Communication is a process of transferring the information from one entity to another. Speech is one of the most common modes of communication. The person who suffers from severe motor impairment, severe cerebral palsy, head trauma and spinal injuries cannot able to express their thoughts in the form of speech. The development of direct neurocontrol over virtual or physical devices would improve quality of life immensely for those who suffer from impaired communication skill. In this methodthe development of an Electroencephalogram (EEG) based Brain Computer Interface (BCI) system is proposed. The thoughts of the individual are extracted from the brain activity of a particular disordered patient and it is stored in the form of data.The word frequencies for basic essential requirements such as food, water and assisting work should be generated and the data is stored in matrix representation. Already obtained EEG signal and the word frequencies should be compared and the matched frequencies are stored separately. Finally, the matched frequencies of corresponding words are displayed using LCD display and then the words are converted into audible signal by using speech synthesizer.
Keywords |
Brain Computer Interface(BCI),
Electroencephalogram(EEG), Liquid Crystal Display
(LCD), SpeechSynthesizer. |
INTRODUCTION |
The communication system for person with severe
disabilities helps him to express thoughts for translating
their actions into activity using BCI.BCI have been developed to address this challenge.BCI are systems that
can bypass conventional channels of communication (i.e.
Muscles and speech) to provide direct communication
and control between the human brain and physical
devices by translating different patterns of brain activity
into commands in real time. |
The patients who suffer from severe motor impariments
(like late stage of Amyotrophic Lateral
Sclerosis(ALS),severe cerebral palsy, head trauma and
spinal injuries) cannot express their thoughts as healthy
human beings because they are not capable of talking or
moving. But still they are conscious and capable of
performing mental tasks equivalent to healthy individual
using brain signals. |
EEG-based BCI techniques acquire, process, and then
translate signals from brain activity into machine codesto
providea directcommunication pathway between the
brain and the external world. The acquisition of brain
activity by EEG-based BCIs can be divided into two
categories: invasive and noninvasive .Invasive BCIs use
sensors inside the human brain to obtain high-quality
brain-activity signals or to send external signals into the
brain. Invasive systems provide a reliable manner for
connecting neurons and devices based on appropriate
surgical techniques. Noninvasive EEG-based BCIs,
which measure the electrical activity of the brain using
electrodes placed along the scalp skin, have been shown
to provide a feasible method for communication of the
human brain with external devices. |
The electroencephalogram (EEG) is a recording of the
electrical activity of the brain from the scalp. EEG is
used in the evaluation of brain disorders. Most
commonly it is used to show the type and location of the
activity in the brain during a seizure. It also used to
evaluate people who are having problems associated with
brain function. These problems include confusion, coma,
and tumors, long-term difficulties with thinking or
memory or weakening of specific parts of the body. |
EEG BRAIN SIGNALS AND RELATED
TECHNIQUES |
The waves recorded are thought to reflect the activity of
the surface of the brain, the cortex. The nerve cells in the
brain produce signals that are called action potentials.
These action potentials move from one cell to another
across a gap called the synapse. Special chemicals called
neurotransmitters help the signals to move across the
gap. There are two types of neurotransmitter: one will
help the action potential to move to the next cell, the
other will stop it moving to another nerve cell. The brain
has to work hard to keep equal amount of each of these
neurotransmitters in the brain. |
The figure 1 shows the frequency band of the beta waves
is13-30 Hz; these are detectable over the parietal and
frontal lobes. The alpha waves have the frequency
spectrum of 8-13Hz and can be measured from the
occipital region in an awake person when the eyes are
closed. The theta waves have the frequency range of 4-8
Hz and are obtained from children and sleeping adults.
