Forecasting of load demand is a very fundamental task and is mandatory in operational planning of power system .At present in the prevailing deregulated scenario load forecasting has become immensely important. Here, in this paper a suitable time series based Auto-Regressive (AR) model of order two has been proposed wherein the influence of temperature has also been included for the purpose of meticulous forecasting. This paper suggests a simple algorithm to forecast short- term load for Assam using the regression based time series method with temperature and then using fuzzy ideology an effort has been made to minimize the error between actual load and predicted load.
Keywords |
Auto-regressive,Deregulated environment,Forecasting, Time series. |
INTRODUCTION |
Theelectric power utilities confront a lot of problems in the economic and technical operation, planning, and control of
a power system in order to fulfill the purpose of supplying quality and reliable electric energy to the consumer in a
secure and economic manner .For optimal planning and operation of power system [1], certain optimization techniques
are applied with the anticipation of reducing the costs and for achieving this objective, the knowledge of future power
system load is the foremost requirement. Demand forecasting [2] is a term used to define the process of predicting the
future load demand beforehand and this is considered as a primary component for energy management system .It
substantially helps the electric utility to take decisions on buying and generating electric power, load switching and
infrastructure development. In the recent years since the last decade with the deregulation of electricity markets the
importance of forecast accuracy of load has become more evident. In absence of an optimal load forecast, the utilities
shall have to face the risk of over- or under- purchasing in the day-ahead market. As electricity demand is closely
influenced by the climatic parameters, there is definitely an impact on demand patterns. The hourly load demand
depends on these vital role parameters and therefore in this paper the effect of temperature on load demand has been
included. |
Load forecasting can be classified into three types: Long-term load forecasting (LTLF)[8] considers peak loads
and consumed energy, on a yearly basis, for many years ahead .As for example, it plays paramount role in the planning
of new electricity utilities and if the load is predicted inaccurately it has to incur important financial cost .It is needed
for expansion, equipment purchases etc. Medium term load forecasting (MTLF) covers a period of one month up to a
few years ahead. It is used for the purpose of scheduling fuel supplies and unit maintenance.Short term load
forecasting (STLF) has a pressing role for electric power system planning. It is used to supply necessary information
for the system management of daily operations and unit commitment. It is quite necessary for power system planners
and demand controllers in ensuring that there would be sufficient generation to meet the required demand. Load serving
entities use forecasts for purpose of system security, to schedule generator maintenance and to plan the most costeffective
dispatch. It leads to prevent equipment failures and black outs. Short Term Load Forecasting (STLF) [8]
refers to forecasts of electricity demand (or load), on an hourly basis, from one to several days or 168 hours ahead.
Here in this paper, load has been forecasted for a period of one week. |
METHODS OF LOAD FORECASTING |
There are numerous load forecasting models of which classical methods and artificial intelligence method are available. |
A. Short-term Load Forecasting Methods |
A diverse number of statistical [1] and artificial intelligence techniques [9] have been developed for short-term load
forecasting. Some of them which finds use in this paper are as follows- |
1) Time Series- Time series forecasting methods[5], [7],[8] have been used for decades in fields such as economics,
digital signal processing, as well as electric load forecasting.Time series method is a basic method to process random
sequence, and is widely applied in STLF for power system. In this method, power load data is viewed as a fluctuant
