Keywords
|
Genetic algorithms, image processing, image representations, license plate detection, machine vision, road vehicle identification, sorting crossover. |
INTRODUCTION
|
A numerous research has been carried out to overcome many problems faced in license plate detection but there is no general method that can be used for detecting license plates in different places or countries, because of the difference in plate style or design. All the developed techniques can be categorized according to the selected features upon which the detection algorithm was based. Color based systems have been built to detect specific plates having fixed colors. External-shape based techniques were developed to detect the plate based on its rectangular shape. Edge-based techniques were also implemented to detect the plate based on the high density of vertical edges inside it. Researches were based on the intensity distribution in the plate?s area with respect to its neighborhood where the plate is considered as Maximally Stable Extremal Region (MSER). Many researchers have combined different features in their systems. The applied detection algorithms ranged from window-based statistical matching methods to highly intelligent-based techniques that uses neural networks or fuzzy logic. GAs has been used rarely because of their high computational needs. |
Detecting license text and at the same time distinguishing it from similar patterns based on the geometrical relationship between the symbols constituting the license numbers is the selected approach .Consequently, a new technique is introduced in this paper which detects LP symbols without using any information associated with the plate?s outer shape or internal colors to allow for the detection of the license numbers in case of shape or color distortion either physically or due to capturing conditions such as poor lighting, shadows and camera position and orientation. To search for the candidate objects and to allow for tolerance in the localization process, a new genetic algorithm has been designed with a new flexible fitness function. Image processing is carried out at first to prepare for the GA phase. Different researches have been tried at different levels under some constraints to minimize the search space of GAs. Researchers based their GA on pixel color features to segment the image depending on stable colors into plate and non plate regions, followed by shape dependent rules to identify the plate?s area. Success rate o f 92.8% was recorded for 40 test samples. GA was used to search for the best fixed rectangular area having the same texture features as that of the prototype template. |
SYSTEM OVERVIEW
|
In this section, an overview of the system is introduced. The proposed system is composed of two phases: image processing phase and GA phase. Each phase is composed of many stages. GA selects the optimum LP symbol locations depending on the input GRM that defines the geometrical relationships between the symbols in the concerned LP. |
IMAGE PROCESSING PHASE
|
In this phase, an input color image is exposed to a sequence of processes to extract the relevant two dimensional objects that may represent the symbols constituting the LP. These processes that are carried out in different stages, will be presented in the following subsections. |
A. Color to grayscale conversion |
The input image is captured as a color image taking into account further processing of the image to extract other information relevant to the concerned vehicle. Color (RGB) to grayscale (gs) conversion is performed using the standard NTSC method by eliminating the hue and saturation information while retaining the luminance as follows: |
gs=0.299*R+0.587*G+0.114*B |
B. Gray to binary using a dynamic adaptive threshold |
Converting the input image into a binary image is one of the most sensitive stages in localizing LPs due to spatial and temporal variations encountered in the plate itself and the environment around it resulting in several illumination problems. Hence binarization of the image according to a fixed global threshold is not suitable to overcome these problems. In our system, a local adaptive method based on the techniques described in image thresholding has been implemented to determine the threshold at each pixel dynamically depending on the average gray level in the neighborhood of the pixel. A simple yet effective rule has been adopted to differentiate between foreground and background pixels. If the pixel intensity is higher than 90% of the local mean it is assigned to the background; otherwise it is assigned to the foreground. |
C. Morphological operations |