The delta waves have the frequency range of 0.5-4 Hz
and are detectable in infants and sleeping adults. The
EEG signals are basically and closely related to the level
of consciousness of a person. When the eyes of the
subject are closed then alpha waves begin to have a very
strong influence over EEG. |
EXISTING METHOD |
A brain computer interface is a communication system
between human brain and the computer that enables
generation of control signals from brain signals such as
sensory motor rhythms and evoked potentials therefore, it
constitutes a novel communication option for people with
severe motor disabilities. The methods are |
1. The brain to computer interface (BCI): In this
method a person sits in front of computer and his
thought is transferred to the PC such that the person
can able to communicate with the system without
usage of keyboard and mouse. |
2. Brain-to-brain interface (BBI): In this method, the
interface is designed to allow a human to control an
animal/human through his thoughts. Its function is by
making the human wear a EEG-based BCI.A mouse on
the other hand is made to wear a focused ultrasound
(FUS) computer-brain interface (CBI). FUS allows a
particular region of neurons in the rat’s brain through
an ultrasound signal. |
DISADVANTAGES |
There is no displaying device for the thoughts of the
person what they think in terms of any alphanumerical or
symbol representation. |
PROPOSED METHOD |
Human communication, nonverbal information such as
intention and emotions, plays an important role,
especially by using information of emotion people can
communicate with each other more smoothly. The
emotion recognition systems in speech or facial
expressions which have been used include several
emotional states such as joy, fear, sadness, disgust,anger,
surprise and neutral.The block diagram of Proposed
method is shown in the Figure 2. |
The basic idea of BCI is to translate user produced
patterns of brain activity into corresponding commands.
A typical BCI is composed of signal acquisition and
signal processing (including preprocessing, feature
extraction, and classification).
Although some BCI systems do not include all
components and others group two or three components
into one algorithm, most systems can
be conceptually divided into signal acquisition,
preprocessing, feature extraction, and classification. |
1) Suitable Brain Signals: The brain signals that are
widely used to develop EEG-based BCIs include P300 potentials, which are a positive potential deflection
on the ongoing brain activity
at a latency of roughly 300 ms after the random
occurrence of a desired target stimulus from nontarget
stimuli (the stimuli can be in visual, auditory, or tactile
modality). |
2)Electroencephalogram Signal Acquisition and
Processing: After the suitable brain signal used to
develop the EEG based BCI is determined, EEG signal
acquisition and processing need to be performed so as to
build the BCI system. |
A)Signal Acquisition:EEG signals can be collected with
electrodes that are placed on the surface of the scalp. The
most widely used electrodes are silver/silver chloride
(Ag/AgCl) because they have low cost, low contact
impedance, and relatively good stability. |
Another important issue of EEG acquisition is the
locations on the scalp where electrodes are placed.
Generally, the electrodes are placed according to the
standard of 10–20 international system, which means
that electrodes are located on the scalp at 10% and 20%
of a measured distance from reference sites including
nasion, inion, left, and right preauricular as
Shown in Fig. 4.The EEG signals are recorded at
locations of the inferior frontal, central, and parietal regions (e.g., F3, F4, C3, C4, P3, P4, P7, P8, F7, and
F8);for ERD BCIs, some electrode locations at frontal,
central, and parietal regions are selected to acquire EEG
signals (e.g., F3, F4, C3, Cz, C4, P3, Pz, and P4),
whereas, for SSVEP BCIs, electrodes are placed at the
occipital region (e.g., O1, O2, and OZ). |
B)Signal Processing:The acquired signals are first
preprocessed in order to remove artifacts such as power
line noise, electromyogram (EMG),
electrocardiogram(ECG), electroculogram (EOG), and
body movement. Features such as the inputs to the
classifier are then extracted from the preprocessed
signals. Finally, the classifier translates these extracted
features into commands that subjects desire to output. |
The simplest and most widely used method to remove
artifacts is filtering including low-pass, high-pass, bandpass,
and notch filtering, which is appropriate to remove
line noise and other frequency-specific noise such as
body movement. This is done before feature extraction to
increase signal to noise ratio (SNR). This process is decomposing or de-noising the captured signal in order
to remove noise andto enhance the EEG signal.Various
steps are as follows. |
1.Sampling - Signal is sampled at 128 Hz.
The range of beta and mu waves is 8 to 13 Hz and
13 to 30 Hzrespectively, so it is sampled. |
2.Filtering-The traditional way to improve SNR
is to filter the EEG signals using band pass filter. Here
thesampled signal is subjected to low pass filter to
blocksignals above 30 Hz. |
3.Channelextraction - Processing the whole edf file
iscomplex, so signal values from required channel
areextracted out separately from the filtered signal. |
4.Wave Decomposition - The length of the wave
isdecreased by reducing the number of values in
signaland retaining the original waveform. Here the
signal isreduced to 1/4th of the original signal using
DiscreteWavelet Transformation (DWT) function.
