Keywords
|
Video image, Flame detection methods, Smoke detection methods and algorithms. |
INTRODUCTION
|
Fire detection is very crucial for protection of environment and safety of people. The detection of fire and smoke in video is particularly applicable in industrial monitoring and surveillance used to monitor buildings and environment as a part of an early warning mechanism that reports preferably start of fire. Video-based systems can detect uncontrolled fires at an early stage before they turn into disaster. The advantage of fire detection technology based on video are: 1] detection techniques are very intuitive. 2] Fire detection with high real-time has no delay caused by induction time as transmission and induction is higher than smoke and temperature due to speed of light. 3] Remote surveillance cameras can be adjusted freely, therefore detection range is larger. 3] Technology has higher reliability and real-time performance. Video fire detection has become has become hot topic for the researcher in the field of fire detection. Detection methods based on image information are more effective than other techniques which use sensor or multisensory wireless network systems. |
Fire detection can improve the accuracy of the fire alarm, real-time and the robustness. Fire detection technology can be divided into different sub-categories based on: 1] The purpose flame or smoke detection, 2] The spectral range of the camera used, 3] The range of the system. Smoke and fire detection is challenging mainly in open environment such as power plants, chemical plants as they affect the surrounding area. Also video surveillance used in military fields and commercial applications. Smoke is the good indicator of fire. For early fire detection system characteristics of smoke need to take into consideration while dealing with image information. Response to environment conditions, unsteady nature of smoke, shape and semi-transparency are the different characteristics of the smoke. Texture, rigid motion, color, shape are the features of fire. If the optimal algorithms can be adopted for each part of detecting motion area and extracting fire characteristics, the system performance can be improved. |
LITERATURE SURVEY
|
As discussed detection of fire is very crucial parameter in many fields of industrial area, forest area, etc. After many researches and study going on the flame detection and smoke detection, some algorithms are specially designed for this purpose. These algorithms designed till now on fire detection through videos are, statistical color model, spatio- Temporal Flame Modelling and Dynamic Texture Analysis and now a day’s optical mass flow estimators is getting attention. The Scientists Kosmas Dimitropoulos, Panagiotis Barmpoutis and Nikos Grammalid are focuses on different modelling algorithms used in flame detection. Also their advantages and disadvantages with respect to application over each other. |
The chart shown in fig. 1 is used to describe scope for fire detection. There are different algorithms which are studied my many researchers for fire and smoke detection in video. This paper describes previously used flame detection techniques and smoke detection techniques with the review. |
FIRE DETECTION TECHNIQUES
|
I. Bosh et al. [1] proposed a fire detection method based on multisensor wireless network. This method consists of wireless sensor network with central monitoring station. Each sensor has two camera’s (visible and thermal) and a motor with different settings to sweep and operate a larger area; and an integrated system of capture, processing and communication. This network allows remote monitoring of each location as well as communication between each sensor and control station. In result, it increases coverage area with faster and safer response. |
The sensor scheme allows automatic monitoring of coverage area as well as processing, generation and transmission of alarms to other elements in the wireless sensor network and to the control station. With a geographic information system (GIS), station can monitor the proper operation of the system and locate the position of each sensor. This paper gives the description of the sensor and the processing scheme, highlighting the improvements of both. In processing scheme, each infrared image is converted into a pixel matrix. Each pixel is composed of resolution cell corresponding to certain coordinates of rank. Pixel-by-pixel processing is done to generate vectors describing the time history of each resolution cell. The different types of detectors are described with the importance given to the decision fusion rules for the persistence and increase detectors, which can gives short and long-term characteristics expected in the fire. |