data sequence with time point, considering the causal relationship between power load and temperature. Auto
regressive[3],[4] model (AR model), moving average model (MA model) and auto regressive & moving average model
(ARMA model) are three main models [3]. ARMA (autoregressive moving average), ARIMA (autoregressive
integrated moving average), ARMAX (autoregressive moving average with exogenous variables), and ARIMAX
(autoregressive integrated moving average with exogenous variables) are the most often used classical time series
methods. ARMA models are usually used for stationary processes while ARIMA is an extension of ARMA to nonstationary
processes. ARMA and ARIMA use the time and load as the only input parameters. Since load generally
depends on the weather and time of the day, ARIMAX is the most natural tool for load forecasting among the classical
time series models. Considering the calculating velocity in STLF, AR(2) model has been selected in this work. |
2) Fuzzy Logic-Fuzzy set theory [10] is one of the most ruling technology in Artificial Intelligence (AI) and it has a
broad spectrum of application in power load forecasting. Fuzzy Systems (FS) offer a very powerful anatomy for
approximate reasoning as it attempts to model the human reasoning process at a cognitive level. FS acquires knowledge
from domain experts and this is encoded within the algorithm in terms of the set of If-Then rules. Fuzzy logic [9], [10]
is a generalization of the usual Boolean logic used for digital circuit design. An input under Boolean logic takes on a
truth value of “0” or “1”. Under fuzzy logic an input has associated with it a certain qualitative ranges which may be
“small”, “medium” and “high”. Fuzzy logic allows one to (logically) deduce outputs from fuzzy inputs. In this sense
fuzzy logic is one of a number of techniques for mapping inputs to outputs (i.e. curve fitting). An important advantage
of fuzzy logic is the absence of a need for a mathematical model mapping inputs to outputs and the absence of a need
for precise inputs. With such simple conditioning rules, properly designed fuzzy logic systems can be very robust when
used for forecasting .After the logical processing of fuzzy inputs, a “defuzzification” process can be used to produce
such precise outputs. Fuzzy logic ,has been therefore used in this paper to improve the result obtained from regression
based time series method. |
B) Temperature Influence on Load |
The weather has an immense influence on electricity consumption. Weather and temperature are important drivers for
electricity consumption. More than 40% of end-use energy consumption is related to the heating and cooling needs in
the residential and commercial sectors. In this section, focus will be given on the effect of temperature although any
other variable can be analyzed in the same way. The power load during the year followed the same the daily and
weekly periods of electric load, which represents the daily and weekly cycles of human activities and behavior patterns,
with some cyclical and random changes. The significance of space cooling on the electric load is very obvious during
the summertime. On the other hand, during the wintertime the reverse relationship between temperature and electric
demand exists because of the need for space heating. |
TIME SERIES MODEL AND FUZZY MODEL |
Time series[5],[6] can be defined as a sequential set of data measured over time, such as the hourly, daily or weekly
peak load. The basic idea of forecasting is to first build a pattern matching available data as accurate as possible, then
obtains the forecasted value with respect to time using established model. Till now, there are many forecasting
methodswhich have been utilized and are classified into two basic types: qualitative and quantitative methods.
Regression analysis, decomposition methods, exponential smoothing, and the Box-Jenkins methodology are some of
the quantitative methods. |
A)AR (2) Model |
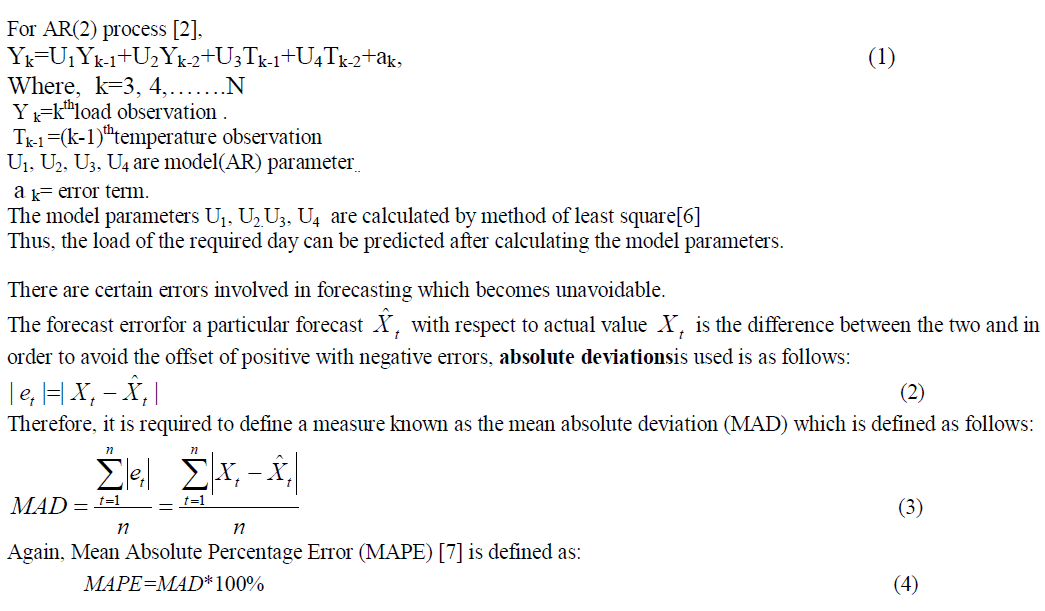 |
Here,MAPE is used to determine the accuracy of load forecasting |
B) Fuzzy Logic System |
In the sense of load forecasting, fuzzy logic [9] does not need precise models relating inputs and outputs and
disturbance. The proper selection of rules and related logic of this method becomes robust when used for forecasting.
