The main aim is Secondary Structure Prediction of proteins causing Diabetic Foot Ulcers using Artificial Neural Networks.Protein structure prediction is one of the most important goals pursued by bioinformatics. The knowledge or prediction of secondary structure improves detection and alignment of remote homologs and helps for drug design. The purpose of this study is to identify the Secondary Structure of proteins causing foot problems in patients with diabetes, which is a major public health concern these days. The most feared factor among the diabetic patients is lower extremity amputation. The sequence of events leading to amputation is initiated by ulceration combined with sensation loss. To prevent complications and amputations it is necessary to detect the foot at risk of plantar ulceration at an early stage of sensation loss. To access the severity of foot ulcer, here Artificial Neural Networks is used to predict the secondary structure of proteins like P14780, P01137, P01912, P18462, P30499. By using this method the recognition of risk factors will be analyzed in efficient manner. Based on the severity of foot ulcer, preventive foot maintenance and regular foot examinations will take place in diabetes patients.to an early diagnosis and treatment against diabetic foot.
Keywords |
Diabetes, Foot Ulcer, P14780, P01137, P01912, P18462, P30499. |
INTRODUCTION |
The amino acid sequence of a protein, the so-called primary structure, can be easily determined from the sequence
on the gene that codes for it. In the vast majority of cases, this primary structure uniquely determines a structure in its
native environment. Knowledge of this structure is vital in understanding the function of the protein. Protein structure
prediction is the prediction of the three-dimensional structure of a protein from its amino acid sequence — that is, the
prediction of its secondary, tertiary, and quaternary structure from its primary structure. The aim is to predict which
secondary structural element will be formed by each residue of the protein [1].the structure of a protein has different
levels and it has an energetically and structurally optimized form. The primary structure is the amino acid of the protein
and can be presented by a sequence with 20 letters, where each letter indicates an individual amino acid. The secondary
structure describes the areas in the primary structure where secondary structure elements occur in the back bone of the
protein. The tertiary structure is the three dimensional structure of a single protein chain. In order to predict the tertiary
structure [3], the secondary structure must be first predicted. However, secondary structures have recently been shown
to be useful in the prediction of regions of the protein likely to undergo structural change and in the classification of
proteins for genome analysis. |
Accurate prediction of protein secondary structure is a step toward the goal of understanding protein folding. A variety
of methods have been proposed that make use of the physicochemical characteristics of the amino acids (5), sequence
homology (6-8), pattern matching (9), and statistical analyses (10-15) of proteins of known structure. In a recent
assessment (16) of three widely used methods (5, 10, 13), accuracy was found to range from 49% to 56% for predictions of three states: helix, sheet, and coil. The limited accuracy of the predictions are believed to be due to the
small size of the data base and/or the fact that secondary structure is determined by tertiary interactions not included in
the local sequence[4]. |
In this paper we describe a secondary structure prediction method that makes use of neural networks. The neural
network technique has its origins in efforts to produce a computer model of the information processing that takes place
in the nervous system (17-20). A large number of simple, highly interconnected computational units (neurons) operate
in parallel. Each unit integrates its inputs, which may be both excitatory and inhibitory, and according to some
threshold generates an output, which is propagated to other units. In many applications, including the present work, the
biological relevance of neural networks to nervous system function is unimportant. Rather, a neural network may
simply be viewed as a highly parallel computational device. Neural networks have been shown to be useful in a variety
of tasks including modelling content-addressable memory (21), solving certain optimization problems (22), and
automating pattern recognition (23). The neural networks used here for secondary structure prediction are of the back
propagation. These networks are organized into layers as shown in fig. 1. Values of the input layer are propagated
through one or more hidden layers to an output layer. Specialization of a neural network to a particular problem
involves the choice of network topology that is, the number of layers, the size of each layer, and the pattern of
connections-and the assignment of connection strengths to each pair of connected units and of thresholds to each unit.