T. Celik et al. [2] proposed another system consisting of real-time flame detector which combines statistical color information with foreground information. Visual fire detection is more useful in area where standard conventional fire detectors cannot be used. This algorithm combines the color information of the flame with temporal changes in the video sequences. Background modeling is used where the camera’s position is fixed and picture observed is stationary. Initially images are consists of Red, Green and Blue (RGB) components. The Background information is modeled with the unimodel Gaussian method; then Mean and covariance matrix are extracted from images which contains Luminance, Chromared and Chromablue components. Background modeling is composed of three steps: first, Estimation of model parameters and change map. Second, adaptation of model parameters and third is permanent change in background. |
For color detection, Water Philips et al [3] uses a lookup table for recognition of fire in video sequences using a manual training set. The lookup table is used to identify the possible fire pixels of color. Color lookup algorithm used in this system may be consisting of following steps: 1] Create pairs of training images. 2] Construct the color histogram, and 3] Color transformation. i.e. transformation of RGB to Boolean value which indicates whether an input color is matches with colors predicate. Then Fire detection algorithm in proposed method combines the color detection information with the background subtraction. The first step of algorithm removes the background information and detects foreground motion. The second step is applied if there is detection of fire-like color objects in the foreground pixels. This step is to reject the non-fire objects from the foreground pixels. Third step is applied to remove the acquired noise in change detection map. In forth step, the blobs are detected using connected component labelling (CCL) algorithm [4]. |
T. Celik and Hasan Demirel et al. [5] further enhance system that uses a statistical color model with Fuzzy logic for fire pixel classification. The proposed system develop two models; one based on luminance and second based on chrominance. Fuzzy logic uses the YCbCr color space for the separation of luminance from chrominance instead of using color spaces such as RGB. Existing historic rules are replaced with the Fuzzy logic to make the classification more robust and effective. This model achieves up to 99.00% correct fire detection rate with a 9.50% false alarm rate. |
SMOKE DETECTION TECHNIQUES
|
Smoke is the early sign of most of the fires. Smoke detection is important and necessary for monitoring air pollution; their effects on human health as well as on nature. Therefore it is mandatory to use good smoke detection method. Smoke sensors used for detection has transport delay of smoke from fire to sensor whereas in video smoke detection system, transport delay of smoke would not occur. Traditional smoke detectors do not provide information about location of fire, burning degree and size of the fire. Also they require a close proximity to the smoke. With the use of video smoke detectors, these problems can be overcome; therefore video smoke detection is more effective and it is a good choice for small area as well as large open space such as forest fires and petrochemical refineries. |
Chen-Yu Lee et al. [6] proposed a smoke detection method using a spatial and temporal analysis. The proposed algorithm provides greater flexibility to smoke detection technique and more reliable to work under different conditions. |
Fig. 2 shows the proposed system architecture of the video smoke detection includes four units: 1] Candidate-region extraction, 2] a feature extraction unit, 3] a classification unit and 4] a verification unit. |
Feature extraction can be done by analysing the spatial and temporal characteristics of video sequences for three main features: edge blurring, gradual energy changes and gradual chromatic configuration changes. This proposed algorithm were combined these three features using a temporal-based alarm decision unit (ADU) and a support vector machine (SVM) techniques to obtain more reliable experimental results. Temporal-based alarm decision unit is developed to decrease the false alarm rate and maintain a high detection rate with a short reaction of time. Support Vector Machine (SVM) algorithm is used to combine all the extracted analysed features of fire. The SVM is trained with the classification and testing can be done fast using a C++ program which makes it suitable for many real-time applications. This proposed algorithm can process 30.98 frames per second. |
The experimental results according to scientist Chen-Yu Lee is the proposed algorithm work very well in real environment; still there are some errors in the proposed method, such as continuous adjustment of the exposure value by the camera and the light reflections from the wet ground. |
Pietro Morerio et al. [7] proposed another approach to smoke detection which is based on color features and dynamics analysis. In proposed system includes five main modules: 1] Change detection, 2] Motion detection, 3] Fire features extraction, 4] Smoke feature extraction and 5] Chaotic feature extraction. |