We use EEGLAB to get the raw signal produced from
emotive headset available in MATLAB. pop_biosig()
function is used to make raw EEG signal in EDF
format available in MATLAB. This function takes the
pathname of EDF file and parameters. |
C)Feature extraction: To make classifiers of BCI
systems have good performance, features that can
significantly distinguish different classes are extracted.
Features that are applied to BCI systems of braincontrolled
mobile devices can be divided into two main
categories: features in time domain which are typically
amplitudes of event-evoked potentials and features in
frequency domain, which are typically frequency power
spectra of EEG signals that can be estimated with
Welch’s periodogram algorithm or other estimation
algorithms. |
D)Classification: A variety of classifiers have been used
to translate these extracted features from EEG signals
into an output command, from simple classifiers such as
nearest neighbor, linear discriminant analysis (LDA), to
nonlinear neural networks(NN), support vector machines
(SVM), and statistical classifiers.Here we assign a class
to set of features extracted from the signal. This class
corresponds to the kind of the metal state identified. |
The feature extraction and matching part are coded in
MATLAB. |
E)Channel extraction:EDF file has raw EEG signal
recorded with 35 channels. Processing the whole EDF
file is complex, so signal values from required channel
are extracted out separately from the raw EEG signal.
We are interested in O7 and O8 channels among the
35 channel. It is the 9th and 12th row in 35*5632 matrix.
The code to extract O1 and O2 channel is A number of digital signal analysis techniques have been
developed and applied to represent the transient sound
signals. Discrete wavelet transform is the most
suitable of these techniques because ofits localization in
both time and frequency.Wavelet transform is a spectral
estimation technique in
which any general function can be expressed as an
infinite series of wavelets. The basic idea underlying
wavelet analysis consists of expressing a signal as a
linear combination of a particular set
of functions (wavelet transform, WT), obtained by shifting and dilating one single function called a mother
wavelet. The decomposition of the signal leads to a set of
coefficients called wavelet coefficients. |
Therefore the signal can be reconstructed as a linear
combination of the wavelet functions weighted by the
wavelet coefficients. In order to obtain an exact
reconstruction of the signal, adequatenumber of
coefficients must be computed. The key feature of
wavelets is the time-frequency localization. It means that
most of the energy of the wavelet is restricted to afinite
time interval. Frequencylocalization means that the
Fourier transform is band limited. The advantage
oftime-frequency localization is that wavelet analysis
varies the time-frequency aspect ratio, producing
good frequency localization at low frequencies (long
time windows), and good time localization at high
frequencies (short time windows). This produces a
segmentation, or tiling of the time-frequency plane that is
appropriate for most physical signals, especially those of
atransient nature.The wavelet technique applied to the
EEG signal will reveal features related to the transient
nature of the signal, which are not obvious by the Fourier
transform. In general, it must be said that no timefrequency
regions but rather time-scale regions are
defined. All wavelet transforms can be specified in terms
of a low-pass filter, which satisfies the standard
quadrature mirror filter condition. |
The extracted wavelet coefficients provide a
compact representation that shows the energy
distribution of the EEG signal in time and frequency.
The frequencies corresponding to different levels of
decomposition for Daubechiesorder-4 wavelet with a
sampling frequency of 128 Hz.Inorder to further
decrease the dimensionality of the extracted feature
vectors, statistics over the set of the wavelet
coefficients was used. The following statistical
features wereused torepresent the time frequency
distribution of the EEG signals: Maximum of the
wavelet coefficients in each Sub-band. Minimum of the
wavelet coefficients in each Sub-band. Mean of the
wavelet coefficients in each sub-band.Standard deviation
of the wavelet coefficients in eachsub-band. |
F)Speechsynthesis:The textual data corresponding to
the trained signal is given to the speech synthesizer.
Speech synthesizer is the component that produces
artificial speech for the given text as input. This allows
java applications to incorporate speech technology in to
the user interface. It defines a cross platform API to
support to command and control dictation system. |
CONCLUSION |
The human computer interaction in concern with the
recognition of emotion in a person with the help of
Electroencephalogram (EEG) signals and speech. EEG
uses an electrical activity of the neurons inside the brain.