Interest in such networks has been stimulated by the recent development of a learning rule for the automatic assignment
of connection strengths and thresholds (24). In a "training" phase, initially random connection strengths (weights) and
thresholds (biases) are modified in repeated cycles by use of a data set, in this case known protein structures. In each
cycle adjustments are made to the weights and biases to reduce the total difference between desired and observed
output. At the end of the training phase, the "knowledge" in the network consists of the connection strengths and
thresholds that have been derived from the training data. This may be contrasted to pattern recognition by expert
systems (9), in which "knowledge" of the problem domain lies in the rules that are supplied by the "expert." |
Figure 1: an artificial neural network is an interconnected group of nodes, akin to the vast network of neurons in the
human brain.The tertiary structure of a protein describes the folding of its secondary structural elements and specifies
the positions of each atom in the protein, including those of its side chains. The known protein structures have come to
light through x-ray crystallographicor nuclear magnetic resonance (nmr) studies. The atomic coordinates of most of
these structures are deposited in a database known as the protein data bank (pdb). These data are readily available via
the internet (http://www.pbd.bnl.gov), which allows the tertiary structures of a variety of proteins to be analyzed and
compared [5]. |
SECONDARY STRUCTURE PREDICTION ARTIFICIAL NEURAL NETWORKS |
Neural Networks are referred to as Artificial Neural Networks (ANNs), Connectionism or Connectionist Models,
Multi-layer perceptron’s (MLPs) and Parallel Distributed Processing(PDP). However, despite all the different terms
and different types, there are a small group of “classic” networks which are widely used and on which many others are
based. These are: Back Propagation, Hopfield Networks, Competitive Networks and networks using Spiky Neurons[6]. |
MMP9_HUMAN P14780 DOI:10.2210/PDB1EAK/PDB P0823 |
MMP9 May play an essential role in local proteolysis of the extracellular matrix and in leukocyte migration. Could
play a role in bone osteoclasticresorption. Cleaves KiSS1 at a Gly-|-Leu bond. Cleaves type IV and type V collagen
into large C-terminal three quarter fragments and shorter N-terminal one quarter fragments.Degrades fibronectin but
not laminin or Pz-peptide. Exists as monomer or homodimer; disulfide-linked. Exists also as heterodimer with a 25 kDa
protein. Macrophages and transformed cell lines produce only the monomeric form. Interacts with ECM1.Activated by
4-aminophenylmercuric acetate and phorbol ester.Up-regulated by ARHGEF4, SPATA13 and APC via the JNK
signaling pathway in colorectal tumor cells.Produced by normal alveolar macrophages and granulocytes. Inhibited by
histatin-3 1/24 (histatin-5). Inhibited by ECM1.Belongs to the peptidase M10A family. Note: This description may
include information from UniProtKB. |
Protein type: Secreted, signal peptide; Protease; Secreted; EC 3.4.24.35; Motility/polarity/chemotaxis. |
Cellular Component: extracellular space; proteinaceous extracellular matrix; protein complex; extracellular region |
Molecular Function: collagen binding; protein binding; zinc ion binding; fibronectin binding; metalloendopeptidase
activity; protein complex binding |
Biological Process: response to nicotine; extracellular matrix organization and biogenesis; macrophage differentiation;
positive regulation of apoptosis; tissue remodeling; response to lipopolysaccharide; proteolysis; anatomical structure
regression; response to estradiol stimulus; extracellular matrix disassembly; response to radiation; cell growth; skeletal
development; response to drug; positive regulation of keratinocyte migration; ossification; response to retinoic acid;
positive regulation of synaptic plasticity; collagen catabolic process; positive regulation of angiogenesis; response to
ethanol; response to hyperoxia; response to mechanical stimulus; response to heat; response to hypoxia; response to
oxidative stress; embryo implantation; transformation of host cell by virus |
Reference #: P14780 (UniProtKB) |
Alt. Names/Synonyms: 67 kDa matrix metalloproteinase-9; 82 kDa matrix metalloproteinase-9; 92 kDagelatinase; 92
kDa type IV collagenase; CLG4B; Gelatinase B; GELB; macrophage gelatinase; MANDP2; matrix metallopeptidase 9
(gelatinase B, 92kDa gelatinase, 92kDa type IV collagenase); matrix metalloproteinase 9 (gelatinase B, 92kDa
gelatinase, 92kDa type IV collagenase); Matrix metalloproteinase-9; MMP-9; MMP9; type V collagenase |
Gene Symbols: MMP9 |