Once the fuzzy inputs are logically processed, an inverse process called the "de-fuzzification" can be used to produce
the outputs. Fuzzy logic systems can be applied for SLTF as well as for LTLF. Fuzzy set theory is one of dominant
technology in Artificial Intelligence (AI) and it has a broad application in load forecasting. Fuzzy Systems (FS) offer a
very powerful framework for approximate reasoning as it attempts to model the human reasoning process at a cognitive
level. FS acquires knowledge from domain experts and this is encoded within the algorithm in terms of the set of If-
Then rules. Fuzzy logic is a generalization of the usual Boolean logic used for digital circuit design. An input under
Boolean logic takes on a truth value of “0” or “1”. Under fuzzy logic an input has associated with it a certain qualitative
ranges. For instance a transformer load may be “small”, “medium” and “high”. Fuzzy logic allows one to (logically)
deduce outputs from fuzzy inputs. In this sense fuzzy logic is one of a number of techniques for mapping inputs to
outputs (i.e. curve fitting). Among the advantages of fuzzy logic are the absence of a need for a mathematical model
mapping inputs to outputs and the absence of a need for precise (or even noise free) inputs. With such generic
conditioning rules, properly designed fuzzy logic systems can be very robust when used for forecasting. Of course in
many situations an exact output (e.g. the precise 12PM load) is needed. After the logical processing of fuzzy inputs, a
“defuzzification” process can be used to produce such precise outputs. Fuzzy logic is therefore used in this paper to
improve the result obtained from regression based time series method. |
Fuzzy control is a control method used in the application of fuzzy mathematics theory, which are deterministic,
effective control of some of the mathematical model cannot be constructed process. Fuzzy system regardless of how to
calculate, from input and output angle it is a nonlinear function. Fuzzy control system for an arbitrary nonlinear
continuous function,is to find a class of membership function, a fuzzy inference rules, a solution method, the fuzzy
system designed can approximate any nonlinear function. |
The fuzzy system is a popular computing framework based on the concepts of ‘fuzzy set theory’, ‘fuzzy if then rules’
and ‘fuzzy reasoning’. The structure of fuzzy inference consists of three conceptual components, namely: |
1.Rule Base containing a selection of fuzzy rules. |
2.Database defining the membership functions. These are used in the fuzzy rules. |
3.Reasoning mechanism that performs the inference procedure upon the rules and given facts and derives a reasonable
output or conclusion. |
Sometimes it is necessary to have crisp output. This requires a method called Defuzzification, to extract a crisp value
that best represents the fuzzy output. With such crisp inputs and outputs, a fuzzy expert system implements a non-linear
mapping from the input space to the output space. This mapping is accomplished by a number of if-then rules, each of
which describes a local behavior of the mapping. Fuzzy logic starts with and builds on a set of user-supplied human
language rules. The fuzzy systems convert these rules to their mathematical equivalents. This simplifies the job of the
system designer and the computer, and results in much more accurate representations of the way systems behave in the
real world.Additional benefits of fuzzy logic include its simplicity and its flexibility. Fuzzy logic can handle problems
with imprecise and incomplete data, and it can model nonlinear functions of arbitrary complexity. |
PROPOSED ALGORITHM |
A) Algorithm for the regression based time series model with Fuzzy ideology |
In order to demonstrate the algorithm, load data & data regarding factors influencing load has been collected for a
period of 359 days (from January 01, 2012 to December 24, 2012). One of the main factors which effect the load
consumption of domestic consumers is temperature. The relationship between load and this factor is linear. The
algorithm not only demands the forecast values of load as input data for the fuzzy model but also the errors in the
