Research on non-invasive brain computer interfaces (BCI) has shown that electroencephalograhy (EEG) on-line signal extraction can be used for communica- tion (spelling), computer game playing and for sensor- assisted navigation. In this study we attempt to quan- tify reaching movement performance using EEG and gaze tracking signals. To achieve this the Berlin Brain Computer Interface has been linked to an eye and head tracker. The task studied was typing at a virtual keyboard, with a data information transfer rate of the resulting BCI of 70 bits/s, demonstrating that non- invasive BCI designs can provide useful means to command robotic devices for Brain Machine Interface (BMI) reaching tasks.
INTRODUCTION |
BCI interfaces present a unique opportunity for the restoration of motor and communicative function for patients
challenged by severe paralysis [1]. As the clinical causes of impairment can greatly vary, so can the residual level
of motor ability and the specific need of assistive technology. In the most affected pa- tients, the ‘locked-in’ group,
there is no residual mo- tor ability. As there are no other means available to the patient to communicate with outside
world, both invasive and non-invasive BCI use is warranted, within the limits posed by limited patient consent and
surgical risks. Nevertheless the relative number of these cases is rare: much more common are cases of spinal
trauma induced tetraplegia, in which arm func- tion is lost, but facial and eye muscle control remain intact. In such
cases, non-invasive means of restora- tion of reaching and grasping promises to offer sig- nificant benefits at limited
risk and cost and is ad- dressed in this study. The kinds of tasks that EEG BCI designs have been applied to
include spelling for communication for ALS and locked-in patients [1], computer games in normal subjects for
purposes of BCI development [2] and navigation of nearly autonomous intelligent robots [3] Meanwhile, invasive
BCI designs have shown effective restoration of grasp function in mon- keys [4, 5] and are currently being tested in
human patients.In the comparison of risks and benefits of various BMI designs, one of the significant performance
met- rics to consider is the expected speed-accuracy trade- off for reaching movements. Some invasive BMI
studies for monkeys report robot movements as fast as 2 seconds and trajectory accuracies on the order of 2 cm [4, 6].
While there are many other valid perform- ance measures, even after restricting criteria to those based on task
performance, given that some BMI de- signs go so far as orienting grippers and grabbing ob- jects, it is point-to-point
movement speed and accu- racy that remains the most basic of motor perform- ance measures which can be expected to
affect per- formance in more complex tasks. |
We have set up an experiment in which the accu- racy of a single reach is limited by the performance of gaze tracking
and the speed is limited by the perform- ance of a non-invasive BCI design. Using typing as a test task, we aimed to
measure the achievable speed- accuracy of a non-invasive brain to robot interface. |
METHODS |
A single, non-impaired volunteer subject was seated at a standard PC workstation. The subject wore a 64- channel EEG
cap connected to an amplifier system (BrainAmp128DC, Munich, Germany) sampling at 1KHz. The subject wore a
pair of eye tracking cam- eras (ViewPoint Eye Tracker, Arrington Research, Scottsdale, AZ) fixed with respect to
the cranium and to a 6 DOF head tracker (3Space Fastrack, Polhemus, Colchester, VT) by means of elastic band
strapped glasses. The combination of stereo eye tracker and head tracker was calibrated to locate the point of gaze on an LCD monitor. A picture of the experimental set- up is found in Figure 1. The EEG classification was based using the
common spatial patterns algorithm [7], in a three class paradigm, consisting of a ‘left’ handed movement
imaginations and a ‘relax’ class. Parameters were chosen such that there was considerable bias towards the rest class.
Deviations from the rest class were then used to trigger desired commands if gaze was steady at that particular time. |
The subject, after the standard 30min BBCI train- ing procedure, was instructed to type at a virtual keyboard shown on
a computer monitor. Its layout was based on the QWERTY arrangement, keeping only the letters, ‘space’ and ‘delete’
keys. The subject was asked to focus on the letter he wished to type, and while doing so, to imagine a left handed
movement. When this movement imagination was detected, the letter being fixated was added to the sentence being
typed, which is shown on the screen, slightly below the keyboard. A key press event blocked the BCI for the next 1s.