Molecular weight: 78,458 Da |
Basal Isoelectric point: 5.69 PredictpI for various phosphorylation states |
CST Pathways: Angiogenesis | GPCRsSignaling to MAPK/Erk |
Secondary structure results using MMP9 tool:
10 20 30 40 50 60 70 |
MSLWQPLVLVLLVLGCCFAAPRQRQSTLVLFPGDLRTNLTDRQLAEEYLYRYGYTRVAEMRGESKSLGPA |
hhhhhhhhheeeeetccccccccccceeeecccccccccchhhhhhhhhhhttcccccccccchhhhhhh |
LLLLQKQLSLPETGELDSATLKAMRTPRCGVPDLGRFQTFEGDLKWHHHNITYWIQNYSEDLPRAVIDDA |
hhhhhhhcccccccccchhhhhhhcccccccccccccccccccccccccceeeeeecccttcchhhhhhh |
FARAFALWSAVTPLTFTRVYSRDADIVIQFGVAEHGDGYPFDGKDGLLAHAFPPGPGIQGDAHFDDDELW |
hhhhhhhhhtcccceeeeccccccheeeeecccccccccccccccceeeeecccccccccccccctthhe |
SLGKGVVVPTRFGNADGAACHFPFIFEGRSYSACTTDGRSDGLPWCSTTANYDTDDRFGFCPSERLYTQD |
eettteeeeeeccccttcccccceeeetcccceeccccccccceeeeecccccccccccccccceeeeec |
GNADGKPCQFPFIFQGQSYSACTTDGRSDGYRWCATTANYDRDKLFGFCPTRADSTVMGGNSAGELCVFP |
ccccccccccceeeetcccceeccccccttcceeccccccccccccccccccccheeeccccttcceeee |
FTFLGKEYSTCTSEGRGDGRLWCATTSNFDSDKKWGFCPDQGYSLFLVAAHEFGHALGLDHSSVPEALMY |
eeeccccccccccttccccceeeeccccccttccetccctttceeeeehhhhhhhhetccccccctheee |
PMYRFTEGPPLHKDDVNGIRHLYGPRPEPEPRPPTTTTPQPTAPPTVCPTGPPTVHPSERPTAGPTGPPS |
hhhhccccccccccccctheeeeccccccccccccccccccccccccccccccccccccccccccccccc |
AGPTGPPTAGPSTATTVPLSPVDDACNVNIFDAIAEIGNQLYLFKDGKYWRFSEGRGSRPQGPFLIADKW |
cccccccccccccceeecccccccccchhhhhhhhhhtcceeeeettceeeeetccccccccceeeetcc |
PALPRKLDSVFEERLSKKLFFFSGRQVWVYTGASVLGPRRLDKLGLGADVAQVTGALRSGRGKMLLFSGR |
cccchhhhhhhhhhttcceeeettceeeeettceeccccccchttcccchhhhhhhhhtttceeeeeetc |
RLWRFDVKAQMVDPRSASEVDRMFPGVPLDTHDVFQYREKAYFCQDRFYWRVSSRSELNQVDQVGYVTYD |
eeeeeeeeeeeccttchhhhhhhcttccccccceeeetttteccccteeeeecccccccccctteeeeee |
ILQCPED |
Eeccccc |
Sequence length : 707 |
SOPMA : |
Alpha helix (Hh) : 112 is 15.84% |
310 helix (Gg) : 0 is 0.00% |
Pi helix (Ii) : 0 is 0.00% |
Beta bridge (Bb) : 0 is 0.00% |
Extended strand (Ee) : 153 is 21.64% |
Beta turn (Tt) : 58 is 8.20% |
Bend region (Ss) : 0 is 0.00% |
Random coil (Cc) : 384 is 54.31% |
Ambigous states (?) : 0 is 0.00% |
Other states : 0 is 0.00% |
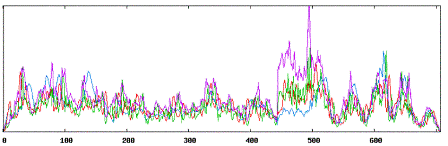 |
Using Neural Networks for MMP9: |
Alpha Helices: 221 |
Beta Sheets: 271 |
Coil or Turn: 215 |
TGFB1 P01137 DOI:10.2210/PDB3RJR/PDB P07200 |
TGFB1 Multifunctional protein that controls proliferation, differentiation and other functions in many cell types.
Many cells synthesize TGFB1 and have specific receptors for it. It positively and negatively regulates many other
growth factors. It plays an important role in bone remodeling as it is a potent stimulator of osteoblastic bone formation,
causing chemotaxis, proliferation and differentiation in committed osteoblasts. Homodimer; disulfide-linked, or
heterodimer with TGFB2. Secreted and stored as a biologically inactive form in the extracellular matrix in a 290 kDa
complex (large latent TGF-beta1 complex) containing the TGFB1 homodimer, the latency-associated peptide (LAP),
and the latent TGFB1 binding protein-1 (LTBP1). The complex without LTBP1 is known as the'small latent TGF-beta1
complex'. Dissociation of the TGFB1 from LAP is required for growth factor activation and biological activity. Release
of the large latent TGF-beta1 complex from the extracellular matrix is carried out by the matrix metalloproteinase
MMP3. May interact with THSD4; this interaction may lead to sequestration by FBN1 microfibril assembly and
attenuation of TGFB signaling. Interacts with the serine proteases, HTRA1 and HTRA3: the interaction with either
inhibits TGFB1-mediated signaling. The HTRA protease activity is required for this inhibition. Interacts with CD109,
DPT and ASPN.Activated in vitro at pH below 3.5 and over 12.5.Highly expressed in bone. Abundantly expressed in
articular cartilage and chondrocytes and is increased in osteoarthritis (OA). Co-localizes with ASPN in chondrocytes within OA lesions of articular cartilage.Belongs to the TGF-beta family. Note: This description may include
information from UniProtKB[7]. |
Protein type: Secreted, signal peptide; Secreted; Motility/polarity/chemotaxis. |
Cellular Component: proteinaceous extracellular matrix; extracellular space; cell surface; microvillus; cell soma;
Golgi lumen; axon; cytoplasm; extracellular region; nucleus |
Molecular Function: protein binding; eukaryotic cell surface binding; enzyme binding; protein homodimerization
activity; growth factor activity; protein heterodimerization activity; punt binding; protein N-terminus binding |
Biological Process: positive regulation of transcription, DNA-dependent; SMAD protein nuclear translocation; female
pregnancy; positive regulation of protein amino acid dephosphorylation; activation of NF-kappaB transcription factor;
