The quality of life of people suffering from severe motor disabilities can benefit from the use of current assistive technology capable of ameliorating communication, house-environment management and mobility, according to the user’s residual motor abilities. Brain–computer interfaces (BCIs) are systems that can translate brain activity into signals that control external devices. Thus they can represent the only technology for severely paralyzed patients to increase or maintain their communication and control options. Here we report on a pilot study in which a system was implemented and validated to allow disabled persons to improve or recover their mobility (directly or by emulation) and communication within the surrounding environment. The system is based on a software controller that offers to the user a communication interface that is matched with the individual’s residual motor abilities. Patients (n = 14) with severe motor disabilities due to progressive neurodegenerative disorders were trained to use the system prototype under a rehabilitation program carried out in a house-like furnished space. All users utilized regular assistive control options (e.g., microswitches or head trackers). In addition, four subjects learned to operate the system by means of a non-invasive EEG-based BCI. This system was controlled by the subjects’ voluntary modulations of EEG sensorimotor rhythms recorded on the scalp; this skill was learnt even though the subjects have not had control over their limbs for a long time. We conclude that such a prototype system, which integrates several different assistive technologies including a BCI system, can potentially facilitate the translation from pre-clinical demonstrations to a clinical useful BCI.
Keywords |
EEG-based brain–computer interfaces; Assistive robotics; Severe motor impairment; Technologies for
independent life |
INTRODUCTION |
The ultimate objective of a rehabilitation program is the reduction of the disability due to a given pathological condition,
that is, the achievement for that clinical status of maximum inde- pendence by means of orthoses and by the management
of the social disadvantages related to the disability by using different types of aids. |
Recently, the development of electronic devices that are capable of assisting in communication and control needs (such
as environmental control or assistive technology) has opened new avenues for patients affected by severe movement
disorders. This development includes impressive advancements in the field of robotics. Indeed, the morphology of
robots has remarkably mutated: from the fixed-base industrial manipulator, it has evolved into a variety of
mechanical structures. These structures are often capable of locomotion using either wheels or legs [17]. As a direct
consequence, the domain of robots’ application has increased substantially, including assistance to hospital patients and
disabled people, automatic surveillance, space exploration and many others [23]. In the case of robotic assistive devices
for severe motor impairments, they still suffer from limitations due to the necessity of residual motor ability (for
instance, limb, head and/or eye movements, speech and/or vocalization). Patients in extreme pathological conditions
(i.e., those that do not have any or only unreliable remaining muscle control) may in fact be. |
prevented from use of such systems. Brain–computer interface (BCI) technology “gives their users communication and
control channels that do not depend on the brain’s normal output channels of peripheral nerves and muscles.” [22],
and can allow completely paralyzed individuals to communicate with the sur- rounding environment [2,7]. A BCI
detects activation patterns in the brain that correspond to the subject’s intent. Whenever the user induces a voluntary
modification of these patterns, the BCI system is able to detect it and to translate it into an action that reflects the user’s
intent. Several animal and some human studies have shown the possibility to use electrical brain activity recorded
within the brain to directly control the movement of robots or prosthetic devices in real time using microelectrodes
implanted within the brain [3,19,16,5,15]. Other BCI systems depend on brain activity recorded non-invasively from
the surface of the scalp using electroencephalography (EEG). EEG-based BCIs can be operated by modulations of
EEG rhythmic activity located over scalp sensorimotor areas that are induced by motor imagery tasks [21]; these
modulations can be used to control a cursor on a computer screen [20] or a prosthetic device for limited hand
movements [13,11]. Thus, it has become conceivable to extend the communication between disabled individuals and
the external environment from mere symbolic interaction (e.g. alphabetic spelling) to aid for mobility. A pioneering
application of BCI consisted of controlling a small mobile robot through the rooms of a model house [10]. The
recognition of mental activity could be put forward to guide devices (mobile robots) or to interact naturally with
common devices within the external word (telephone, switch, etc.). This possible application of BCI technology has not
been studied yet. Its exploration was the principal aim of this study. |
These considerations prompted us to undertake a study with the aim of integrating different technologies (including a
BCI and a robotic platform) into a prototype assistive communica- tion platform. The goal of this effort was to
demonstrate that application of BCI technology in people’s daily life is possi- ble, including for people who suffer from
diseases that affect their mobility. The current study, which is part of a project named ASPICE, addressed the
implementation and validation of a technological aid that allows people with motor disabilities to improve or recover
their mobility and communicate within the surrounding environment. The key elements of the system are: |
(1) Interfaces for easy access to a computer: mouse, joystick, eye tracker, voice recognition, and utilization of signals
collected directly but non-invasively from the brain using an EEG-based BCI system. The rationale for the multiple
access capacities was twofold: (i) to widen the range of users, but tailoring the system to the different degrees of patient
disability; (ii) to track individual patient’s increase or decrease (because of training or reduction of abilities,
respectively) to interact with the system, according to the residual muscular activity present at the given moment of the
disease course and eventually to learn to control the system with different accesses (up to the BCI) because of the
nature of neurodegenerative diseases which provoke a time progressive loss of strength in different muscular segments.