This module gives a well-known subtraction algorithm to obtained pixels which are different than normal pixels of the background picture. A motion detection algorithm is used for the detection of smoke and fire pixels. Pixel selection can be done according to dynamics of the area so as to reduce false detection. Fire and smoke pixels are separated using color information and feature extraction module. Separation of pixels is done with the YCbCr. Then Chaotic analysis is performed. The segmented blobs are wrapped in rectangle to reduce the amount of information and thus optimize elaboration speed. |
After Chaotic analysis, data fusion is done to generate a new pre-alarm. The main function of this module is to reject the false alarms caused due to other fire-like moving objects. It is done by the classifying pre-alarm rectangles with the help of a multi-layered perceptron (MLP). MLP consists of multi-layered network structure divided into input layer, some hidden layers and output layer. The three output neurons are used in this method which represents three possible prealarm states: pre-alarm of smoke type, pre-alarm of fire type and no pre-alarm. |
PROPSED FIRE DETECTION METHOD
|
The proposed system is a new video based fire and smoke detection system that makes use of optical flow features calculated from optical flow vectors created by different optical flow methods for feature vector extraction and then uses trained neural networks for feature vector classification. The main part of this system is the optical flow vector creation that will be used to estimate the amount of motion undergone by an object while moving from one frame to another. |
Fig. 3 shows the block diagram of proposed fire and smoke detection system. The overall system is mainly divided into two parts; one is flame based detection and other one is smoke based detection. |
The proposed system composed of following three steps [8]: |
1] Preprocessing is involved with the color transformation and that converts the input video into sequences of frames which is suitable for processing. |
2] Feature extraction using optical flow estimators i.e. Optical Mass Transport (OMT) and Non-Smooth Data (NSD); which is designed for detection of fire and smoke. From optical mass transport essential pixels features are extracted that are OMT transport energy and OMT sink/source matching. From Non-Smooth Data two essential pixels features are extracted that are NSD flow magnitude and NSD directional variance. |
3] Classification to detect the flame region in the video frame using Supervised machine-learning-based algorithm such as Neural Network. Neural networks are trained on dataset of features and ground truth; Backpropagation neural network is used for training neural network. Classification algorithms use the analysed features as an input and decision at the output whether the target is present or not. |
CONCLUSION
|
The proposed system will give the combine result at the output whether smoke and fire is present or not. The system performance can be improved with the use of optimal algorithms for detecting motion area and extracting features of fire. Neural network based outputs from both flame and smoke modules are combined to get a final output with different states as: both smoke and flame are present, fire with smoke but without flame, fire with flame but without smoke and non-fire i.e. no smoke and no flame. The enhanced system will performed well than the existing system in terms of detection rate. |
Figures at a glance
|
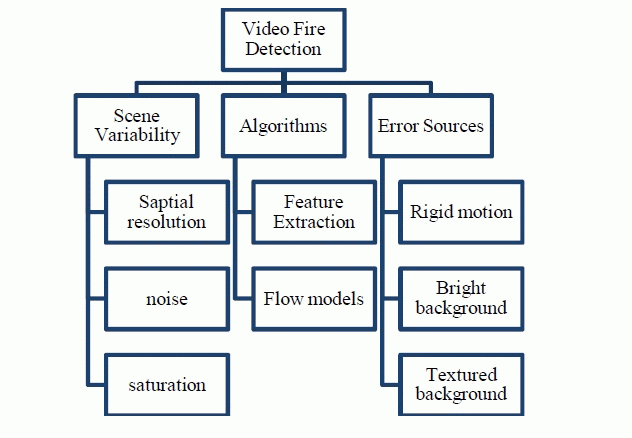 |
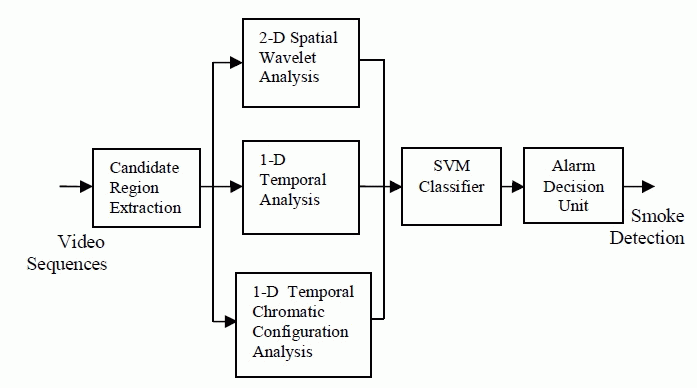 |
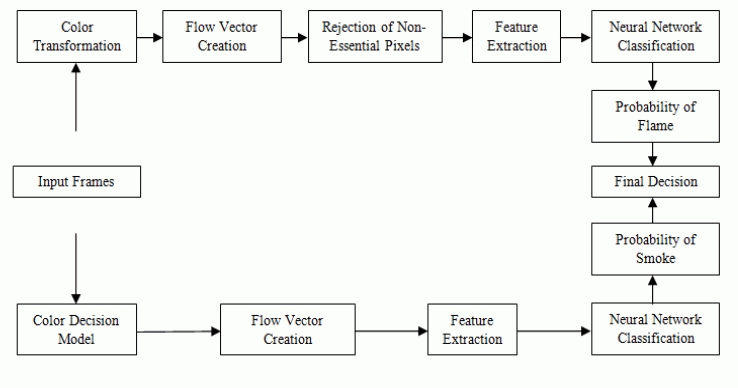 |
Figure 1 |
Figure 2 |
Figure 3 |
|
|
References
|
- I. Bosch, A. Serrano, and L. Vergara, “Multisensor Network System for Wildfire Detection Using Infrared Image Processing”, Hindawi Publishing Corporation, the Scientific World Journal, Volume 2013.
- T. Celik, H. Demirel, H. Ozkaramanli, M. Uyguroglu, “Fire Detection In Video Sequences Using Statistical Color Model,” in Proc. IEEE Int.Conf. Acoust. Speech Signal Process.,vol. 2. Toulouse, France, May 2006.
- W. Phillips III, M. Shah, and N.V Lobo, “Flame recognition in video”, Pattern recognition letters, vol. 23, pp. 319-327,2002.
- Rafael C. Gonzalez, Richard E. Woods, Digital Image Processing, Prentice Hall, 2002.
- TurgayÇelik, HuseyinOzkaramanli, HasanDemirel, “Fire Pixel Classification Using Fuzzy Logic and Statistical Color Model”. ICASSP 2007.
- Chen-Yu Lee, Chin-Teng Lin, Chao-Ting Hong and Miin-Tsair Su, “Smoke Detection Using Spatial and Temporal Analyses”, ICIC International ©2012 ISSN 1349-4198.
- PietroMorerio, LucioMarcenaro, Carlo S. Regazzoni, GIANLUCA Gera, “Early fire and smoke detection based on color features and motionanalysis”, 978-1-4673-2533-2/12/$26.00©2012IEEE.
- Martin Mueller, Peter Karasev, Ivan Kolesov, and Allen Tannenbaum, “Optical Flow Estimation for Flame Detection in Videos”, IEEE Transactions On Image Processing, Vol. 22, No. 7, July 2013.
|