regulation of protein import into nucleus; positive regulation of MAP kinase activity; connective tissue replacement
during inflammatory response; regulation of transforming growth factor beta receptor signaling pathway; negative
regulation of ossification; cell cycle arrest; inner ear development; positive regulation of isotype switching to IgA
isotypes; regulatory T cell differentiation; positive regulation of interleukin-17 production; response to drug; positive
regulation of chemotaxis; positive regulation of smooth muscle cell differentiation; active induction of host immune
response by virus; positive regulation of blood vessel endothelial cell migration; negative regulation of immune
response; regulation of sodium ion transport; negative regulation of fat cell differentiation; negative regulation of blood
vessel endothelial cell migration; lymph node development; positive regulation of protein secretion; positive regulation
of cell division; positive regulation of transcription from RNA polymerase II promoter; response to progesterone
stimulus; endoderm development; positive regulation of odontogenesis; myelination; negative regulation of
phagocytosis; evasion of host defenses by virus; positive regulation of cellular protein metabolic process; G1/S
transition checkpoint; myeloid dendritic cell differentiation; negative regulation of transcription from RNA polymerase
II promoter; phosphate metabolic process; negative regulation of cell proliferation; negative regulation of T cell
proliferation; regulation of DNA binding; ureteric bud development; negative regulation of release of sequestered
calcium ion into cytosol; positive regulation of cell proliferation; salivary gland morphogenesis; protein kinase B
signaling cascade; protein export from nucleus; inflammatory response; positive regulation of exit from mitosis; aging;
epidermal growth factor receptor signaling pathway; positive regulation of phosphoinositide 3-kinase activity; positive
regulation of bone mineralization; positive regulation of peptidyl-serine phosphorylation; SMAD protein complex
assembly; positive regulation of protein kinase B signaling cascade; embryonic development; positive regulation of
protein complex assembly; positive regulation of protein import into nucleus; induction of apoptosis; response to
hypoxia; epithelial to mesenchymal transition; negative regulation of cell growth; negative regulation of cell-cell
adhesion; negative regulation of transforming growth factor beta receptor signaling pathway; negative regulation of
skeletal muscle development; mononuclear cell proliferation; regulation of cell migration; protein amino acid
phosphorylation; hyaluronan catabolic process; response to vitamin D; negative regulation of neuroblast proliferation;
transforming growth factor beta receptor signaling pathway; receptor catabolic process; germ cell migration; response
to glucose stimulus; chondrocyte differentiation; defense response to fungus, incompatible interaction; negative
regulation of mitotic cell cycle; T cell homeostasis; cell growth; tolerance induction to self antigen; regulation of
striated muscle development; platelet activation; organ regeneration; negative regulation of DNA replication; virus-host
interaction; hemopoietic progenitor cell differentiation; negative regulation of transcription, DNA-dependent; positive
regulation of epithelial cell proliferation; cell death; positive regulation of collagen biosynthetic process; viral
infectious cycle; response to estradiol stimulus; negative regulation of cell cycle; positive regulation of histone
deacetylation; response to radiation; platelet degranulation; negative regulation of protein amino acid phosphorylation;
response to wounding; lipopolysaccharide-mediated signaling pathway; adaptive immune response based on somatic
recombination of immune receptors built from immunoglobulin superfamily domains; negative regulation of epithelial
cell proliferation; intercellular junction assembly and maintenance; regulation of binding; MAPKKK cascade; cellular
calcium ion homeostasis; gut development; protein import into nucleus, translocation; ATP biosynthetic process; positive regulation of histone acetylation; positive regulation of protein amino acid phosphorylation; negative
regulation of myoblast differentiation; blood coagulation; positive regulation of cell migration |
Reference #: P01137 (UniProtKB) |
Alt. Names/Synonyms: CED; DPD1; LAP; Latency-associated peptide; TGF-beta 1 protein; TGF-beta-1; TGFB;
TGFB1; TGFbeta; Transforming growth factor beta-1; transforming growth factor, beta 1 |
Gene Symbols: TGFB1 |
Molecular weight: 44,341 Da |
Basal Isoelectric point: 8.83 PredictpI for various phosphorylation states |