Morphological operations such as dilation and erosion are important processes needed for most pattern recognition systems to eliminate noisy objects and retain only objects expected to represent the targeted patterns. In LP detection, closing operation (dilation followed by erosion) is performed to fill noisy holes inside candidate objects and to connect broken symbols. On the other hand, opening (erosion followed by dilation) is applied to remove objects that are thinner than the LP symbols. In our system, closing is applied to fill spaces that break the bodies of symbols using a 3-pixeldisk element. |
GA PHASE
|
In the following sections, the formulation of the GA phase to resolve the 2D compound object detection problem will be introduced in details, indicating the encoding method, initial population setup, fitness function formulation, selection method, mutation and crossover operator design and parameters setting. |
A. Chromosome encoding |
Encoding of a compound object such as the LP is accomplished based on the constituting objects inside it. Since the next step after plate detection is to recognize the license number, hence the main symbols identifying the plate number should be included as a minimum. Other symbols in the LP can be added to extend the representation for more layout discrimination if needed. An integer encoding scheme has been selected where each gene i is assigned an integer j which represents the index to one of the M objects output from the size filtering stage. The information that will be used for each object j is as follows: |
? The upper left corner coordinates (X, Y) of the rectangle bounding the object. |
? The height (H) and width (W) of the rectangle bounding the object. |
B. Defining the fitness function |
The proposed fitness is selected as the inverse of the calculated objective distance between the prototype chromosome and the current chromosome. Before clarifying how the objective distance is measured, we will show first how the geometric relationships between the objects inside a compound object are represented, followed by a discussion of parameter adaption in case of various LP detection layouts. |
Compound object representation |
For any two objects, we will use two types of geometrical relationships that can be defined as follows: |
1. Position relationship: |
The position relationship will be represented by the relative distances between the bounding boxes of the two objects in the X and Y directions. |
2. Size relationship: |
The size relationship will be represented as the relative differences in their bounding boxes? heights and widths. |
C. The selection method |
In our system, the Stochastic Universal Sampling (SUS) method has been adopted for the selection of offspring in the new generation. In SUS method , each individual is mapped to a continuous segment of a line equal in size to its fitness as in roulette-wheel selection. Then, a number of equally spaced pointers are placed over the line depending on the percentage of individuals to be selected. |
D. Mutation operators |
Mutation is needed because successive removal of less fit members in genetic iterations may eliminate some aspects of genetic material forever. By performing mutation in the chromosomes, GAs ensure that new parts of the search space are reached to maintain the mating pool variety. We have implemented two types of interchangeably used mutation operators; substitution operator and swap operator as follows: |
Substitution operator |
In this type of operators, a random position in the chromosome is selected and the corresponding allele is changed by a new random object from the M available objects. The new object should be legitimate which means it does not belong to the current mutated chromosome. |
Swap operator |
In this operator, we implemented the reciprocal exchange mutation that selects two genes randomly and swaps them. This operator has the advantage of rearrangement of the mutated chromosome in a way that may improve its fitness by reordering of the internal objects to match the prototype?s order. |
E. Crossover operator |
There are many methods to implement the crossover operator. For instance, single point crossover, two point crossover, n-point crossover, uniform crossover, three parent crossover and, alternating crossover, etc. These operators are not suitable for our problem because the resultant children will not be valid because of repeated genes that may be produced in the generated chromosomes. Also, if we prevent repetition, the resultant children„s fitness will be enhanced slowly because of the randomness of these mechanisms. An alternative solution is to design a suitable crossover operator that insures enhancement of the generated offspring. Since, in case of LP detection problem, GA is used to search for a sequence of objects having nearly the same y-position and placed in order according to their x-positions, then the problem can be gradually solved by dividing the recombined chromosomes? objects according to their ypositions into two groups and then sorting each group (constituting a chromosome) according to the x-positions. Following the above discussion, we propose a new crossover method that depends mainly on sorting as follows: |