EEG machine is used for acquisition of the electrical
potential generated by the neurons when they are active.
Speech is the most natural form of communication. A
much of work is been done in speech recognition in
various languages. It is one of the components that
closely related to emotions. Very less work has been
carried out using combine aspects of speech, emotion
and EEG. Speech recognition is the process of converting an acoustic signal captured by a microphone
or a telephone to a set of words. The recognized words
can be the final results as for applications such as
commands & control, data entry and document
preparation.Emotion recognition by computers is
becoming very popular.This paper attempts to present
various techniques that can be used to recognize
emotions using speech and EEG brain signals. |
The communication system for person with severe
disabilities helps him to express thoughts for translating
their actions into activity using BCI. This improves the
quality of life of dumb people. The advantage of this
proposed work is there is no need of any caretakers for
assistance of patients and can be controlled by user
alone. |
Figures at a glance |
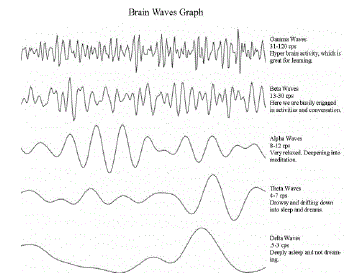 |
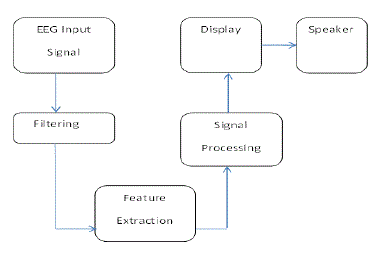 |
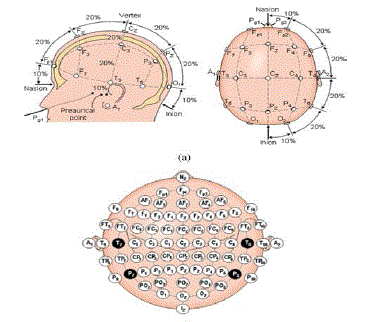 |
Figure 1 |
Figure 2 |
Figure 3 |
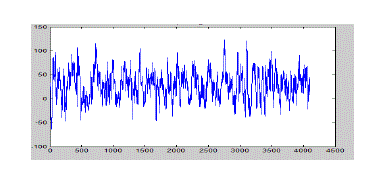 |
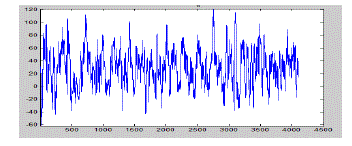 |
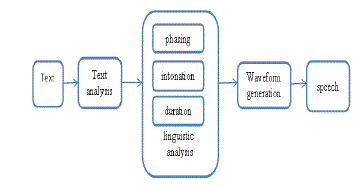 |
Figure 4 |
Figure 5 |
Figure 6 |
|
|
References |
- StaffordMichahial, R.RanjithKumar,Hemath Kumar P,Puneeth Kumar A,Speech synthesizer And Feature Extraction using DWT with classification By Euclidian Distance and neural network of EEG signals, International Journal of Advanced Research in Computer and Communication Engineering Vol. 1, Issue 4, June 2012.
- Lun-De Liao,Chin-TengLin,KalebMcDowell,Alma E. Wickenden,KlausGramann, Tzyy-Ping Jung,Li-Wei Ko,Jyh-Yeong Chang, Member,IEEE, Biosensor Technologies for Augmented Brain- Computer Interfaces in the Next Decades, Proceedings of the IEEE Vol. 100, May 13th, 2012.
- Luzheng Bi, Xin-An Fan, and Yili Liu, Member, IEEE,EEG-Based Brain-Controlled Mobile Robots:A Survey, IEEE transactions on human-machine systems, vol. 43, no. 2, march 2013.
- PriyankaAbhang,ShashibalaRao,Bharti W. Gawali,PramodRokade, Member,IEEE, Emotion Recognition using Speech and EEG Signal âÃâ¬Ãâ A Review,International Journal of Computer Applications (0975 âÃâ¬Ãâ 8887) Volume 15âÃâ¬Ãâ No.3, February 2011.
|