CST Pathways: Signaling Pathways Activating p38 MAPK |Wnt/ß-Catenin Signaling |
Secondary structure results using TGFB1 tool: |
10 20 30 40 50 60 70 |
MPPSGLRLLLLLLPLLWLLVLTPGRPAAGLSTCKTIDMELVKRKRIEAIRGQILSKLRLASPPSQGEVPP |
cctthhhhhhhhhhhheeeeecttcccccccccchhhhhhhhhhhhhhhhhhhhhhhecccccccccccc |
GPLPEAVLALYNSTRDRVAGESAEPEPEPEADYYAKEVTRVLMVETHNEIYDKFKQSTHSIYMFFNTSEL |
cccchhhhhhhhhhhhhhhhhhccccccccchhhhhhhhhheeecccccccccccccccceeeeeehhhh |
REAVPEPVLLSRAELRLLRLKLKVEQHVELYQKYSNNSWRYLSNRLLAPSDSPEWLSFDVTGVVRQWLSR |
hhhccchhhhhhhhhheeecccccccchhhhhhccccccheehheeeccccccceeeeehhhhhhhhhhc |
GGEIEGFRLSAHCSCDSRDNTLQVDINGFTTGRRGDLATIHGMNRPFLLLMATPLERAQHLQSSRHRRAL |
ttccceeeeeeccccccccccceeecchhhccccccceeecccccceeeeeecccccccccccccccccc |
DTNYCFSSTEKNCCVRQLYIDFRKDLGWKWIHEPKGYHANFCLGPCPYIWSLDTQYSKVLALYNQHNPGA |
cccccccccccccchhheeeehhhhtcceeeeccttccceeeccccceeeccccchhhhhhhhccccttc |
SAAPCCVPQALEPLPIVYYVGRKPKVEQLSNMIVRSCKCS |
cccccccccccccceeeeeetcccchhhhhhheehccccc |
Sequence length : 390 |
SOPMA : |
Alpha helix (Hh) : 125 is 32.05% |
310 helix (Gg) : 0 is 0.00% |
Pi helix (Ii) : 0 is 0.00% |
Beta bridge (Bb) : 0 is 0.00% |
Extended strand (Ee) : 68 is 17.44% |
Beta turn (Tt) : 12 is 3.08% |
Bend region (Ss) : 0 is 0.00% |
Random coil (Cc) : 185 is 47.44% |
Ambigous states (?) : 0 is 0.00% |
Other states : 0 is 0.00% |
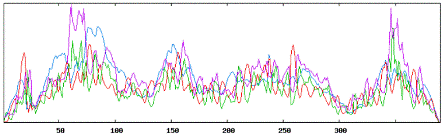 |
Using Neural Networks for TGF: |
Alpha Helices: 132 or 33.85% |
Beta Sheets: 158 or 40.51% |
Coil or Turn: 100 or 25.64% |
HLA-DRB1 P01912 DOI:10.2210/PDB1A6A/PDB P01903 |
HLA-DRB1 iso3 Binds peptides derived from antigens that access the endocytic route of antigen presenting cells
(APC) and presents them on the cell surface for recognition by the CD4 T-cells. The peptide binding cleft
accommodates peptides of 10-30 residues. The peptides presented by MHC class II molecules are generated mostly by
degradation of proteins that access the endocytic route; where they are processed by lysosomal proteases and other
hydrolases. Exogenous antigens that have been endocytosed by the APC are thus readily available for presentation via
MHC II molecules; and for this reason this antigen presentation pathway is usually referred to as exogenous. As
membrane proteins on their way to degradation in lysosomes as part of their normal turn-over are also contained in the
endosomal/lysosomal compartments; exogenous antigens must compete with those derived from endogenous
components. Autophagy is also a source of endogenous peptides; autophagosomes constitutively fuse with MHC class
II loading compartments. In addition to APCs; other cells of the gastrointestinal tract; such as epithelial cells; express
MHC class II molecules and CD74 and act as APCs; which is an unusual trait of the GI tract. To produce a MHC class
II molecule that presents an antigen; three MHC class II molecules (heterodimers of an alpha and a beta chain)
associate with a CD74 trimer in the ER to form a heterononamer. Soon after the entry of this complex into the
endosomal/lysosomal system where antigen processing occurs; CD74 undergoes a sequential degradation by various
proteases; including CTSS and CTSL; leaving a small fragment termed CLIP (class-II-associated invariant chain
peptide). The removal of CLIP is facilitated by HLA-DM via direct binding to the alpha-beta-CLIP complex so that
CLIP is released. HLA-DM stabilizes MHC class II molecules until primary high affinity antigenic peptides are bound.
The MHC II molecule bound to a peptide is then transported to the cell membrane surface. In B-cells; the interaction
between HLA-DM and MHC class II molecules is regulated by HLA-DO. Primary dendritic cells (DCs) also to express
HLA-DO.Lysosomalmiroenvironment has been implicated in the regulation of antigen loading into MHC II molecules;
increased acidification produces increased proteolysis and efficient peptide loading. Genetic variation in HLA-DRB1 is
a cause of susceptibility to sarcoidosis type 1 (SS1). Sarcoidosis is an idiopathic, systemic, inflammatory disease
characterized by the formation of immune granulomas in involved organs. Granulomas predominantly invade the lungs
and the lymphatic system, but also skin, liver, spleen, eyes and other organs may be involved. Belongs to the MHC
class II family. Note: This description may include information from UniProtKB[8]. |
Protein type: Membrane protein, integral |
Cellular Component: Golgi membrane; membrane; lysosomal membrane; late endosome membrane; integral to plasma
membrane; plasma membrane; trans-Golgi network membrane; external side of plasma membrane; MHC class II
protein complex |
Molecular Function: MHC class II receptor activity; peptide antigen binding. |