forecasted values of load. The algorithm for the proposed model can be briefed as follows- |
1.Start |
2.Read load data and temperature data |
3.Initialise Day=1 |
4.Form the AR(2) model including temperature |
5.Determine the forecasted load |
6.Calculate MAPEbetween actual and forecasted load |
7.Apply Fuzzy ideology to minimize error between forecasted load of AR model and actual load |
8.Calculate forecasted load using fuzzy |
9. Calculate new MAPE |
10. Check if day<7,if yes then update Day=Day+1 and repeat from step (4) and if not go to next step |
11.Stop |
B) Fuzzy logic methodology for STLF |
1)Fuzzification |
Fuzzification is the process of converting crisp numerical values into the degrees of membership related to the
corresponding fuzzy sets. A MF will accept as its argument a crisp value and return the degree to which that value
belongs to the fuzzy set the MF represents. In order to express the fuzziness of data, this paper makes an arrangement
of fuzzy subsets for different inputs and outputs in complete universe of discourse as membership functions. The
relationship between several inputs and output may be nonlinear but linear membership functions have been used for
simplicity. A triangular membership function is used for the inputs as well as the output. The two inputs taken for
STLF are actual load and forecasted load. |
2) Fuzzy Rule Base |
Firstly, the historical data are examined and the maximum and the minimum range of different parameters are obtained.
These ranges are used in the process of the fuzzification of different parameters such as actual load and forecasted load
obtained from AR(2) model. After the fuzzification is done, based on the different parameter of load forecasting, rules are prepared. This rules are the heart of the fuzzy system, so utmost care should be taken to prepare these rules. Once,
the rules are prepared obtain the error at the desired hour. The output obtained is compared with the actual load and the
error in load forecasting is used to improve the rule base for future forecast. This improvement in rule of fuzzy logic
increases the accuracy of the load forecasting. The inputs to the fuzzy set based classifier i.e. hourly data of forecasted
load obtained from AR(2) model and actual load are given to the fuzzy inference system through fuzzification block.
The fuzzy inference block is the heart of the system as it processes the input data and gives output as the forecasted
load. The inference system accomplishes the task of forecasting by the used of the fuzzy rule based prepared by the
forecaster. The accuracy of the forecast depends on the experience of the forecaster, the rules prepared by the forecaster
and the number of rules prepared. After, the inference system gives output; the defuzzification block converts the
fuzzified output to the crisp output which can be further displayed on a graph known as the load curve. |
Here, IP1 represents input 1 which is the actual load. |
IP2 represents input 2 which is the forecast load from AR (2) model. |
OPL, OPM, OPH represents the corresponding low, medium and high of the output, Error.
The fuzzy rules for the input and output i.e. the actual load and the forecasted load is fuzzified using the triangular
membership function according to the rules given in Table I to obtain the output according to the rules shown in figure
2 |
3)Defuzzification |
After fuzzy reasoning we have a linguistic output variable which needs to be translated into acrisp value. The objective
is to derive a single crisp numeric value that best represents the inferred fuzzy values of the linguistic output variable.
Defuzzification is such inverse transformation which maps the output from the fuzzy domain back into the crisp
domain. |
Fig 1 shows the basic block diagram of the proposed work. The inputs to the fuzzy set based classifier i.e. hourly data
of actual load and forecasted load from AR (2) are given to the fuzzy inference system through fuzzification block. The
fuzzy inference block is the heart of the system as it processes the input data and gives output as the forecasted load.
