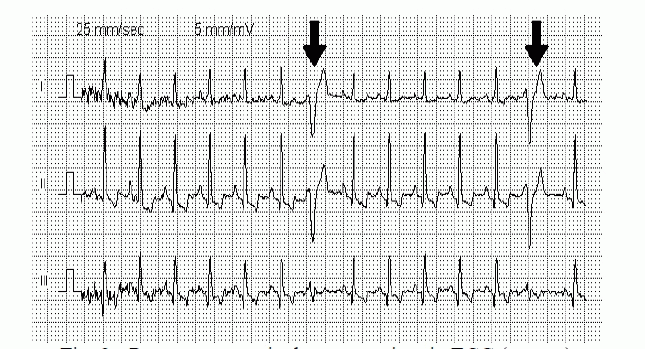 |
Figure 1 |
Figure 2 |
|
|
References
|
- Jie Zhou, “Automatic Detection of Premature Ventricular Contraction Using Quantum Neural Networks,” Third IEEE Symposium onBioinformatics And Bioengineering, pp. 169-173, 2003.
- Saurabh Pal, “Detection of Premature Ventricular Contraction Beats Using ANN,” International Journal of Recent Trends in Engineering andTechnology, vol. 3, no. 4, May2010.
- Philip de Chazal, “Automatic Classification of Heartbeats Using ECG Morphology and Heartbeat Interval Features,” IEEE Transaction onBiomedical engineering , vol. 51, no.7, pp. 1196-1206, July 2004.
- 4.YasmineBenchaib , Ma.Chikh “ A specialized Learning for Neural Classification of Cardiac Arrhythmias,” Journal of Theoretical & AppliedInformation Technology, vol. 6, no. 1, pp 092-100, 2009.
- G.M.A. EhsanurRahman, NahidHasan an, M Saddam Hossain Khan, “Using Blood Pressure Data to Detect Premature Ventricular ContractionBeat,” International Journal of Engineering & Technology, vol. 12, no. 06, December 1012.
- TurkerInce, SerkanKiranyaz, Manoj Gabbouj, “Automated patient- specific classification of premature ventricular contraction,” Engineering inMedical and Biology Society, 30th Annual International IEEE Confrence, pp. 5474-5477, 2008.
- Omer T. Inan, Laurent Giovangrandi and Gregory, “Robust Neural-Network-Based Classification of Premature Ventricular Contractions UsingWavelet Transform and Timing Interval Features,” IEEE Transaction on Biomedical Engineering, vol. 53, no. 12, pp. 2507-2515, December 2006.
- Rameshwari S Mane, A N Cheeran ,Vaibhav D Awandekar, Pritya Rani, “Cardiac Arrhythmia Detection By ECG Feature Extraction,” International Journal of Engineering Research and Applications, vol. 3, pp. 327-332, March-April 2013.
- Wei Jiang, Seong G. Kong, “Block-Based Neural Network for Personalized ECG Signal Classification,” IEEE Transaction on Neural Network,vol. 18, no. 6, pp. 1750-1761, November 2007.
- Kei-ichiro Minami, Hiroshi Nakajima, Takeshi Toyoshima, “Real -Time Discrimination of Ventricular Tachyarrhythmia with Fourier-TransformNeural Network,” IEEE Trasanction on Biomedical Engineering, vol. 46, no. 2, pp. 179-185, February 1999.
- Enseih Sadat HosseiniRooteh, Youmin Zhang, Zhigang Tian, “Comparision of Parallel and Single Neural Network in Heart Arrhythmia Detection by Using ECG Signal Analysis,” Annual Confrence of the Prognostics and Health Management Society, 2011.
- Durgesh Kumar Ojha, Monica Subashini, “Analysis of Electrocardiogram(ECG) Signal for the Detection of Abnormalities Using MATLAB”World Academy of Science, Engineering and technology, International Journal of Medical Health, Pharmaceutical and Biomedical Engineering, vol.8, no. 2, 2014.
- A.Quelli, B.Elhadadi, H.Aissaoui, B.Bouikhalene, “AR Modeling for Automatic Cardiac Arrhythmia Diagnosis using QDF Based Algorithm,”International Journal of Advanced Research in Computer Science and Software Engineering, vol. 2, no. 5, May 2012.
|