(2) Controllers for intelligent motion devices that can follow complex paths based on a small set of commands.
(3) Information transmission and domotics that establish the information flow between subjects and the appliances
they are controlling. |
The goal pursued by designing this system was to fulfill needs (related to several aspects of daily activities) of a class
of neu- romuscular patients by blending several current technologies into an integrated framework. We strove to use
readily available hardware components, so that the system could be practically replicated in other home settings.
The validation of the system prototype has been initially realized with the participation of healthy volunteers and subsequently
with subjects with severe motor disabilities due to progressive neurodegenerative disorders. The disabled
subjects described in this report were trained to use the system prototype with different types of access during a
rehabilitation program carried out in a house-like furnished space. |
MATERIALS AND METHODS |
Subjects and clinical experimental procedures |
In this study, 14 able-bodied subjects and 14 subjects suffering from Spinal Muscular Atrophy type II (SMA II) or
Duchenne Muscular Dystrophy (DMD) underwent system training. These neuromuscular diseases cause a progressive
and severe global motor impairment that substantially reduces the subject’s autonomy. Thus, these subjects required
constant support by nursing staff. Sub- jects were informed regarding the general features and aims of the study, which
was approved by the ethics committee of the Santa Lucia Foundation. All sub- jects (and their relatives when required) gave their written informed consent. In particular, an interactive discussion with the patients and their relatives allowed
assessment of the needs of individual patients. This allowed for appropriate sys- tem customization. The characteristics
of these patients are reported in Table 1. In general, all patients have been unable to walk since they were adolescent.
They all relied on a wheelchair for mobility. All wheelchairs except two were electrically powered and were controlled
by a modified joystick that could be manipulated by either the residual “fine” movements of the first and second finger
or the residual movements of the wrist. All patients had poor residual muscular strength either of proximal or distal arm
muscles. Also, all patients required a mechanical support to maintain neck posture. Finally, all patients retained effective
eye movement control. Prior to the study, no patient used technologically advanced aids. |
The clinical experimentation took place at the Santa Lucia Foundation and Hospital where the system prototype
(ASPICE) was installed in a three-room space that was furnished like a common house and devoted to Occupational
Ther- apy. Patients were admitted to the hospital for a neurorehabilitation program. The first step in the clinical
procedure consisted of an interview and physi- cal examination performed by the clinicians. This interview determined
several variables of interest as follows: the degree of motor impairment and reliance on the caregivers for everyday
activities, as assessed by current standardized scale (Barthel Index, BI for ability to perform daily activities [8]); the
familiarity with transducers and aids (sip/puff, switches, speech recognition, joysticks) that could be used as input to
the system; the ability to speak or communicate with an unfamiliar person; the level of informatics alphabetization
measured by the number of hours/week spent in front of a computer. Corresponding questions were structured in a
questionnaire that was administered to the patients at the beginning and end of the training. A level of system
acceptance by the users was schematized by asking the users to indicate with a number ranging from of the output devices controlled by the most individual adequate access. The training consisted of weekly sessions; for
a period of time ranging from 3 to 4 weeks (except in the case of BCI training, see below), the patient and (when
required) her/his caregivers were practicing with the system. During the whole period, patients had the assistance of an
engineer and a therapist who facilitated interaction with the system |
System prototype input and output devices |
The system architecture, with its input and output devices, is outlined in Fig. 1. A three-room space in the hospital was
furnished like a com- mon house, and the actuators of the system were installed. Care was taken to make an installation
that would be easily replicable in most houses. The place was provided with a portable computer to run the core
program (see Section 3). This core program was interfaced with several input devices that supported a wide range of motor capacities from a wide variety of users. For instance, keyboard, mouse, joystick, trackball touchpad and buttons