The inference system accomplishes the task of improved forecasting by reducing error by the use of the fuzzy rule base
prepared by the forecaster .The accuracy of the forecast depends on the experience of theforecaster, the rules prepared
by the forecaster and the number of rules prepared. After, the inference system gives output; the defuzzification block
converts the fuzzified output to the crisp output .Fig 2 shows thetriangular membership function for two inputs which
are taken as the actual and forecasted load here and erroris considered as output for improved load forecasting.In order
to process the input to get the output reasoning there are six steps involved in the creation of a rule based fuzzy system: |
1. Identify the inputs and their ranges and name them. |
2. Identify the outputs and their ranges and name them. |
3. Create the degree of fuzzy membership function for each input and output. |
4. Construct the rule base that the system will operate under |
5. Decide how the action will be executed by assigning strengths to the rules |
6. Combine the rules and defuzzify the output |
RESULTS AND DISCUSSIONS |
A) Data Set Details |
The data used are fifty one weeks and two days i.e. 359 days hourly load demand measured in Megawatt (MW) from
January 01, 2012 to December 24, 2012. They are gathered from State Load Dispatch Centre (SLDC), Assam, India
.Also .the temperature data in Celsius for the same period as load data are available from the Regional Meteorological
Centre (RMC) located at the Lokpriya Gopinath Bordoloi International Airport, Borjhar, Assam .The method is applied for short term load forecasting [6] using the real time data of State Load Dispatch Centre (SLDC) for the 359
days’ time span in order to forecast the last week of the year 2012 i.e from the period of 24th December to 31st
December,2012 and it is implemented in MATLAB and graphs are plotted with number of samples representing hourly
duration for the whole day in the x axis and corresponding actual load and forecast load on y axis. |
CONCLUSION |
Forecasting [3] load demand with high accuracy is required to avoid energy wasting and prevent system failure. Precise
forecasting of load is very important for electric utilities in a competitive environment created by the electric industry
de-regulation. Here, Fig 3 shows the graph of actual load versus predicted load for 24 hours on 28th December,2012 and
Table II shows the comparison of MAPE without Fuzzy and with Fuzzy for the lastweek of year 2012. |
In this paper, the forecasted load has been obtained by using regression based time series analysis. With inclusion of
temperature the forecasted results of regression model is slightly enhanced.Moreover, the accuracy of prediction has