Biological Process: T-helper 1 type immune response; detection of bacterium; cytokine and chemokine mediated
signaling pathway; antigen processing and presentation of exogenous peptide antigen via MHC class II;
immunoglobulin production during immune response; T cell receptor signaling pathway; humoral immune response
mediated by circulating immunoglobulin; negative regulation of T cell proliferation; inflammatory response to
antigenic stimulus; regulation of interleukin-4 production; negative regulation of interferon-gamma production; T cell
costimulation; immune response; protein tetramerization. |
Reference #: P01912 (UniProtKB). |
Alt. Names/Synonyms: 2B13; cell surface glycoprotein; Clone P2-beta-3; Clone P2-beta-4; DR-1; DR-16; DR-5; DR-
8; DR1; DR16; DR5; DR8; DRB1; DRw10; DRw11; DRw8; FLJ75017; FLJ76359; HLA class II antigen beta chain;
HLA class II histocompatibility antigen, DR-1 beta chain; HLA class II histocompatibility antigen, DRB1-1 beta chain;
HLA class II histocompatibility antigen, DRB1-10 beta chain; HLA class II histocompatibility antigen, DRB1-11 beta
chain; HLA class II histocompatibility antigen, DRB1-16 beta chain; HLA class II histocompatibility antigen, DRB1-3
chain; HLA class II histocompatibility antigen, DRB1-8 beta chain; HLA-DR-beta 1; HLA-DR1B; HLA-DRB; HLADRB1;
HLA-DRB1*; human leucocyte antigen DRB1; leucocyte antigen DR beta 1 chain; leucocyte antigen DRB1;
lymphocyte antigen DRB1; major histocompatibility complex, class II, DR beta 1; MHC class I antigen DRB1*1;
MHC class I antigen DRB1*16; MHC class I antigen DRB1*8; MHC class II antigen DRB1*10; MHC class II antigen
DRB1*11; MHC class II antigen DRB1*3; MHC class II antigen HLA-DR13; MHC class II HLA-DR beta 1 chain;
MHC class II HLA-DR-beta cell surface glycoprotein; MHC class II HLA-DRw10-beta; SS1. |
Gene Symbols: HLA-DRB1 |
Molecular weight: 30,120 Da |
Basal Isoelectric point: 8.21 PredictpI for various phosphorylation states |
Secondary structure results using HLA DRB1 tool: |
10 20 30 40 50 60 70 |
MVCLRLPGGSCMAVLTVTLMVLSSPLALAGDTRPRFLEYSTSECHFFNGTERVRYLDRYFHNQEENVRFD |
eeeeecccchhhhhhhhhheeeccccccccccccheeehhccceeecttcchhheehhhhctthheeeec |
SDVGEFRAVTELGRPDAEYWNSQKDLLEQKRGRVDNYCRHNYGVVESFTVQRRVHPKVTVYPSKTQPLQH |
tttcheeeechtcccchhhhccchhhhhhhhhhhhhhccccccccchhhhccccctteeeeecccccccc |
HNLLVCSVSGFYPGSIEVRWFRNGQEEKTGVVSTGLIHNGDWTFQTLVMLETVPRSGEVYTCQVEHPSVT |
cheeeeeettcccccceeeeecttccccheeeeeeeccttcceeeeeeeeeccccttceeeeeeccttcc |
SPLTVEWRARSESAQSKMLSGVGGFVLGLLFLGAGLFIYFRNQKGHSGLQPRGFLS |
cceeeecccccchhhhhhhhhctheehhhhhhhhhheeeeccttccccccccceee |
Sequence length : 266 |
SOPMA : |
Alpha helix (Hh) : 68 is 25.56 |
310 helix (Gg) : 0 is 0.00% |
Pi helix (Ii) : 0 is 0.00% |
Beta bridge (Bb) : 0 is 0.00% |
Extended strand (Ee) : 75 is 28.20% |
Beta turn (Tt) : 23 is 8.65% |
Bend region (Ss) : 0 is 0.00% |
Random coil (Cc) : 100 is 37.59% |
Ambigous states (?) : 0 is 0.00% |
Other states : 0 is 0.00% |
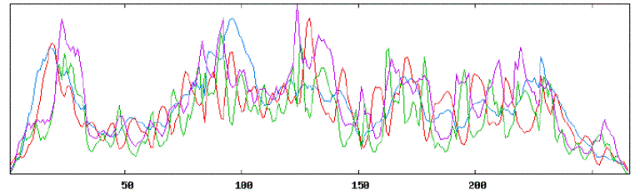 |
Using Neural Networks for HLA: |
Alpha Helices: 232 or 35.37% |
Beta Sheets: 241 or 36.74% |
Coil or Turn: 183 or 27.90% |
HLA-A GENE P18462 DOI:10.2210/PDB1Q94/PDB P13764, P61769 , P12499 |
HLA-A are a group of human leukocyte antigens (HLA) that are encoded by the HLA-A locus on human
chromosome 6p. The HLA genes constitute a large subset of the Major histocompatibility complex (MHC) of humans.
HLA-A is a component of certain MHC class I cell surface receptor isoforms that resides on the surface of all nucleated
cells and platelets. The receptor is a heterodimer, and is composed of a heavy, alpha (α) chain and smaller beta (β)
chain. The alpha chain is encoded by a variant HLA-A gene, and the beta chain (β2-microglobulin) is composed by the
invariant Beta-2 microglobulin gene[12]. |
MHC Class I molecules are part of a process that presents polypeptides from host of foreign derivation to the immune
system. Under normal conditions, if a peptide of foreign, pathogenic, source is detected, it alerts the immune system
that the cell may be infected with a virus, and, thus, target the cell for destruction[9]. |
The HLA-A gene is part of the Human MHC complex on chromosome 6. The region is at the telomeric end of the HLA
complex between theHLA-G and HLA-E genes. HLA-A gene encodes the larger, α-chain, constituent of HLA-A.
Variation of HLA-A α-chain in certain ways is key to HLA function. This variation promotes diversity of class I
recognition in the individual and also promotes genetic diversity in the population. This diversity allows more types of
foreign, virus or cancer, antigens to be 'presented' on the cell surface, but also allows a subset of the population to
survive if a new virus spreads rapidly through the population. |