allowed access to the system through upper limb residual motor abilities. Other- wise, microphone and head
tracker could be used when motor disability was extremely impairing for the limbs but the neck muscles or
comprehensi- ble speech were preserved. Thus, we could customize these input devices to the users’ residual motor
abilities. In fact, users could utilize the aids they were already familiar with (if any), and that have been interfaced to
provide a low level input to a more sophisticated assistive device. On the other hand, the variety of input devices
provided robustness to the decrease. |
The figure shows that the system interfaces the user to the surrounding environment. The modularity is assured by the
use of a core unit that takes inputs by one of the possible input devices and sends commands to one or more of the
possible actuators. Feedback is provided to keep the user informed about the status of the system. of patient’s ability,
which is a typical consequence of degenerative diseases. |
When the user was not able to master any of the above mentioned devices, or when the nature of a degenerative disease
suggested that the patient may not be able to use any of the devices in the future, the support team proposed to the
patient to start training on the use of a BCI. |
As for the system output devices, we considered (also based upon patient’s needs/wishes), a basic group of domotics
appliances such as neon lights and bulbs, TV and stereo sets, motorized bed, acoustic alarm, front door opener,
telephone and wireless cameras (to monitor the different rooms of the house ambient). The system also included a
robotic platform (a Sony AIBO) to act as an extension of the ability of the patient to move around the house (“virtual”
mobility). The AIBO was meant to be controlled from the system control unit in order to accomplish few simple
tasks with a small set of commands. As previously mentioned, the system should cope with a variety of disabilities
depending on the patient conditions. There- fore, three possible navigation systems were designed for robot
control: single step, semi-autonomous, and autonomous mode. Each navigation mode was associated with a Graphical
User Interface in the system control unit (see Section 3). |
Brain–computer interface (BCI) framework and subject training |
As described, the system contained a BCI module meant to translate com- mands from users that cannot use any of the
conventional aids. This BCI system was based on detection of simple motor imagery (mediated by modulation of sensorimotor
EEG rhythms) and was realized using the BCI2000 software system [14]. Users needed to learn to modulate
their sensorimotor rhythms to achieve more robust control than the simple imagination of limb movements can produce.
Using a simple binary task as performance measure, training is meant to improve performances from 50–70% to
80–100%. An initial screening session suggested, for each subject, the signal features (i.e., amplitudes at particular
brain locations and frequencies) that could best discriminate between imagery and rest. The BCI system was then
configured to use these brain signal feature, and to thus translate the user’s brain signals into output control signals that
were communicated to the ASPICE central unit. |
During the initial screening session, subjects were comfortably seated on a reclining chair (or when necessary a
wheelchair), in an electrically shielded, dimly lit room. Scalp activity was collected with a 96 channel EEG system
(BrainAmp, Brainproducts GmbH, Germany). EEG data sampling frequency was 200 Hz; signals were bandpassfiltered
between 0.1 and 50 Hz before digi- tization. In this screening session, the subject was not provided with any
feedback (any representation of her/his brain signals). The screening session consisted of alternate and random
presentation of cues on opposite sides of the screen (either up/down, i.e., vertical, or left/right, i.e., horizontal). In
coupled runs, the sub- ject was asked to execute (first run) or to image (second run) movements of her/his hands or feet
upon the appearance of top or bottom target, respectively. In horizontal runs, the targets appeared on the left or right
side of the screen and the subject was asked to move (odd trials) or to imagine (even trials) his/her left or right hand. In
vertical runs, the targets appeared on top or bottom of the screen, and the subject had to concentrate on his/her upper or
lower limbs. This sequence was repeated three times for a total of 12 trials. |
We then analyzed the brain signals recorded during these tasks offline. In these analyses, we compared brain signals
associated with the top target to those associated with the bottom target, and did the same for left and right targets.