been improvised by sending the forecasted values obtained from the AR (2) model again to a fuzzy inference system.
Its final forecasting efficiency has been evaluated by computing the mean absolute percentage error (MAPE) between
the actual and predicted values.The forecasting results for the whole week starting from 25th December to 31st
December of the year 2012 has been obtained in a similar manner with minimum MAPE.Here, from25th December,
2012 to 31stDecember,2012 we were able to obtain average MAPE of 4.57% without fuzzy and 2.49 % with fuzzy
which represents a high degree of accuracy. Hence, the Regression based time series model with fuzzy ideology has
been found to have given satisfactory results. |
Tables at a glance |
 |
 |
Table 1 |
Table 2 |
|
|
Figures at a glance |
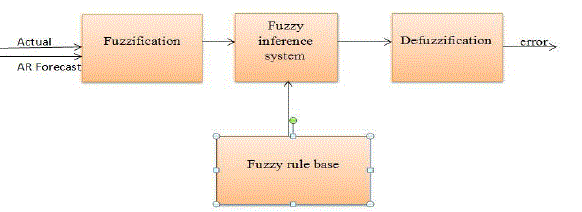 |
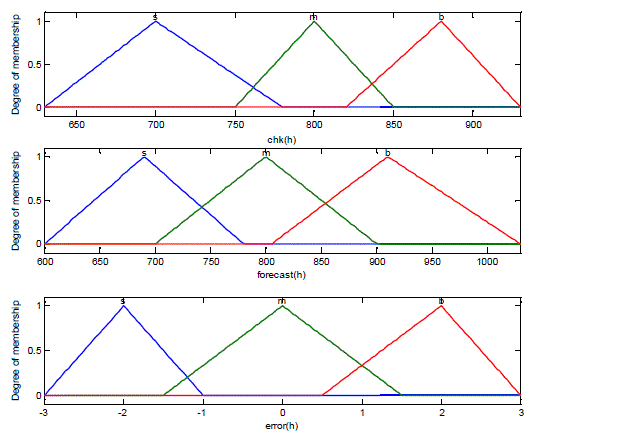 |
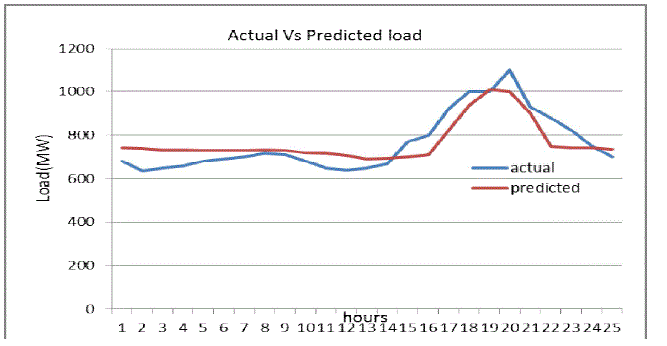 |
Figure 1 |
Figure 2 |
Figure 3 |
|
|
References |
- Moghram and S. Rahman , âÃâ¬ÃÅAnalysis and evaluation of five short term load forecasting techniques,âÃâ¬Ã IEEE Trans. Power System, vol. 4,No 4,pp. 1484âÃâ¬Ãâ1491, Nov. 1989.
- J. P. Rothe, A. K. Wadhwani and S. Wadhwani, âÃâ¬ÃÅShort Term Load Forecasting Using Multi Parameter RegressionâÃâ¬ÃÂ, (IJCSIS) InternationalJournal of Computer Science and Information Security,Vol. 6,No 2, 2009
- D. Papalexopoulos and T. C. Hesterberg, âÃâ¬ÃÅA regression-based approach to short-term load forecasting,âÃâ¬Ã IEEE Trans. Power Syst., vol.5,No 4,pp. 1535âÃâ¬Ãâ1550, Nov. 1990.
- B.L.Bowerman, R.T.O'Connell, and A.B.Koehler, "Forecasting, Time Series, and Regression: An Applied Approach," 4th ed. California:Thomson Brooks/Cole, 2005.
- D.C.Montgomery, L.A.Johnson, J.S.Gardiner, "Forecasting & Time Series Analysis," 2th ed. New York: McGraw-Hill, 1990.IEEETransactions on Power Systems, Vol. 8,No 1, February 1993
- Georgr gross, Francisco D. Galiana , âÃâ¬ÃÅShort-term load forecastingâÃâ¬ÃÂ, Preceeding of the IEEE, vol.75,, December 1987, pp.1558-1573.
- M. T. Hagan and S. M. Behr, âÃâ¬ÃÅThe time series approach to short-term load forecasting,âÃâ¬Ã IEEE Trans. Power Syst., vol. 2, pp.785âÃâ¬Ãâ79, Aug. 1987
- J.Y. Fan and J.D. McDonald, âÃâ¬ÃÅA Real-Time Implementation of Short-Term Load Forecasting for Distribution Power SystemsâÃâ¬Ã IEEETransactions onPower Systems, vol.9, no.2, pp.988-994, 1994.
- M. Chow and H. Tram, "Application of fuzzy logic technology for spatial load forecasting,"IEEE Transactions on Power Systems, vol. 12, pp.1360-1366, 1997.
- Kun-Long Ho, Yuan-Yih Hsu, Chih-Chien Liang and Tsau-Shin Lai, âÃâ¬ÃÅShort-Term Load Forecasting of Taiwan Power System Using AKnowledge-Based Expert SystemsâÃâ¬ÃÂ, IEEE Transactions on Power Systems, vol. 5, Nov. 1990.
|