These changes are also key to inter-individual histocompatibility of organs and tissues. Difference in exposed structures
of homologous proteins between individuals gives rise to antigen-antibody reactions when tissues are transplanted. This
form of antigenicity gives rise to serotypes in tissue recipients. Refined serotypes are what scientists have used for
grouping HLA. |
There are many variant alleles of the gene. The HLA-A gene was discovered after a long process of determining MHC
antigens. The original alleles discovered for MHC class I were not separated according to genes. The first 15 HL A1, 2,
3, 4, 5, 6, 7, 8, 9, 10, 11, 12, 13, 14, 15 contained antigens from many HLA loci. HL A1, 2, 3, 9, 10, 11 were later
found limited to a maximum of 2 in any given person. For example, a person could have A1, A2, A7, A8 but not A1,
A2, A3, and A11 or A7, A8, A14, A15. Given the exclusion HLA-A alleles were sorted according A and B, creating
HLA-A and HLA-B serotype groups, in late 1970s the first A and B isoforms were finally sequenced[10]. |
Secondary structure results using HLA A tool: |
10 20 30 40 50 60 70 |
MAVMAPRTLVLLLSGALALTQTWAGSHSMRYFYTSVSRPGRGEPRFIAVGYVDDTQFVRFDSDAASQRME |
heeccttheeeehhhhhhhhhhhhtccheeeeeeeeccttccccceeeeeeettteeeeecttccccccc |
PRAPWIEQEGPEYWDRNTRNVKAHSQTDRESLRIALRYYNQSEDGSHTIQRMYGCDVGPDGRFLRGYQQD |
ttcceecttccthhcttcheeeccccchhhhhhhhhhhhcccttccheehhhecccccttcceeeecccc |
AYDGKDYIALNEDLRSWTAADMAAQITQRKWETAHEAEQWRAYLEGRCVEWLRRYLENGKETLQRTDAPK |
ccttcceeeehhhhhhhhhhhhhhhhhhhhhhhhhhhhhhhhhhhhhhhhhhhhhhhttchhhecccccc |
THMTHHAVSDHEATLRCWALSFYPAEITLTWQRDGEDQTQDTELVETRPAGDGTFQKWASVVVPSGQEQR |
cceeccccccchhhhhhheetccccceeeeeccttccccctheeeecccttccceeeeeeeeecccccce |
YTCHVQHEGLPKPLTLRWEPSSQPTIPIVGIIAGLVLFGAVIAGAVVAAVMWRRKSSDRKGGSYSQAASS |
eeeeeccttcccceeeecccccccccceeeeetteeeehhhhtthhhhhhhetcccccccccccchhccc |
DSAQGSDMSLTACKV |
cccttcceeeehhhe |
Sequence length : 365 |
SOPMA : |
Alpha helix (Hh) : 108 is 29.59% |
310 helix (Gg) : 0 is 0.00% |
Pi helix (Ii) : 0 is 0.00% |
Beta bridge (Bb) : 0 is 0.00% |
Extended strand (Ee) : 90 is 24.66% |
Beta turn (Tt) : 40 is 10.96% |
Bend region (Ss) : 0 is 0.00% |
Random coil (Cc) : 127 is 34.79% |
Ambigous states (?) : 0 is 0.00% |
Other states : 0 is 0.00% |
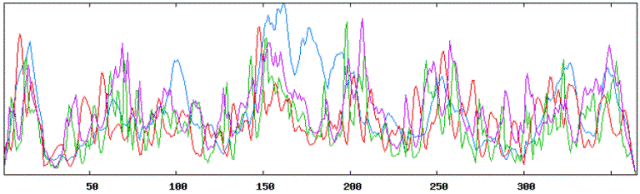 |
Using Neural Networks for HLA-A: |
Alpha Helices: 368 or 36.04% |
Beta Sheets: 373 or 36.53% |
Coil or Turn: 280 or 27.42% |
HLA-C GENE P30499 DOI:10.2210/PDB1EFX/PDB P04222, P61769, P52292 |
HLA-C belongs to the HLA class I heavy chain paralogues. This class I molecule is a heterodimer consisting of a
heavy chain and a light chain (beta-2 microglobulin). The heavy chain is anchored in the membrane. Class I molecules
play a central role in the immune system by presenting peptides derived from endoplasmic reticulum lumen. They are
expressed in nearly all cells. The heavy chain is approximately 45 kDa and its gene contains 8 exons. Exon one encodes
the leader peptide, exons 2 and 3 encode the alpha1 and alpha2 domain, which both bind the peptide, exon 4 encodes the alpha3 domain, exon 5 encodes the transmembrane region, and exons 6 and 7 encode the cytoplasmic tail.
Polymorphisms within exon 2 and exon 3 are responsible for the peptide binding specificity of each class one molecule.
Typing for these polymorphisms is routinely done for bone marrow and kidney transplantation. Over one hundred
HLA-C alleles have been described . |
Genetics Home Reference provides information about psoriatic arthritis, which is associated with changes in the HLAC
gene. |
UniProt (1C06_HUMAN) provides the following information about the HLA-C gene's known or predicted
involvement in human disease. |
Psoriasis 1 (PSORS1): A common, chronic inflammatory disease of the skin with multifactorial etiology. It is
characterized by red, scaly plaques usually found on the scalp, elbows and knees. These lesions are caused by abnormal
keratinocyte proliferation and infiltration of inflammatory cells into the dermis and epidermis. Note=Disease
susceptibility is associated with variations affecting the gene represented in this entry. |
Entrez Gene lists the following diseases or traits (phenotypes) known or believed to be associated with changes in
the HLA-C gene. |
• Congenital human immunodeficiency virus |
• Psoriasis susceptibility 1 |
UniProt and Entrez Gene cite these articles in OMIM, a catalog designed for genetics professionals and researchers that
provides detailed information about genetic conditions and genes[11]. |
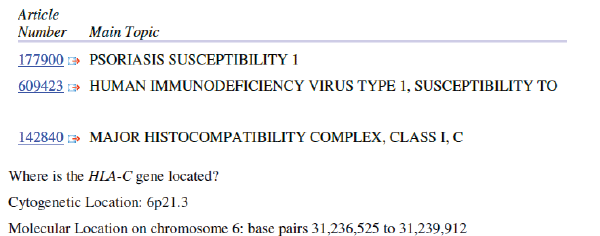 |
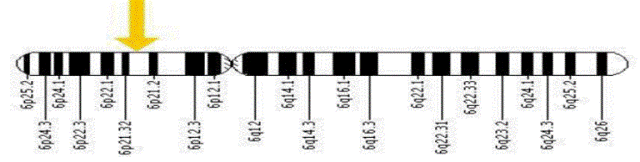 |
The HLA-C gene is located on the short (p) arm of chromosome 6 at position 21.3.