These analyses aimed at detecting a set of EEG features that maximized pre- diction of the current cue. The analysis
was carried out by replicating the same signal conditioning and feature extraction that was subsequently used in on-line
processing (training session). Data sets were divided into epochs (usually 1 s long) and spectral analysis is performed
by means of a Maximum Entropy algo- rithm with a resolution of 2 Hz. Differently from the on-line processing, when
the system only computes the few features relevant for BCI control, all possible features in a reasonable range (i.e., 0–
60 Hz in 2 Hz bins) were extracted and analyzed simultaneously. A feature vector was extracted from each epoch. This
vector was composed of the spectral amplitude at each frequency bin for each channel. When all features in the two
datasets under contrast were extracted, a statistical analysis (r2 , i.e., the proportion of the total variance of the signal
amplitude accounted for by target position [9]) was performed to assess signifi- cant differences in the values of each
feature in the two conditions. At the end of this process, r2 values were compiled in a channel-frequency matrix and
head topography (examples are shown in Figs. 3 and 4 in Section 3) and evaluated to identify the set of candidate
features to be enhanced with training. |
During the following training sessions, the subjects were provided feedback of these features, so that they could learn
how to improve their modulation. A subset of electrodes (out of the 59 placed on the scalp according to an extension of
the 10–20 International System) were used to control the movement of a computer cursor, whose position was
controlled in real time by the amplitude or the subject’s sensorimotor rhythms. Each session lasted about 40 min and
consists of eight 3-min runs of 30 trials each. We collected a total of 5–12 training sessions for each patient; training
ended when performance was stabilized. Each subject’s performance was assessed by accuracy (i.e., the percentage of
trials in which the target was hit) and by r2 value. The training outcome was monitored over sessions. Upon successful
training, the BCI was connected to the prototype system, and the subject was asked to utilize its button interface using
BCI control. |
During experimentation with the ASPICE system, BCI2000 was config- ured to stream its output (current cursor
position) in real time over a TCP/IP connection. Goals of the cursor were dynamically associated with an action of the
system, similarly to commands issued through the other input devices (e.g. button presses). |
RESULTS |
System prototype and robotic platform implementation |
Implementation of the prototype system core started at the beginning of this study, and its successive releases took
advan- tage of advice and daily interaction with the users. It was eventually realized as follows. |
The core unit received the logical signals from the input devices and converted them into commands that could be used
to drive the output devices. Its operation was organized as a hier- archical structure of possible actions, whose
relationship could be static or dynamic. In the static configuration, it behaved as a “cascaded menu” choice system and
was used to feed the feed- back module only with the options available at the moment (i.e. current menu). In the
dynamic configuration, an intelligent agent tried to learn from use which would have been the most probable choice the user will make. The user could select the commands and monitor the system behavior through a graphical interface.
Fig. 2A shows a possible appearance of the feedback screen, including a feedback stimulus from the BCI. The
prototype sys- tem allowed the user to operate remotely electric devices (e.g. TV, telephone, lights, motorized bed,
alarm, and a front door opener) as well as monitoring the environment with remotely controlled video cameras. While
input and feedback signals were carried over a wireless communication, so that mobility of the patient was minimally
affected, most of the actuation commands were carried via a powerline-based control system. |
The robotic platform (AIBO, Fig. 2B) was capable of three navigation modes that allowed us to serve the different
needs of the users. The first mode was single-step navigation. In this mode, the user had complete control of robot
movement. This was useful for fine motion in cluttered areas. The second mode was semi-autonomous navigation. In
this mode, the user spec- ified the main direction of motion and the robot automatically avoided obstacles. The third
and final mode was autonomous navigation. In this mode, the user specified the target destination in the apartment
(e.g., the living room, the bedroom, the bath- room, and the battery charging station). The robot autonomously traveled
to the target. This mode was useful for quickly reaching some important locations, and for enabling AIBO to charge its
battery autonomously when needed. We expected that this mode would be particularly useful for severely impaired
patients who may be unable to send frequent commands. All three navigation modes contained some level of obstacle
avoidance based on a two-dimensional occupancy grid (OG) built by the on-board laser range sensor, with the robot
either stationary or in motion. |
In single-step mode, the robot was driven, with a fixed step size, in one of six directions (forward, backward, lateral
left/right, clockwise or counter clockwise rotations). Before performing the motion command, the robot generated an
appro- priate OG (oriented along the intended direction of motion) to verify whether the step could be performed
without colliding with obstacles. Depending on the result of the collision check, the robot decided whether or not to
step in the desired direction. |
In semi-autonomous mode, the user specified a general direc- tion of motion. Instead of executing a single step, the
robot walked continuously in the specified direction until it received a new command (either a new direction or a stop).