The dimensions of the keys were under1.5x1.5cm except space and delete which were 4cm wide. The distance from
eye to screen was roughly 60cm. The cursor was visible and the screen also showed a horizontally moving ball
providing feed- back of the BCI classifier state to the subject. |
The results are shown below for a typical sentence. |
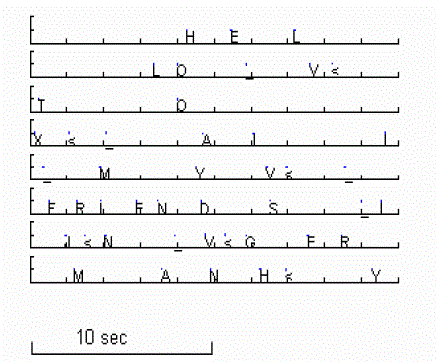 |
Figure 2: A typical sequence of key presses vs. time. On average, 68.4% of keys ‘pressed’ were intended in the sense
of ‘next character in the intended sentence’. However, if the ‘delete’ key can be counted as ‘intended’, 84.2% of
key presses were correctly detected. The process resulted in a typing rate of 14.2 correct chars/min (equiv. to 70.5
bits/min) for the 3 repeated sentences tested. |
DISCUSSION |
As a demonstration of the efficacy and simplicity of combining eye tracking and EEG for BMI design, we believe
that this pilot study was successful. Yet one may ask why EEG-BCI is necessary at all, and the move command
or set of commands is not instead given by eye blinks, facial EMG or a voice command, if these abilities are
present in the target patient set. The answer is quite simple: producing a movement by imagining it is quite different
than talking one’s arm, real or prosthetic, into doing so. The intuitive link and qualitative experience, we hope, would
be a motivating factor for the continued and successful use of such a BMI by the patients whose lives can be positively
affected by it. Certainly, useful everyday arm movements in- volve more than just point-to-point reaching: concur- rent
grasping and hand orientation are also important and remain to be tested for BMI designs. Much of the benefit
assessment of assistive technology will depend upon upcoming ‘realistic setting’ studies of long enough duration to
provide reliable feedback from disabled users and their physicians. |
Although the current study limited itself to 2D tar- get identification, it is easy to imagine how the gaze/BCI procedure
can be extended to pick out 3D targets on physical objects for a physical robot to reach to. The question remains as
to what 3D target accuracy stereo gaze tracking can provide vs. the 2D accuracy reported herein, which is common but
is aided by a priori knowledge of distance of gaze point from the eyes. Future improvements require better on- line
classifications of ‘rest’ vs. several ‘active’ states to improve responsiveness and perhaps control multi- ple motor
parameters at once via BCI. |
Figures at a glance |
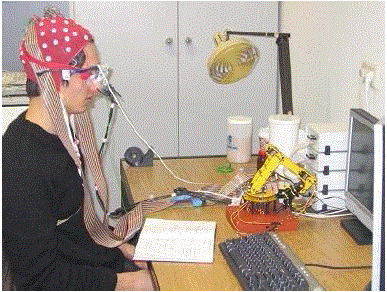 |
Figure 1 |
|
|
References |
- J. R. Wolpaw, McFarland, D.J., Vaughan, T.M. and Schalk, G., "The Wadsworth Center Brain-Computer Interface (BCI) Research andDevelopment Program.," IEEE Transactions on Neural Systems & Rehabilitation Engineering, vol. 11, pp. 204-207, 2003.
- S. Lemm, B. Blankertz, G. Curio, and K.-R. Müller., "Spatio-spectral filters for improved classification of single trial EEG.," IEEETranactions on. Biomedical Enineering., vol. 52, pp. 1541-1548, 2005.
- J. d. R. Millán, F. Renkens, J. Mouriño, and W. Gerstner:, "Brain-actuated interaction.," Artificial Intelli- gencevol. 151, pp. 241-259, 2004.
- J. M. Carmena, M. A. Lebedev, R. E. Crist, J. E. O'Doherty, D. M. Santucci, D. F. Dimitrov, P. G. Patil, C. S. Henriquez, and M. A.Nicolelis, "Learning to control a brain-machine interface for reaching and grasping by pri- mates," PLoSBioogyl, vol. 1, pp. E42, 2003.
- A. B. Schwartz, "Cortical neural prosthetics," Annu Rev Neurosci, vol. 27, pp. 487-507, 2004.
- W. Wu, Y. Gao, E. Bienenstock, J. P. Donoghue, and M. J. Black, "Bayesian population decoding of motor cortical activity using a kalmanfilter," Neural Computing, vol. 18, pp. 80-118, 2006.
- G. Dornhege, B. Blankertz, G. Curio, and K.R. Mueller, "Boosting bit rates in non-invasive EEG single trial classifications by featurecombination and multi-class para- digms," IEEE Transactions on Biomedical Engineering, vol. 51, pp. 993-1002, 2004.
|