More precisely, the HLA-C gene is located from base pair 31,236,525 to base pair 31,239,912 on chromosome 6. |
Secondary structure results using HLA C tool: |
10 20 30 40 50 60 70 |
MRVMEPRTLILLLSGALALTETWACSHSMKYFFTSVSRPGRGEPRFISVGYVDDTQFVRFDSDAASPRGE |
heeccttheeeehhhhhhhhhhhhhhhhheeeeeeeccttccccceeeeeeettceeeeecttccccccc |
PRAPWVEQEGPEYWDRETQKYNRQAQTDRVSLRNLRGYYNQSEAGSHTLQWMCGCDLGPDGRLLRGYDQ
Y |
cccccccccccchhhhhhhhhhhhhhhhhhhhhhhhhhhcccccccceeeeeetccccttcceeetcche |
AYDGKDYIALNEDLRSWTAADTAAQITQRKWEAAREAEERRAYLEGTCVEWLRRYLENGKESLQRAEHPK |
eettcceeehhhhhhhhhhhhhhhhhhhhhhhhhhhhhhhhhhhhhhhhhhhhhhhhttchhhhhccctt |
THVTHHPVSDHEATLRCWALGFYPAEITLTWQWDGEDQTQDTELVETRPAGDGTFQKWAAVMVPSGEEQR |
ceeecccccchhhhhhhheetccccceeeeeecttccccchheeeecccccccceeeeeeeeccttccce |
YTCHVQHEGLPEPLTLRWEPSSQPTIPIVGIVAGLAVLAVLAVLGAVVAVVMCRRKSSGGKGGSCSQAAS |
eeeeeccttcccceeeecccccccccceeeehhhhhhhhhhhhhhhhheeeeeccccccccccccchhhc |
SNSAQGSDESLIASKA |
cccccccchhhhhhhh |
Sequence length : 366 |
SOPMA : |
Alpha helix (Hh) : 138 is 37.70% |
310 helix (Gg) : 0 is 0.00% |
Pi helix (Ii) : 0 is 0.00% |
Beta bridge (Bb) : 0 is 0.00% |
Extended strand (Ee) : 82 is 22.40% |
Beta turn (Tt) : 25 is 6.83% |
Bend region (Ss) : 0 is 0.00% |
Random coil (Cc) : 121 is 33.06% |
Ambigous states (?) : 0 is 0.00% |
Other states : 0 is 0.00% |
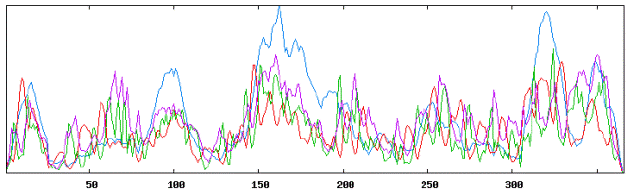 |
Using Neural Networks for HLA-C: |
Alpha Helices: 659 or 37.59% |
Beta Sheets: 636 or 36.28% |
Coil or Turn: 458 or 26.13% |
CONCLUSION |
The purpose of the system is to determine the protein structure prediction in different gene’s like mmp9, tgfb1, hladrb1,
hla-a, hla-c which play major role in central nervous system. In artificial neural networks, the mlp neural network
is used and which is trained by back propagation algorithm for generating the outputs for protein secondary structure
prediction (alpha helix, beta sheet and coils). An important conclusion that can be drawn from the experimental
evaluation is that the proposed “back propagation” algorithm results in optimal training to the neural network. More
efforts in structural analysis in concern with mutational studies can provide better insight towards development of drug resistance profiles of this gene. Thus the present study of modeling of proteins p14780, p01137, p01912, p18462,
p30499 and mmp9, tgfb1, hla-drb1, hla-a, hla-c genes has brought future prospective to an early diagnosis and
treatment against diabetic foot and helps for drug design. |
|
Figures at a glance |
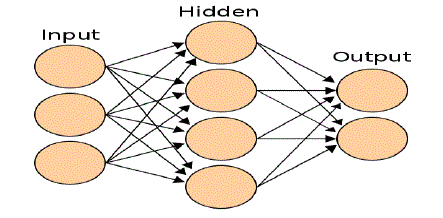 |
Figure 1 |
|
|
References |
- Lavery LA, Armstrong DG, Harkless LB. Classification of diabetic foot wounds. J Foot Ankle Surg. 1996.
- Armstrong DG, Lavery LA, Harkless LB. Treatment-based classification system for assessment and care of diabetic feet. J Am Podiatr Med Assoc. 1996; 86:311âÃâ¬Ãâ6.
- Levin, J. M., Robson, B. & Gamier, J. (1986) FEBS Lett. 205, 303-308.
- Nishikawa, K. &Ooi, T. (1986) Biochim. Biophys. Acta 871, 45-54.
- Wu, T. T. &Kabat, E. A. (1973) J. Mol. Biol. 75, 13-31.
- Caputo GM, Cavanagh PR, Ulbrecht JS, Gibbons GW, Karchmer AW. Assessment and management of foot disease in patients with diabetes. N Engl J Med. 1994.
- Gibrat, J., Gamier, J. & Robson, B. (1987) J. Mol. Biol. 198, 425-443.
- Val GD, Kriegsman DM, Assendelft WJ. Patient education for preventing diabetic foot ulceration. Cochrane Database Syst Rev 2001;
- Heeb, D. (1949) The Organization of Behavior (Wiley, NewYork).
- Minsky, M. (1954) Unpublished Doctoral Dissertation (Princeton Univ., Princeton, NJ).
- Rosenblatt, F. (1962) Principles of Neurodynamics (Spartan, New York).
- Rumelhart, D., Hinton, G. & Williams, R. (1986) Nature (London) 323, 533-536.
|