Autonomous obstacle avoidance was obtained by the use of artificial poten- tial fields. The OG was generated as the
robot moved, and then used to compute the robot velocities. Our algorithm used vortex and repulsive fields to build the
velocity field. The velocity field was mapped to the configuration space velocities either with omnidirectional
translational motion or by enforcing nonholonomic-like motion. The first conversion was consistent with the objective
of maintaining as much as possible the robot orientation specified by the user whereas with the second kind of
conversion, the OG provided more effective collision avoidance. |
In autonomous navigation mode, the user controlled robot movement towards a fixed set of destinations. To allow the
robot to autonomously reach these destinations, we designed a physical roadmap that connected all relevant
destinations in the experimental arena. The robot used a computer vision algorithm to navigate. The roadmap consisted
of streets and crossings, |
Fig. 2. Panel A: Appearance of the feedback screen. In the feedback application, the screen is divided into three
panels. In the top panel, the available selections (commands) appear as icons. In the bottom right panel, a feedback
stimulus by the BCI (matching the one the subject has been training with) is provided. The user uses modulation of brain activity to move the cursor at the center to hit either the left or the right bars – in order to focus the previous or
following icon in the top panel – or to hit the top bar – to select the current icon. In the bottom left panel, the feedback
module displays the video stream from the video camera that was chosen beforehand in the operation. Panel B: An
experiment of BCI-controlled navigation of the AIBO mobile robot. Here, the user is controlling the BCI to emulate a
continuous directional joystick mode which drives the robot to its target (the bone). The robot automatically avoids
obstacles. |
which were marked on the floor using white adhesive tape. Edge detection algorithms were used to visually identify
and track streets (i.e., straight white lines) and crossings (i.e., coded squares), while path approaching and following
algorithms were used to drive the robot. The robot behavior was represented by a Petri Nets based plan. The robot
traveled towards the selected destination using a series of cascaded actions. Initially, the robot sought a street. When it
detected a street, the AIBO approached it and subsequently followed it until at least one crossing was detected. Then,
the robot identified its position and orientation on the roadmap. The robot then used a Dijkstra-based graph search to
find the shortest path to its destination. Depending on the result of the graph search, the robot approached and followed
another street (repeat the corresponding actions in the plan), or stop if the crossing corresponded to the desired
destination. |
The three navigation modes were compared in a set of exper- iments in which some of the able-bodied users controlled
the robot to move from a source to a destination. The task was repeated 5 times for each of the three navigation modes
and results were averaged. A mouse was used as input device for all modes. In semi-autonomous navigation,
omnidirectional trans- lational motion was used for mapping desired user velocities to the configuration space.
Comparison between the three modes was based on execution time and user intervention (i.e., num- ber of times the
user had to intervene by clicking on the GUI for updating the commands; Table 2). According to the average execution
time and user intervention, the qualitative properties expected for each mode were confirmed. |
User feedback drew our attention to the noise produced by AIBO’s walking. We minimized the noise by reducing the
veloc- ity of the legs’ tips during ground contact. |
Finally, the robot could assist the users in visually moni- toring the environment and communicating with the caregiver.
Visual monitoring was achieved by transmitting a video stream acquired by the robot camera to the control unit over a
wireless connection; image compression was performed on-board before transmission. The robot could also be utilized
for communica- tion with the caregiver by requesting it to play pre-recorded vocal sentences (e.g., “I am thirsty” or
“Please come”) on its speakers.More information about the control strategy implemented for the AIBO, is available at
[18]. |
Clinical validation |
All 14 able-bodied subjects tested the successive releases of the system for 8–12 sessions. The purpose of system use
by able- bodied subjects was to validate system security and safety. The system input devices were all functionally
effective in control- ling the domotic appliances and the small robotic device (AIBO). At the time of the study, these
subjects were also enrolled in the BCI training with and without interfacing it with the system prototype. Early results
on BCI training will be reported in the pertinent section of this paper. |
Several patients (see Table 1) were also able to master the final release of the system within 5 sessions, performed once
or twice a week. According to the score of the BI, all patients depended almost completely on caregivers, especially
those with the diag- nosis of DMD (n = 6 subjects; BI score <35) who required artificial ventilation, had minimal
residual mobility of the upper limbs and very slow speech. Because of the high level of muscu- lar impairment, five of
the DMD patients had the best access to the system via joystick, which required minimal efficiency of the residual muscular contraction at the distal muscular segments of the upper limbs (minimal flexion-extension of the hand
fingers). One additional DMD patient found a trackball to be most com- fortable for her level of distal muscle strength
(third patient in was slightly higher compared to the DMA patients. Neverthe- less, the SMA patients also required
continuous assistance for daily life activity (BI ≤50). These patients had to the system via a joystick (3 patients),
touchpad (2 patients), keyboard (1 patient), and button (2 patients). The variety in the access devices in this class of
patients was related to a still functionally effective residual motor abilities of the upper limbs (mainly proximal
muscles), both in terms of muscular strength and range of movements preserved. None of the patients was comfortable
in accessing the system via head-tracker because of the weakness of the neck muscles. At the end of the train- ing, all
patients were able to control the domotic appliances and the robotic platform using one of the mentioned input methodologies.