1. The two parent chromosomes are combined into one longer array Carray that includes a number NC of non repeated genes. The underlined gene number indicates its repetition and that only one copy of it will be transferred to Carray. |
2. The genes inside Carray are sorted in ascending order according to the Y-coordinate of the object corresponding to each gene. |
3. Carray is scanned from left to right starting from index 1 to L, to construct the first child giving it the first L genes . |
4. Carray is scanned from left to right starting from index NC-L+1 to NC, to construct the second child giving it the last L genes . |
5. Each child is sorted in ascending order according to the X-coordinate of each gene?s object to produce the final shape of each child. |
F. Replacement strategy |
Many replacement strategies are used in case of replacing only a portion of the population between generations. The most common strategy is to probabilistically replace the less fit individuals in the previous generation. In elitist strategy the best fit individuals of the previous generation are appended to the current population. In our proposed system, the best 10% of the parents are selected and appended to the offspring (90%) to produce the new generation (100%). |
G. Stopping criteria |
The GA stops if one of the following conditions is met: |
1-The best chromosome?s objective distance (OD) is less than 5. (This value is found by trial and error). |
2-The average objective distance (AOD) is not improved for 6 successive generations. In this case, the chromosome having minimum objective distance can be accepted if it is less than 8. This maximum limit will affect the allowable angle range for the detected license numbers. |
CONCLUSION
|
A new genetic based prototype system for localizing 2D compound objects inside plane images has been introduced and tested in the localization of LP symbols. The results were encouraging and a new approach for solving the LP detection problem relying only on the geometrical layout of the LP symbols has been experimentally proved. Also, a flexible system has been introduced that can be simply adapted for any LP layout by constructing its GRM matrix. The system proved to be invariant to object distance (scaling), insensitive with respect to perspective distortion within a reasonable angle interval, and immutable to a large extent to the presence of other types of images in the vehicle background. Due to the independency on color and the adaptive threshold used for binarization, the proposed system possessed high immunity to changes in illumination either temporarily or spatially through the plate area. Furthermore, it is proved that although leaving some features in the compound object representation due to the variable nature of the internal objects such as the aspect ratios and the relative widths, a high percentage success rate was achieved with the aid of the adaptability aspect of the GAs. The ability of the system to differentiate between LP text and normal text has been proved experimentally. A very important achievement is overcoming most of the problems arising in techniques based on CCAT by allowing the GA to skip gradually and randomly one or more symbols to reach to an acceptable value of the objective distance. Moreover, an enhancement in the performance of the developed GA has been achieved by applying the new USPS crossover operators, which greatly improved the convergence speed of the whole system. |
Figures at a glance
|
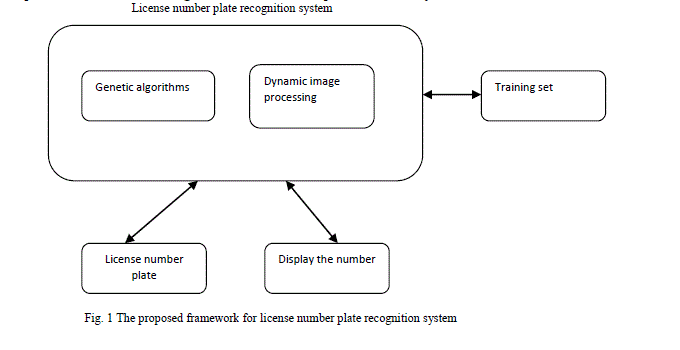 |
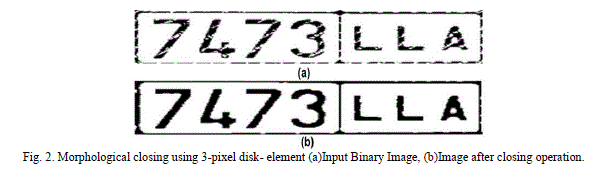 |
Figure 1 |
Figure 2 |
|
References
|
- Y. Qiu, M. Sun, and W. Zhou, “License Plate Extraction Based on Vertical Edge Detection and Mathematical Morphology,” InternationalConference on Computational Intelligence and Software Engineering, pp. 1-5., 11-13 Dec. 2009.
- A. Ahmadyfard and V. Abolghasemi, “ Detecting License Plate Using Texture and Color Information,” IST 2008, InternationalSymposium on Telecommunications, pp. 804–808, 2008.
- G. Li, R. Yuan, Z. Yang, and X. Huang, “A Yellow License Plate Location Method Based on RGB Model of Color Image and Texture ofPlate,” Second Workshop on Digital Media and its Application in Museum & Heritages, pp. 42–46, 2007.
- S.H. Mohades Kasaei, S.M. Mohades Kasaei and S.A. Monadjemi, “A Novel Morphological Method for Detection and Recogn ition ofVehicle License Plate,” American Journal of Applied Science, vol.6 no.12, pp. 2066-2070, 2009.
- Wenjing Jia, Huaifeng Zhang, Xiangjian He and Massimo Piccardi “ Mean Shift for Accurate License Plate Localization” Proceedings of the 8thInternational IEEE Conference on Intelligent Transportation Systems Vienna, Austria, September 13-16, 2005
|