According to the early results of the questionnaire, all patients were independent in the use of the system at the
end of the training and they experienced (as they reported) “the possi- bility to interact with the environment by
myself.” A schematic evaluation of the degree of the system acceptance by the users revealed that amongst the several
system outputs, the front door opener was the most accepted controlled device (mean score 4.93 in a range 1–5)
whereas the robotic platform (AIBO) received the lowest score (mean 3.64). Four of the motor impaired users had
interacted with the system via BCI (see below). |
We documented this overall clinical experience in a system manual for future use by users and installers, and also
described suggested training guidelines. This manual will eventually be available to the community. |
Brain–computer interface (BCI) application |
Over the 8–12 sessions of training, subjects acquired brain control with an average accuracy higher than 75% (accuracy
expected by chance alone was 50%) in a binary selection task. Table 3 shows the average accuracy for the last 3 of the
8–12 training sessions for each subject. As shown in Fig. 3 for one representative normal subject (Subject 1 in Table 3),
the topo- graphical and spectral analysis of r2 values revealed that since the beginning of the training, motor cortical
reactivity was |
Top panel: topographical maps of r2 values during the first (to the left) and the last (to the right) training sessions, for
EEG spectral features extracted at 14 Hz. The patterns changed both in spatial distribution and in absolute value (note
the different color scales). Bottom panel: time course of BCI performance over training sessions, as measured by the
percentage of correctly selected targets. Error bars indicate the best and the worst experimental run in each session. |
localized over sensorimotor scalp areas. This pattern persisted over training and corresponded to good performance in
cursor control. Four patients out of 14 underwent a standard BCI train- ing (Table 3, P1–4). Similar to healthy subjects,
these patients acquired brain control that supported as accuracies above 60% in the standard binary decision task. The
patients employed imagery of foot or hand movements. Brain signal changes asso- ciated with these imagery tasks were
mainly located at midline centro-parietal electrode positions. Fig. 4 shows for one rep- resentative patient (second row
in Table 1; P1 in Table 2) in a session near the end of training, the scalp topography of r2 at the frequency used to
control the cursor with an average accuracy of 80%. In this case, control was focused at Cz (i.e., the vertex of the head).
When BCI training was performed in the system environ- ment, the visual feedback from the BCI input device was
included into the usual application screen (bottom right panel of the screen in Fig. 2A) Through this alternative input,
healthy subjects could control the interface by using two targets to scroll through the icons and to select the current
icon, respectively. One more icon was added to disable selection of commands (turn off BCI input) and a combination
of BCI targets was programmed to re-establish BCI control of the system. All 4 patients were able to successfully
control the system. However, system per- formance achieved in these patients using the BCI input was lower than hat
for muscle-based input. |
DISCUSSION |
The quality of life of an individual suffering from severe motor impairments is importantly affected by its complete
dependence upon the caregivers. An assistive device, even the
most advanced, cannot substitute – at the state of the art – the |
Fig. 4. EEG patterns related to the intentional brain control in a SMA patient. Left panel: spectral power density of the
EEG of the most responsive channel. Red and blue lines correspond to the subset of trials in which the user tried to hit
the top and the bottom target, respectively. Right Panel: Topographical distributions of r2 values at the most responsive frequency (33 Hz). The red colored region corresponds to those regions of the brain that exhibited brain control. (For
interpretation of the references to color in this figure legend, the reader is referred to the web version of the article.)
assistance provided by a human. Nevertheless, it can contribute to relieve the caregiver from continuous presence in the
patient’s room since the patient can perform some simple activities on his/her own. Most importantly, because the
patient can call the attention of the caregiver using some form of alarm. This sug- gests that the cost of care for patients
in stable conditions could be reduced since the same number of paramedics or assistants can care after a higher number
of patients. In a home environ- ment, the life of familiars can be less hardly affected by the presence of the impaired
relative. In this respect, the prelimi- nary findings we reported would innovate the concept of assistive technology
device and they may bring it to a system level, that is, the user is no more given many devices to perform sepa- rate
activities but the system provides unified (though flexible) access to all controllable appliances. Moreover, we
succeeded in the effort of including many commercially available com- ponents in the system, so that affordability and
availability of components is maximized. |
From a clinical perspective, the perception of the patient, as revealed by the analysis of questionnaires, is that he/she
does not have to rely on the caregiver for all tasks. This may increase the patient’s sense of independence. In addition,
this indepen- dence grants a sense of privacy that is absent when patients have to rely on caregivers. For these two
reasons, the patients reported to expect that their quality of life would substantially improve if they could use such a
system in their homes. As an additional indication that supports this notion, the patients selected the front door opener
as their favorite output device. The ability to decide autonomously or at least to participate to the decision on who can
be part of their life environment at any given moment was systematically reported as highest in sys- tem acceptance.
The possibility to control the robot received a lower acceptance score, although the patients were well aware of the
potential usefulness of the device as virtual mobility in the house. At least one main aspect has to be considered in
inter- preting these findings: the higher level of demand in controlling the robot, that in turn increases the probability of
failure and the level of the related sense of frustration. Although further studies are needed in which a larger cohort of
patients is confronted with the system and a systematic categorization of the system impact on the quality of life should
take into account a range of out- comes (e.g. mood, motivation, caregiver burden; employability; satisfaction) [6,1,12],
the results obtained from this pilot study are encouraging for the establishment of a solid link between the field of
human machine interaction and neurorehabilitation strategy [4]. |
Exploration of potential impact of BCI on the users’ inter- action with the environment is peculiar to this work when
compared to the previous studies on the usefulness of the BCI- based interfaces, i.e. [7,20,11]. Although the
improvement of quality-of-life brought by such an interface is expected to be relevant only for those patients who are
not able to perform any voluntarily controlled movement, the advances in the BCI field are expected to increase the
performance of this communication channel, thus making it effective for a broader population of individuals. Upon
training, the able-bodied subjects enrolled in this study were able to control a standard application of the BCI (i.e. a
cursor moving on a screen as implemented in the BCI2000 framework) by modulating their brain activity recorded over
the scalp centro-parietal regions, with an overall accuracy over 70%. Similar levels of performance were achieved by
the patients who underwent BCI training with standard cursor control applica- tion. All patients displayed brain signal
modulations over the expected centro-parietal scalp positions. This confirms findings in [7,20,11] and extends them to
other neurological disorders (DMD and SMA). Our study is thus additional evidence that people with severely
disabling neuromuscular or neurologi- cal disorders can acquire and maintain control over detectable aspects of brain
signals, and use this control to drive output devices. When patients and control subjects were challenged with a
different application of the BCI, i.e., the system prototype rather than the cursor used in the training period,
performance in mastering the system were substantially maintained. This shows that an EEG-based BCI can be
integrated into an environmen- tal control system. Several important aspects yet remain to be addressed. This includes
the influence on BCI performance of the visual channel as the natural vehicle of information (in our case the set of
icons to be selected) and as BCI feedback channel (which is mandatory for the training and performing processes in the
actual “BCI task”). As mentioned above, motivation, mood, and other psychological variables are of relevance for a
success- ful user–machine interaction based on her/his residual muscle activity. This becomes crucial in the case of
severely paralyzed patients who are the eligible candidate for the BCI approach. |
In conclusion, in this pilot study, we integrated an EEG-based BCI and a robotic platform in an environmental control
system. This provides a first application of this integrated technology platform towards its eventual clinical significance. In particular, the BCI application is promising in enabling people to operate an environmental control
system, including those who are severely disabled and have difficulty using conventional devices that rely on muscle
control. |
ACKNOWLEDGEMENTS |
This work has been partially supported by the Italian Telethon Foundation (Grant: GUP03562) and by the National
Insti- tutes of Health in the USA (Grants: HD30146, EB00856, and EB006356). |
Tables at a glance |
|
|
Figures at a glance |
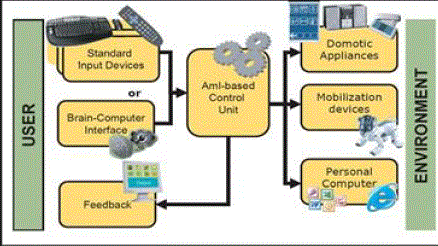 |
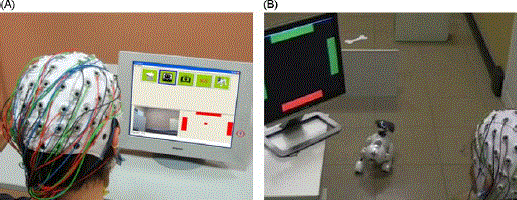 |
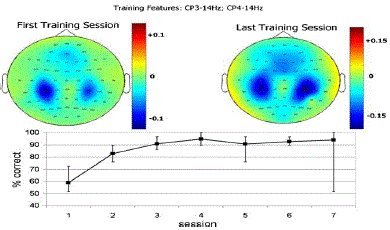 |
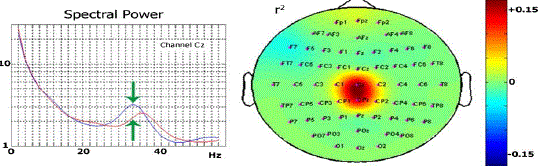 |
Figure 1 |
Figure 2 |
Figure 3 |
Figure 4 |
|
|
References |
- J.R. Bach, J. Vega, J. Majors, A. Friedman, Spinal muscular atrophy type 1 quality of life, Am. J. Phys. Med. Rehabil. 82 (2) (2003) 137– 142.
- N. Birbaumer, N. Ghanayim, T. Hinterberger, I. Iversen, B. Kotchoubey, A. Kubler, J. Perelmouter, E. Taub, H. Flor, A spelling device for the paralyzed, Nature 398 (1999) 297–298.
- J.K. Chapin, K.A. Moxon, R.S. Markowitz, M.A. Nicolelis, Real-time con- trol of a robot arm using simultaneously recorded neurons in the motor cortex, Nat. Neurosci. 2 (1999) 664–670.
- J. Hammel, Technology and the environment: supportive resource or barrier for people with developmental disabilities? Nurs. Clin. North Am. 38 (2) (2003) 331–349.
- L.R. Hochberg, M.D. Serruya, G.M. Friehs, J.A. Mukand, M. Saleh, A.H. Caplan, A. Branner, D. Chen, R.D. Penn, J.P. Donoghue, Neuronal ensem- ble control of prosthetic device by a human with tetraplegia, Nature 442 (2006) 164–171.
- M. Kohler, C.F. Clarenbach, L. Boni, T. Brack, E.W. Russi, K.E. Bloch, Quality of life, physical disability, and respiratory impairment in Duchenne muscular dystrophy, Am. J. Respir. Crit. Care Med. 172 (8) (2005) 1032–1036.
- A. Ku¨ bler, F. Nijboer, J. Mellinger, T.M. Vaughan, H. Pawelzik, G. Schalk, D.J. McFarland, N. Birbaumer, J.R. Wolpaw, Patients with ALS can use sensorimotor rhythms to operate a brain–computer interface, Neurology 64 (2005) 1775–1777.
- F. Mahoney, D. Barthel, Functional evaluation: the Barthel Index, Md. State Med. J. 14 (1965) 61–65.
- D.J. McFarland, et al., Spatial filter selection for EEG-based communica- tion, Electroencephalogr. Clin. Neurophysiol. 103 (3) (1997) 386–394.
- J. del R. Milla´n, F. Renkens, J. Mourin˜ o, W. Gerstner, Non-invasive brain- actuated control of a mobile robot by human EEG, IEEE Trans. Biomed. Eng. 51 (2004) 1026–1033.
- G.R. Muller-Putz, R. Scherer, G. Pfurtscheller, R. Rupp, EEG-based neu- roprosthesis control: a step towards clinical practice, Neurosci. Lett. (2005) 1–8, 382 (1–2) 169–174.
- B. Natterlund, L.G. Gunnarsson, G. Ahlstrom, Disability, coping and qual- ity of life in individuals with muscular dystrophy: a prospective study over five years, Disabil. Rehabil. 22 (17) (2000) 776–785.
- G. Pfurtscheller, G.R. Muller, J. Pfurtscheller, H.J. Gerner, R. Rupp, ‘Thought’-control of functional electrical stimulation to restore hand grasp in a patient with tetraplegia, Neurosci. Lett. 351 (1) (2003) 33–36.
- G. Schalk, D. McFarland, T. Hinterberger, N. Birbaumer, J. Wolpaw, BCI2000: a general-purpose brain–computer interface (BCI) system, IEEE Trans. Biomed. Eng. 51 (2004) 1034–1043.
- A.B. Schwartz, X.T. Cui, D.J. Weber, D.W. Moran, Brain-controlled inter- faces: movement restoration with neural prosthetics, Neuron 52 (2006) 205–220.
- M.D. Serruya, N.G. Hatsopoulos, L. Paninski, M.R. Fellows, J.P. Donoghue, Brain–machine interface: instant neural control of a movement signal, Nature 416 (2002) 141–142.
- R. Siegwart, I.R. Nourbakhsh, Introduction to Autonomous Mobile Robots, The MIT Press, 2004.
- The Aspice Project web page at the LabRob web site, http://www.dis. uniroma1.it/∼labrob/research/ASPICE.html, 2006.
- J. Wessberg, C.R. Stambaugh, J.D. Kralik, P.D. Beck, M. Laubach, J.K. Chapin, J. Kim, J. Biggs, M.A. Srinivasan, M.A. Nicolelis, Real-time pre- diction of hand trajectory by ensemble of cortical neurons in primates, Nature 408 (2000) 361–365.
- J.R. Wolpaw, D.J. McFarland, Control of a two-dimensional movement signal by a noninvasive brain–computer interface in humans, Proc. Natl. Acad. Sci. U.S.A. 51 (2004) 17849–17854.
- J.R. Wolpaw, N. Birbaumer, W.J. Heetderks, D.J. McFarland, P.H. Peck- ham, G. Schalk, E. Donchin, L.A. Quatrano, C.J. Robinson, T.M. Vaughan, Brain–computer interface technology: a review of the first international meeting, IEEE Trans. Rehabil. Eng. 8 (2000) 161–163.
- J.R. Wolpaw, N. Birbaumer, D.J. McFarland, G. Pfurtscheller, T.M. Vaughan, Brain–computer interfaces for communication and control, Clin. Neurophysiol. 113 (6) (2002) 767–791. [23] A. Zelinsky, Field and Service Robotics, Springer Verlag, 1997.
|